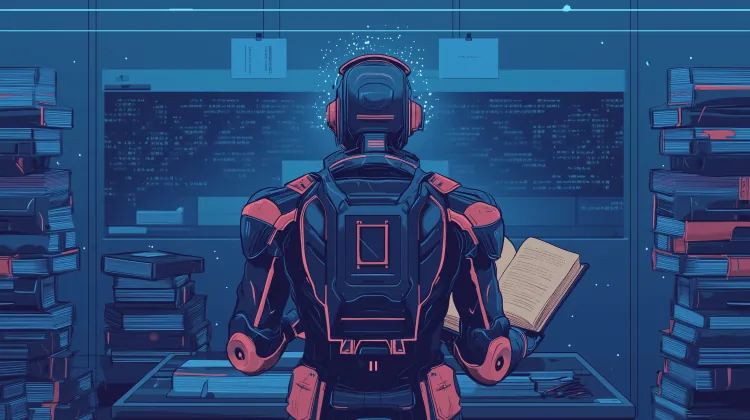
Is More Data Hurting Our AI? Researchers Uncover ‘Catastrophic Overtraining’ in Large Language Models
2025-03-28
Author: Arjun
A groundbreaking study has emerged from top academic institutions, revealing that the common belief that more pre-training data leads to enhanced performance in large language models (LLMs) might not hold true. This alarming research introduces the term "Catastrophic Overtraining," warning developers that excessive data can compromise the models' effectiveness.
Conducted by a team of esteemed researchers from Carnegie Mellon University, Stanford University, Harvard University, and Princeton University, the study, titled "Overtrained Language Models Are Harder to Fine-Tune," is now available on arXiv. Lead researcher Jacob Mitchell Springer and his collaborators highlight a troubling trend that challenges conventional wisdom in AI training.
The Law of Diminishing Returns
The study identifies an unexpected issue: models trained on increasingly larger datasets—often comprising millions of web-sourced tokens—risk diminishing accuracy during the fine-tuning process. While one might assume that adding more tokens would improve performance, the researchers found the opposite to be true in some scenarios.
For instance, they analyzed AI2’s OLMo-1B model, comparing its performance when trained on 2.3 trillion tokens versus 3 trillion tokens. Shockingly, the larger dataset resulted in over 2% worse performance on standardized language benchmarks. In some tasks, performance dipped even more severely, up to 3%.
Why Sensitivity Matters
So, what causes this performance drop? The researchers discovered that the additional training makes models more sensitive, leading to a phenomenon they call "progressive sensitivity." This increased fragility renders models more vulnerable to losing their previously acquired skills when fine-tuning is applied. Essentially, the more you train these models, the harder it becomes to adapt them without degrading their capabilities.
This over-sensitivity results in what the researchers describe as "forgetting," whereby new data replaces the model’s original strengths. Their findings indicated an "inflection point" in training; for the OLMo-1B model, this critical threshold appeared around 2.5 trillion tokens. Beyond this, added training can yield negative effects on subsequent fine-tuning.
Backed by Evidence
The study's conclusions are reinforced by extensive testing across various tasks and datasets, including instruction tuning and multimodal fine-tuning applications. The findings consistently showed a trend where models exceeded ideal token budgets underperformed when fine-tuned.
To solidify their hypothesis, the team even developed a theoretical model utilizing linear networks to explain why overtraining raises sensitivity levels. The mathematics indicated that catastrophic overtraining is likely inevitable if pre-training continues indefinitely without appropriate constraints.
Key Takeaways for Developers
The researchers emphasize the need for balance in AI training regimens, challenging the popular notion that more data is always better. Their research suggests that longer pre-training can enhance a model’s foundational abilities; however, it also heightens the risk of detrimental effects during fine-tuning.
For businesses looking to optimize their operations using LLMs, this research implies that they might achieve more reliable outcomes by fine-tuning lighter models that do not succumb to overtraining.
Looking Ahead: The Future of AI Models
With this enlightening study, the field of AI model development stands at a crossroads. It highlights critical considerations for researchers and developers about how to structure training and optimize performance while avoiding the pitfalls identified by the researchers.
As the quest for increasingly powerful LLMs continues, understanding the implications of Catastrophic Overtraining will be essential for creating robust and adaptive models that can truly revolutionize industries. Further investigations are necessary to explore variables, such as pre-training methods, optimizing for various tasks, and influencing data distributions, to navigate these complex training dynamics successfully.
This research marks a pivotal moment for the future of AI, urging stakeholders to reevaluate their strategies for developing high-performing, fine-tunable models.