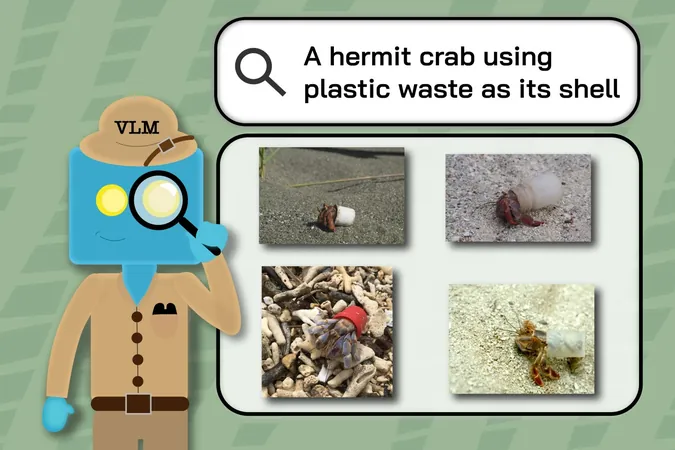
New Study Unveils Hidden Weaknesses in AI Models for Wildlife Image Retrieval
2024-12-20
Author: Daniel
Introduction
Ecologists face a monumental task when trying to capture the diversity of North America's approximately 11,000 tree species, with only a fraction represented in the vast databases of nature images. These extensive collections contain millions of snapshots, covering everything from butterflies to humpback whales, and serve as an invaluable research tool. They provide critical insights into unique behaviors, migration patterns, and the impacts of pollution and climate change on wildlife.
Challenges in Current Image Datasets
However, existing nature image datasets are still lacking in effectiveness. Searching for relevant images manually can be a daunting and time-consuming process for researchers. Enter multimodal vision language models (VLMs)—advanced artificial intelligence systems trained on both text and images, designed to enhance the process of image retrieval.
The Study
A groundbreaking study conducted by a collaborative team from MIT’s Computer Science and Artificial Intelligence Laboratory (CSAIL), University College London, and iNaturalist aimed to assess the capabilities of these VLMs in aiding ecological research. The team set up a test involving the “INQUIRE” dataset, which consists of 5 million wildlife images alongside 250 tailored search prompts sourced from ecologists and biodiversity experts.
The Struggle to Find Specifics
In their evaluations, the researchers discovered that while larger, more sophisticated VLMs could sometimes deliver relevant results for straightforward queries—such as identifying debris on a reef—they struggled significantly with more complex and specialized questions. For instance, while VLMs managed to locate jellyfish on the beach, they faltered when tasked with identifying “axanthism in a green frog,” a specific biological condition affecting skin coloration.
Insights into Model Performance
Using the INQUIRE dataset, the team could evaluate how effectively VLMs narrowed down a pool of 5 million images to the most relevant 100 results. Larger models, such as “SigLIP,” excelled at producing relevant matches for simple search queries, while smaller ones faltered. Nonetheless, even the most advanced models struggled with nuance; the top-performing model only achieved a precision score of 59.6% for complex searches.
Collaborative Efforts for Better Data
The INQUIRE dataset represents a significant collaborative effort, developed through extensive discussions with professionals from various ecological fields. Annotators spent 180 hours sifting through the iNaturalist dataset to generate search queries related to specific and rare wildlife events, such as a "hermit crab using plastic waste as a shell."
The Future of Biodiversity Research
With continued advancements, the hope is that VLMs like those tested in this study will not only bolster ecological research but also drive significant real-world impacts in conservation and environmental monitoring. As researchers refine these models, they will pave the way for a future where AI can unlock the hidden narratives within our planet's biodiversity and aid in its preservation.