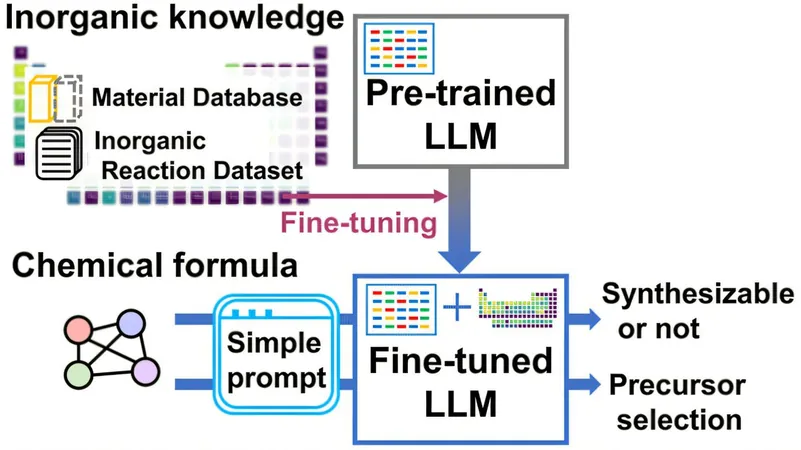
Revolutionary AI Model Set to Transform Material Design and Enhance Industry Competition
2025-03-27
Author: Daniel
Introduction
In a groundbreaking advancement for material science, a research team from Seoul National University has unveiled a cutting-edge technology utilizing Large Language Models (LLMs) to not only predict the synthesizability of novel materials but also provide insightful explanations for these predictions. This pioneering work, led by Professor Yousung Jung, involved collaboration with researchers from Fordham University in the United States.
The Importance of Synthesizability
Why does this breakthrough matter? Synthesizability, or the potential to successfully create a material in a lab, is a critical aspect of material design. Poor predictions can lead to wasted time and resources on experiments with unfeasible materials. Traditional techniques have struggled with low accuracy and have often failed to explain why certain materials might be synthetic failures, but this innovative approach promises a solution.
Innovative LLM Approach
In a significant departure from conventional methods, the research introduces an LLM that not only achieves precise synthesizability predictions but also deciphers the underlying reasons behind these assessments. The model was finely tuned using diverse inorganic crystal datasets formatted as text, allowing it to classify materials based on their synthesizability, predict precursor compounds necessary for their synthesis, and interpret key influential factors.
Understanding Predictions
A remarkable facet of this research is the model's ability to provide context and explanations for its predictions, revealing why certain crystal structures are easier to synthesize than others. This feature enables scientists to uncover complex correlations that have thus far gone unnoticed, paving the way for a more informed approach to material development.
Industrial Implications
The implications of this technology are monumental, particularly for advanced industries in Korea, such as semiconductors and secondary batteries. By streamlining material design processes and minimizing trial-and-error methodologies, LLM-based predictive technology could significantly cut development times, enabling faster innovation and maintaining Korea's competitive edge in technology.
Commercialization Potential
Moreover, if commercialized, this research could revolutionize how companies and research institutes rapidly identify new materials and assess their feasibility for large-scale production.
Expert Insights
Professor Yousung Jung emphasized the significance of the study, stating, "Not only can LLMs predict the synthesizability of new materials with high accuracy, but they can also elucidate the reasoning behind these predictions, revealing essential chemical principles."
Postdoctoral researcher Seongmin Kim, a key contributor from the Institute of Chemical Processes at Seoul National University, is poised to continue this vital work, integrating machine learning with materials science to explore transformative shifts in material development.
Conclusion
This advancement is not just a leap forward in scientific research; it's a potential game-changer for industries striving for innovation and efficiency in a rapidly evolving technological landscape. Stay tuned for more updates as this technology paves the way for the materials of tomorrow!