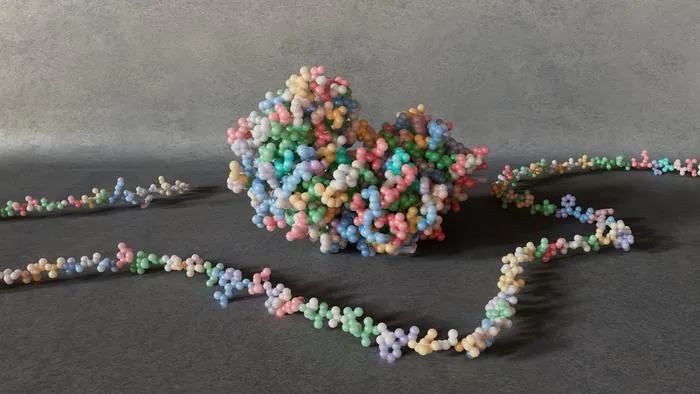
Revolutionary AI is Transforming Enzyme Engineering: Here’s How!
2025-01-24
Author: Wei Ling
Overview of the Breakthrough
In a groundbreaking development that may reshape energy, materials, biotechnology, and medical fields, researchers have unveiled a machine-learning guided platform that accelerates enzyme engineering. This innovative platform not only designs thousands of novel enzymes but also predicts their real-world behavior and evaluates their performance across various chemical reactions.
The study, which features in the esteemed Nature Communications, was spearheaded by a collaborative team from Stanford University and Northwestern University. Their paper, titled "Accelerated enzyme engineering by machine-learning guided cell-free expression," presents an ingenious approach to overcoming the limitations currently faced in enzyme engineering.
Tackling the Challenges
The primary obstacle in enzyme engineering has been the cumbersome task of generating and analyzing vast datasets that map sequence-function relationships for predictive design. The researchers tackled this challenge by creating a unique machine learning (ML)-guided platform that integrates cell-free DNA assembly with functional assays, effectively streamlining the mapping of protein sequence spaces.
Professor Michael Jewett from Stanford University, a leading author of the study, emphasized the revolutionary nature of the approach. "Our computational process enables us to engineer enzymes significantly faster without the need for living cells, which typically adds time to the process," he explained. "With machine learning, we can simulate and predict the most efficient enzyme variants from mutated DNA sequences, allowing us to finalize our experiments in days instead of the usual months."
The Evolution of Enzyme Engineering
Traditionally, scientists have had to modify existing enzymes found in nature to tailor them for specific functions, a process that requires sourcing DNA for these variants from external vendors and transferring it into cells for enzyme production. However, this new method allows the entire process to be conducted on a computer, enabling the achievement of enzyme activity enhancements without the trial and error of thousands of live chemical reactions.
While enzyme engineering isn't a novel concept, the application of machine learning to expedite this process is revolutionary. Known as "directed evolution," the discipline traditionally relied on random mutations to discover enzyme variants. Jewett's team is now leveraging computational power to attain this evolution at an unprecedented pace.
Notable Achievements and Future Directions
One notable success of the new platform was the synthesis of a small-molecule pharmaceutical achieved at a remarkable 90% yield, a staggering improvement from an initial 10% yield. This success illustrates the model's potential to establish multiple specialized enzymes simultaneously, which could then be used to develop additional therapeutics. Jewett is currently seeking a pharmaceutical partner to further advance this promising model.
Looking ahead, Jewett’s group aims to expand the application of their machine-learning models beyond amide bond formation—which is critical in pharmaceuticals—to encompass a broader range of chemical reactions. They envision exploring opportunities in sustainability and the burgeoning bioeconomy, such as creating enzymes that can degrade environmental toxins or improve the bioavailability of protein-rich foods.
Challenges Ahead
However, challenges remain. Jewett acknowledged that high-quality, high-volume functional data is scarce, posing obstacles for AI integration. "AI thrives on data, and we're still in the early phases of gathering robust datasets," he noted. Currently, the study evaluated about 3,000 enzyme mutants across 1,000 products and around 10,000 chemical reactions.
As researchers continue to harness the power of machine learning to enhance enzyme engineering, the demand for comprehensive data sets is set to grow. Jewett remains optimistic about the future trajectory of the field, stating, "This is just the beginning. With continued innovation and data acquisition, we can expect remarkable advancements in enzyme engineering."
Conclusion
Stay tuned, as the unfolding journey of AI-enhanced enzyme engineering promises to unleash a wave of scientific advancements that could change the face of various industries!