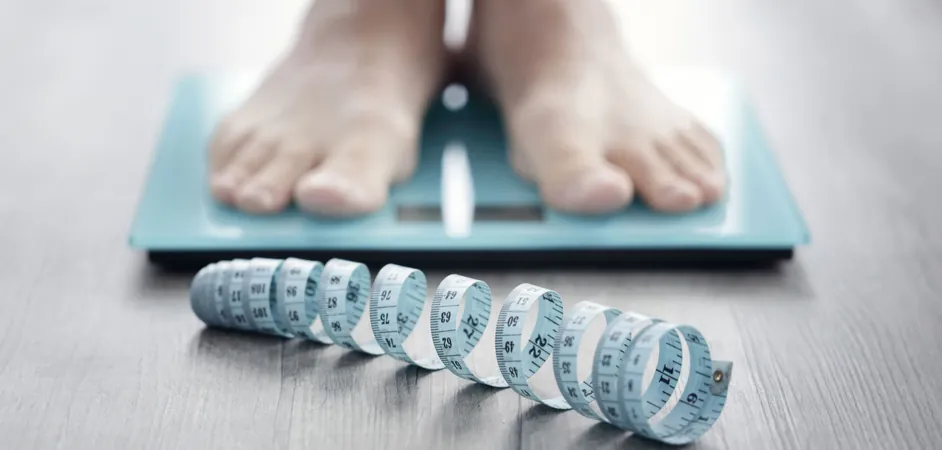
Revolutionary AI Model Revolutionizes Cancer Cachexia Detection
2025-04-27
Author: Li
Unveiling a Game-Changer in Cancer Care
At the recent American Association for Cancer Research (AACR) Annual Meeting, researchers unveiled a groundbreaking artificial intelligence (AI) model that drastically improves the detection of cancer cachexia, a debilitating condition many cancer patients face. This innovative model analyzes standard clinical data and imaging information with impressive accuracy, raising hopes for better patient outcomes.
What is Cancer Cachexia?
Cancer cachexia is a severe syndrome marked by systemic inflammation, significant muscle loss, and drastic weight reduction. Sabeen Ahmed, a graduate student from the University of South Florida and Moffitt Cancer Center, emphasized the critical nature of early detection. "Identifying cancer cachexia allows for timely lifestyle and pharmacological interventions that can slow muscle wastage and enhance patients' quality of life." Unfortunately, traditional methods are often subjective and late in identifying the condition, leading to missed opportunities for intervention.
Harnessing AI for Enhanced Detection
Ahmed and her team proposed that an AI-driven biomarker could revolutionize the detection process. Utilizing a sophisticated multilayer perceptron model, their approach combines imaging scans with various clinical data to generate an AI-derived risk indicator for cancer cachexia. This advanced method can unveil intricate patterns often overlooked by conventional analyses.
Impressive Accuracy in Detection
In tests involving patients with pancreatic cancer, the AI model successfully identified cachexia in 77% of cases. Adding lab results boosted accuracy to 81%, while incorporating structured clinical notes skyrocketed it to 85%. Furthermore, when predicting patient survival rates, the AI model proved its prowess, surpassing traditional methods by enhancing accuracy across pancreatic, colorectal, and ovarian cancers.
How Does the Model Work?
The AI-driven model operates in two critical steps. First, it analyzes diagnostic images like CT scans to measure skeletal muscle mass through an automated algorithm. This ensures that confidence levels are reported, highlighting any potential discrepancies from manual assessments.
Secondly, it aggregates various clinical data—lab results, electronic medical records, and physical metrics like height and weight—together with skeletal muscle evaluations to compute the AI-driven biomarker.
Validation and Reliability
The AI model's measurements were rigorously validated against expert radiologists' assessments, showing a median discrepancy of just 2.48%. This small margin underscores the reliability of this AI approach, reinforcing its potential as a clinical tool.
The Future of Cancer Care
Ahmed’s research reveals a scalable, objective method for detecting cancer cachexia, potentially allowing healthcare providers to implement interventions earlier. With machine learning's growing role in personalized treatment strategies, this development is poised to transform cancer care.
Looking Ahead: Limitations and Opportunities
While the study's findings are promising, the AI model was tested primarily within specific cancer types, limiting its current applicability. Additionally, the reliance on CT scans for muscle analysis suggests a need for incorporating more imaging modalities for expanded robustness. As always, the effectiveness of any AI tool will depend on the quality of the data it analyzes.
Supported by various prestigious institutions, Ahmed’s team shows that innovation in cancer care through AI could lead to life-saving advancements. With ongoing research, the future of personalized cancer treatments looks brighter than ever.