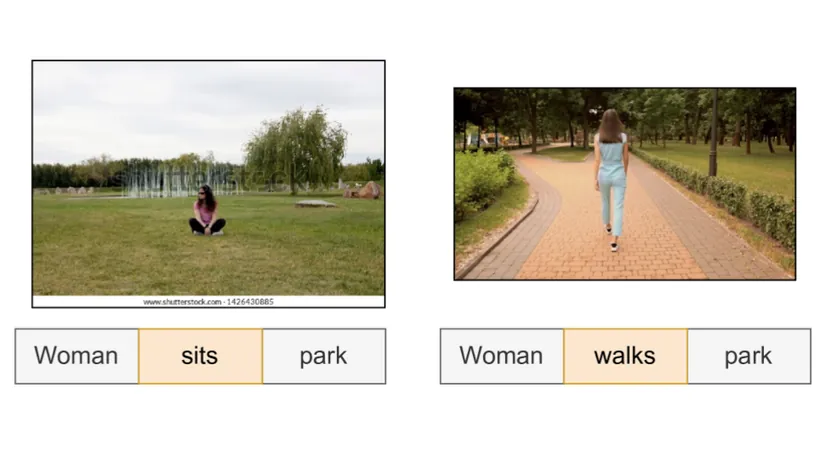
Quantum Computing Steps into the Future: New Framework Rivals Classical AI in Text-Image Tasks
2025-01-05
Author: Rajesh
Groundbreaking Research from University College London
In an exciting breakthrough for artificial intelligence, researchers at University College London (UCL) have unveiled a cutting-edge quantum framework called MultiQ-NLP. This innovative model seamlessly integrates language and image data within a structured, quantum-driven approach, showcasing impressive results that may redefine our understanding of AI interpretation and transparency.
By encoding both text and images into quantum circuits, the MultiQ-NLP framework takes advantage of quantum computing’s innate ability to manage intricate tensor structures. This not only enhances the efficiency of processing complex data but also improves the interpretability of AI systems, potentially addressing one of the key criticisms of traditional AI models: their "black box" nature.
Revolutionizing Multimodal Understanding
The team tested their new quantum model on a widely recognized image classification task, known as SVO-Probes, which challenges AI to accurately match image captions. Remarkably, the quantum model achieved results that are comparable to existing classical AI methods. This accomplishment suggests that quantum-enhanced techniques could play a crucial role in future applications of language and image understanding.
Addressing AI's Transparency Dilemma
One of the significant hurdles faced by modern large language models (LLMs) is their lack of transparency. With billions of parameters tangled in complex webs, it is often nearly impossible to discern why specific words are chosen or how AI interprets visual data in conjunction with textual information. The UCL researchers aim to remedy this through their novel treatment of language and images as mathematical constructs, ideally suited for quantum processing.
By focusing on the structural relationships intrinsic to language—such as syntax and grammar—MultiQ-NLP can model these factors more naturally than conventional approaches that often rely solely on brute-force pattern recognition.
A Sophisticated Tensor Model for Text and Images
At the core of MultiQ-NLP lies the representation of language and images as higher-order tensors, which are multidimensional arrays capturing the complex relationships between words. Traditional hidden layer models can struggle with tensor-based training on classical hardware due to high computational costs. However, on quantum processors, these tensors can be represented as qubits, allowing for more refined and effective processing of intricate relationships.
The framework takes an existing quantum natural language processing method and expands it to encompass images. By translating visual data from classical neural networks into quantum states, MultiQ-NLP achieves a cohesive mathematical representation that treats language and imagery simultaneously.
Exciting Results from Rigorous Testing
The researchers put MultiQ-NLP to the test using the SVO-Probes dataset, which challenges models to correlate image-text pairs by focusing on grammatical roles. Their quantum model competed excellently against leading classical models, highlighting the framework’s potential to enhance AI interpretability. The results underscore the importance of capturing syntax and grammatical structure, especially in more complex language scenarios.
While simpler models without structural consideration performed adequately on basic tasks, the profound advantage of syntax-driven quantum models in more complicated language challenges is undeniable.
Future Challenges and Opportunities
Despite these promising outcomes, the research team acknowledges several limitations that warrant further exploration. Currently, these experiments were conducted on simulators, as real quantum hardware is still developing. This limitation meant that smaller datasets were utilized, which could inhibit the model’s full potential.
Scaling this framework is vital for future advancements. As the field of quantum computing evolves, integrating advanced quantum devices and optimization techniques could dramatically enhance MultiQ-NLP’s performance on substantial datasets.
The Potential Impact on AI and Quantum Technologies
The implications of scaling MultiQ-NLP are profound. With the growing significance of large language models in sectors such as healthcare, finance, and law, the potential for a more intelligible AI could change the way users and regulators perceive technology's reasoning. An approach that combines structured representations with quantum computing could innovate how we understand and manipulate data, leading to applications that extend far beyond current technological boundaries.
Towards a More Transparent AI Future
The MultiQ-NLP framework not only matches classical AI capabilities in complex text-image tasks but also enhances interpretability, a characteristic that is crucial as society increasingly relies on AI systems. As researchers continue to refine quantum data processing methods, frameworks like MultiQ-NLP could play a pivotal role in shaping an AI landscape that is both powerful and comprehensible.
Stay tuned for more advancements in this fascinating area of research, as it holds the promise of revolutionizing our interaction with artificial intelligence, making it smarter and more transparent than ever before!