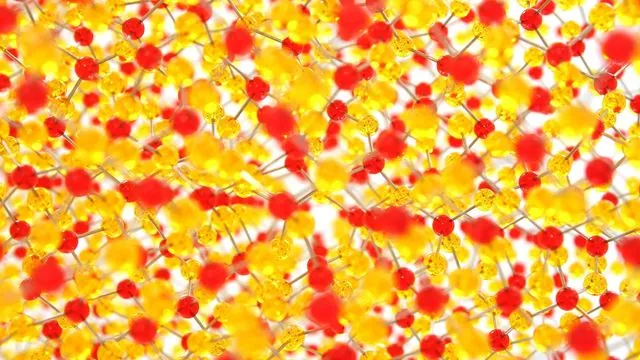
Revolutionary AI Model Unlocks Secrets of Crystalline Structures!
2024-09-20
Author: Rajesh
Introduction
For over a century, scientists have relied on X-ray crystallography to decode the intricate structures of crystalline materials, encompassing a wide array of substances including metals, rocks, and ceramics. Traditionally, this method shines when dealing with intact crystals. However, when presented with powdered samples—random fragments of the original crystal—scientists encounter significant challenges in reconstructing the structure effectively.
The Breakthrough with Crystalyze
In an exciting breakthrough, researchers from MIT have developed a cutting-edge generative AI model named Crystalyze, poised to transform how we determine the structures of powdered crystals. This innovative tool could drastically enhance our understanding of materials crucial for various applications ranging from batteries to magnets.
According to Danna Freedman, the Frederick George Keyes Professor of Chemistry at MIT, "Understanding structure is fundamental for any material. It's critical for superconductivity and magnetism, and it influences every materials-centric application you can imagine."
Freedman, alongside Jure Leskovec from Stanford, spearheaded the study published in the Journal of the American Chemical Society. Graduate student Eric Riesel from MIT and undergraduate Tsach Mackey from Yale served as lead authors of this pivotal research.
The Science Behind Crystals
Crystalline materials, including metals and numerous inorganic solids, consist of ordered lattices formed from identical repeating units—essentially “boxes” holding precisely arranged atoms. X-rays directed at these lattices reveal vital information about atomic positions and interatomic bonds through a process known as diffraction.
However, when dealing with powdered crystal forms, the task of determining structures becomes notably more complex. Freedman explains, "A powder is essentially a collection of microcrystals. Each microcrystal retains the same lattice of a larger crystal but occupies a disordered orientation."
With countless powdered materials collected over decades, many still await structural analysis. To tackle this challenge, Freedman and her team trained their machine-learning model using data from the Materials Project, a comprehensive database of over 150,000 materials. They employed simulation techniques to replicate X-ray diffraction patterns and utilized these patterns to educate their AI model, Crystalyze, which predicts structures based on these inputs.
What Makes Crystalyze Unique?
The Crystalyze model breaks down the prediction process into manageable subtasks. Initially, it identifies the size and shape of the crystalline lattice and the atoms that will fill the structure. Then, it predicts atomic arrangements within the lattice “box.” For each input diffraction pattern, Crystalyze crafts several potential structures, making it possible to verify these predictions against actual diffraction data.
"Our generative AI model can create several novel guesses, sometimes up to a hundred," Riesel notes. "By comparing the predicted powder pattern against the actual input pattern, we can confidently confirm our findings."
Proven Results
In tests using thousands of simulated diffraction patterns and over 100 experimental patterns from the RRUFF database—housing data for nearly 14,000 natural crystalline minerals—Crystalyze achieved a commendable accuracy rate of 67%. The research team further applied the model to patterns that had remained unsolved until now, identifying structures for over 100 previously enigmatic patterns.
Moreover, the team successfully utilized their model to decipher three structures born from high-pressure reactions involving elements that generally resist forming compounds at atmospheric pressure—a technique that could lead to the development of truly novel materials with unique properties.
Notable examples include both graphite and diamond, which are composed entirely of carbon yet possess dramatically different structural and physical characteristics. The new materials identified in Freedman’s lab, featuring bismuth as an element, hold potential for creating advanced permanent magnets.
Freedman proudly states, "We uncovered numerous new materials from pre-existing data, and most importantly, we resolved three unknown structures from compounds created in our lab that represent the first new binary phases of these combinations."
Conclusion
The findings from this innovative research could prove invaluable in multiple fields related to material science. Interested researchers can access the user-friendly interface for the Crystalyze model at crystalyze.org, opening new doors in the quest to unlock the complexities of powdered crystalline materials.
Stay tuned for more groundbreaking developments in material science that could reshape technology and industrial applications!