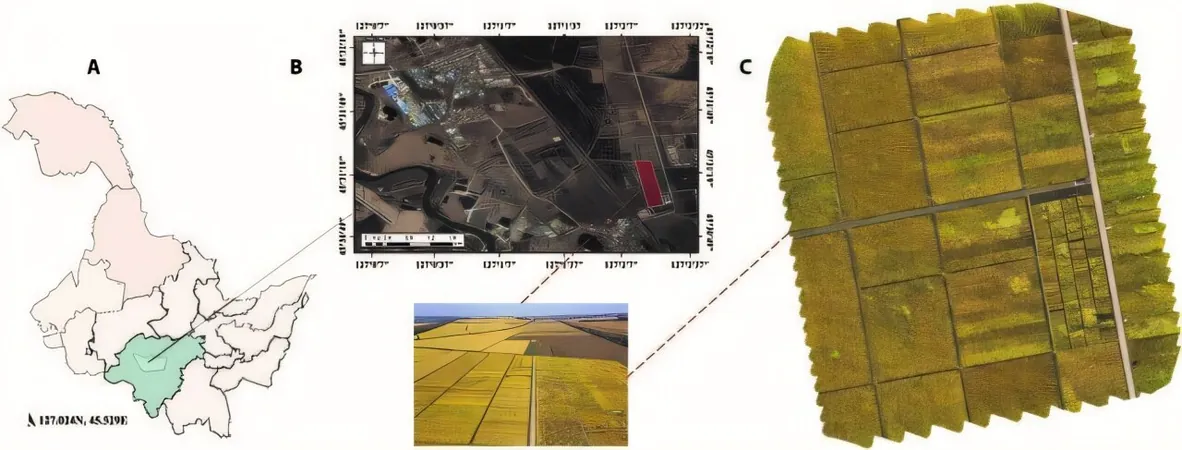
Revolutionary AI Model Transforms Rice Lodging Detection, Promising Unprecedented Agricultural Efficiency
2024-11-11
Author: Daniel
Introduction
In a groundbreaking development for the agricultural sector, researchers have unveiled an AI-powered model that is reshaping rice lodging detection, leading to enhanced productivity and sustainability in farming. Utilizing state-of-the-art convolutional neural network (CNN) architecture alongside cutting-edge optimization algorithms, this innovative model not only improves accuracy but also cuts down on computational expenses compared to traditional methods.
Understanding Rice Lodging
Rice lodging, a major agricultural concern, occurs when crops bend or fall due to adverse environmental conditions like strong winds or heavy rain. This phenomenon can severely impact crop yield by disrupting photosynthesis, complicating harvesting, and increasing susceptibility to pests. Consequently, effective monitoring and prediction of lodging are paramount for farmers and agronomists striving to safeguard their crops and optimize yields.
Limitations of Traditional Methods
Historically, rice lodging assessments have relied on labor-intensive and often inaccurate approaches, such as manual visual inspections, complex mathematical models, and satellite remote sensing. These conventional techniques fall short in scalability and immediate responsiveness, which are crucial for large-scale agricultural practices.
The AAUConvNeXt Model
A new study published in the journal Plant Phenomics details a transformative model known as AAUConvNeXt, developed through multi-objective optimization using the innovative AFOA-APM algorithm. This model enhances the previous UConvNeXt CNN architecture, enabling precise segmentation of rice lodging instances.
Key Innovations and Results
The research emphasizes the importance of adjusting convolutional layer channels dynamically—boosting them in critical learning layers and toning them down in less critical ones. This strategic design balances model complexity with resource consumption.
Experimental results have been striking, showcasing AAUConvNeXt's superiority over established models such as DeepLabV3+ and HRNet. It achieved impressive metrics: a Pixel Accuracy (PA) of 96.3%, Mean Pixel Accuracy (MPA) of 96.3%, and a mean Intersection over Union (mIoU) of 93.2%. Besides, the model reduces the overall parameter count and computational complexity by 8.66%, making it a resource-efficient solution for farmers.
Segmentation Accuracy
The high segmentation accuracy is particularly noteworthy, especially in distinguishing between different lodging scenarios—full, partial, and non-lodged states—which have historically proven challenging for existing detection systems.
Research Insights
Ablation studies revealed that the synergy between AFOA and APOM significantly boosted segmentation metrics, marking a substantial leap in performance compared to its predecessors. The targeted adjustment of channels allows the AAUConvNeXt model to proficiently learn both crucial early-stage and refined features necessary for precise lodging detection.
Expert Commentary
Dr. Xiaobo Sun, the senior researcher involved in the study, stated, "By harnessing the power of deep learning coupled with intelligent optimization, this model represents a significant leap forward in crop lodging monitoring. Our innovation could transform rice farming practices by providing timely, reliable, and cost-effective solutions to some of agriculture's most pressing challenges."
Future Implications
The implications of the AAUConvNeXt model extend beyond mere crop monitoring; its integration into farming practices could herald a new era of efficient crop management. As global demand for food surges, advancements like these promise to enhance agricultural productivity and sustainability, paving the way for a future where technology meets food security needs.
Conclusion
Farmers and researchers alike are encouraged to explore how this breakthrough model can be incorporated into their practices, potentially revolutionizing crop management while addressing the challenges posed by climate change and environmental variability.