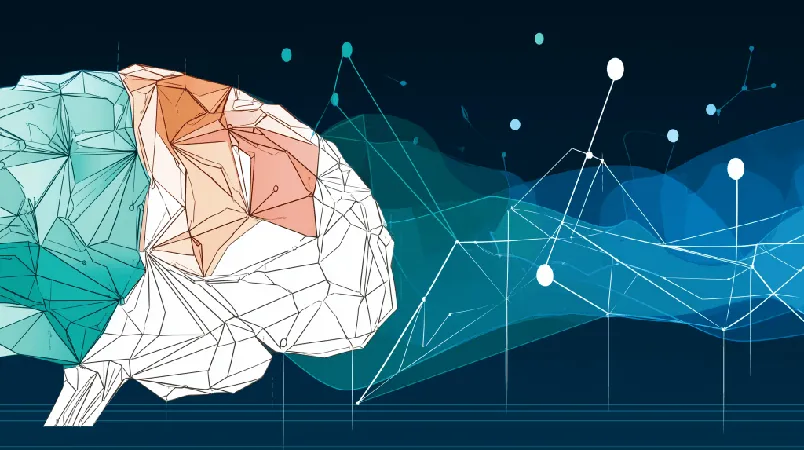
Introducing Sakana's Groundbreaking 'Continuous Thought Machines': A Leap Towards Human-like AI Reasoning!
2025-05-12
Author: Nur
The Revolutionary AI from Sakana
In an exciting development, Tokyo-based startup Sakana, co-founded by leading former Google AI scientists, has unveiled its cutting-edge model architecture known as Continuous Thought Machines (CTM). This innovative approach aims to revolutionize AI language models, making them more adaptable and capable of tackling diverse cognitive challenges—think navigating complex mazes or solving problems without unnecessary prompts—in a way that mirrors human thought processes.
How CTMs Resemble Human Thinking
Unlike traditional Transformer models that process data in fixed layers simultaneously, CTMs unfold their computations over time within each unit, or 'neuron.' Each neuron retains a history of its previous activity, which guides its future decisions about when to activate. This thoughtful internal state allows CTMs to dynamically adjust their reasoning time and depth, setting them apart with a richer informational capacity.
What Sets CTMs Apart from Transformers?
While most large language models still hinge on the foundational 'Transformer' architecture detailed in Google Brain's famous 2017 paper, CTMs introduce a unique time-based structure. Each neuron operates on its own internal timeline, making activation decisions based on its memory history. This flexibility allows the model to deepen its reasoning when necessary, adapting to the complexities of varying tasks.
CTMs utilize 'ticks' to represent internal steps—deciding how many to take based on input complexity. This approach is a notable shift from conventional deep learning models, steering closer to a more biologically-inspired intelligence that processes information contextually and adaptively.
Key Innovations in CTM Architecture
The CTM leverages two groundbreaking mechanisms. First, each neuron maintains a short history or working memory to inform its next activation. Second, neural synchronization occurs organically. Neurons communicate internally to decide when to process information collectively, enhancing attention on critical data points.
Thanks to these features, CTMs manage to conserve computational resources on simpler tasks while engaging in deeper thought processes for more challenging ones. Demonstrations of this model, including maze navigation and reinforcement learning, have highlighted its transparency in decision-making, a rarity in current AI systems.
CTMs: Early Performance Compared to Transformers
Although CTMs are not aimed at achieving maximum benchmark scores, early results from standardized assessments are promising. On the renowned ImageNet-1K benchmark, CTMs achieved competitive accuracies of 72.47% top-1 and 89.89% top-5. While these scores may lag behind the latest Transformer models, they emphasize CTMs' unique design and adaptability.
In maze-solving tasks, for example, CTMs generate systematic outputs from raw images without relying on positional embeddings, showcasing a human-like sequence of cognition. The model’s ability to gauge its own confidence also stands out, naturally aligning predictions with accuracy without requiring costly adjustments.
The Road Ahead for CTMs
Despite their impressive capabilities, CTMs are still experimental. Sakana emphasizes that this model serves as a foundation for ongoing research rather than a ready-for-market solution. Currently, training these models demands more resources than traditional Transformers, largely due to their varied temporal structure.
However, Sakana's commitment to community engagement shines through with the open-sourced CTM architecture. Researchers are encouraged to explore its potential through GitHub, featuring resources like pretrained checkpoints and training scripts.
What Enterprises Should Know About CTMs
With enterprise applications on the horizon, decision-makers should pay attention to CTMs' advantages in adaptive computation and enhanced interpretability. Their unique reasoning structure may prove invaluable for organizations dealing with fluctuating input complexities or strict regulatory standards.
Moreover, the architecture’s compatibility with existing systems makes it a flexible option for seamless integration into current workflows.
A Vision for the Future of AI
Sakana's commitment to merging evolutionary computation with modern AI reflects a desire for models that learn and adapt in real time, much like biological organisms in an ecosystem. This vision is evident in their various initiatives, aiming not just to compete but to collaborate with the broader AI research community.
As the landscape of AI evolves, Sakana stands out as a pioneer advocating for systems that think, evolve, and learn—paving the way for a future where AI can reason like humans.