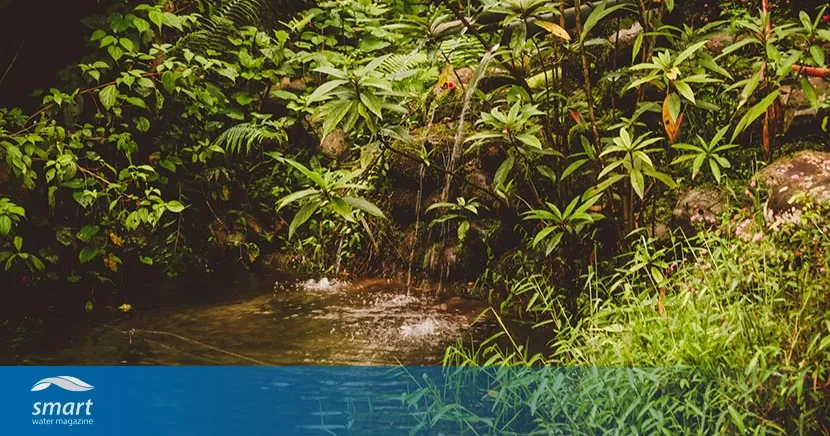
Machine Learning Breakthrough: New Tool Predicts Groundwater Contamination Risks!
2024-11-13
Author: Siti
Introduction
In a groundbreaking development for environmental science, an interdisciplinary research team has unveiled an innovative machine learning framework that predicts the presence of inorganic pollutants in groundwater, even with limited water quality samples. This cutting-edge tool has the potential to revolutionize water quality monitoring, enabling regulators and public health authorities to prioritize which aquifers require testing the most.
Scope of the Research
Focusing initially on Arizona and North Carolina, the model could eventually be adapted to address groundwater quality issues globally. Groundwater serves as a vital drinking water source for millions, yet many regions suffer from incomplete data on water quality—making this tool crucial for public health.
Challenges with Traditional Monitoring
“Traditional water quality monitoring is not only time-consuming but extremely expensive,” explains Yaroslava Yingling, a key contributor to the study and a distinguished professor at North Carolina State University. “Given the constraints on resources, it makes strategic sense to identify which groundwater sources are most in need of testing.”
Dataset Utilization
The researchers leveraged an extensive dataset of over 20 million data points gathered from 140 years of monitoring across 50 different water quality parameters in Arizona and North Carolina. Utilizing this historical data, the machine learning model predicts which inorganic pollutants might be present and in what concentrations, based solely on the parameters for which data is available.
Findings and Discrepancies
Remarkably, initial findings revealed a significant discrepancy between actual monitoring data and model predictions. While field data indicated that 75-80% of sampled sites were within acceptable contamination levels, the machine learning model suggests that only 15% to 55% of these locations may be genuinely risk-free. This points to a crucial need for additional testing in identified 'hot spots.'
Implications for Resource Allocation
Minhazul Islam, a Ph.D. student at Arizona State University and a co-author of the study, states, “By illuminating areas requiring immediate attention, state agencies can better allocate resources and implement targeted water treatment solutions.”
Future Work and Optimism
The researchers are optimistic about the model's real-world application but recognize that further testing is necessary to validate its predictions. Future plans include broadening the dataset to encompass more geographic regions, incorporating new data layers about environmental conditions, and conducting field tests to enhance the accuracy of groundwater safety measures.
Long-term Goals
“By continually refining this model, we aim to pave the way for proactive measures to ensure water safety around the world,” asserts Paul Westerhoff, a professor at Arizona State University involved in the research.
Additional Uses for the Model
Additionally, the model shows promise for monitoring phosphorus levels, a nutrient that can wreak havoc on ecosystems when overabundant. Jacob Jones, director of the National Science Foundation-supported Science and Technologies for Phosphorus Sustainability Center at NC State, emphasizes the model’s role in addressing potential contamination risks efficiently, fundamentally aiding in sustainable agricultural and environmental practices.
Conclusion
The implications of this research are profound, highlighting an empowering step towards enhanced public health safeguarding and the sustainable management of crucial groundwater resources.