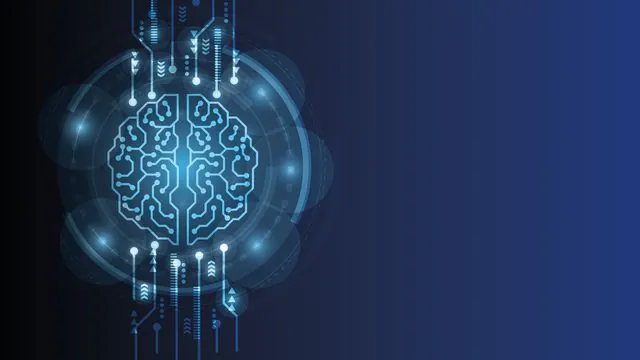
How Diverse Data in Machine Learning is Revolutionizing Predictive Models for Mental Health
2024-11-04
Author: Nur
How Diverse Data in Machine Learning is Revolutionizing Predictive Models for Mental Health
In the field of neuroimaging, one of the ultimate goals is to connect brain activity to behavior. This pursuit not only enhances our understanding of how the brain influences behavior but also paves the way for personalized treatments catered to mental health and neurological conditions. Researchers often employ brain scans and behavioral data to teach machine learning models that can predict an individual’s symptoms or possible illnesses based on brain function. However, the true power of these models lies in their ability to generalize — that is, to make accurate predictions across varying settings and diverse populations.
A recent study from Yale University has unveiled groundbreaking insights into this matter, highlighting that predictive models can indeed perform impressively well when tested against datasets that differ significantly from the ones used for training. The lead author of the study, Brendan Adkinson, emphasizes that such testing is critical for nurturing clinically applicable predictive models, as detailed in their findings published in the journal *Developmental Cognitive Neuroscience*.
Adkinson notes, "It's quite common for predictive models to excel when evaluated on data that closely resembles their training sets. However, these models often falter when faced with datasets that possess different characteristics, rendering them ineffective for real-world applicability." This diminished performance stems from various factors, including differences in age, sex, race, ethnicity, geographical location, and the specific clinical symptoms presented by individuals in the different datasets.
Instead of perceiving these variances as obstacles, researchers like Adkinson propose regarding them as fundamental elements of model development. “For predictive models to hold clinical value, they must demonstrate effective prediction capabilities despite dataset-specific peculiarities,” he explains.
In their research, the Yale team trained machine learning models to predict two cognitive traits: language abilities and executive function. They utilized three large datasets that were distinctly different from one another. Each model was trained on one of the datasets and subsequently tested against the other two. Remarkably, despite the substantial differences between the datasets, the models showcased commendable performance in line with neuroimaging standards. Adkinson revealed, “This indicates that creating generalizable models is not only feasible, but testing with diverse features is paramount to their success.”
Looking ahead, Adkinson is eager to delve deeper into generalizability, particularly within specific populations. A significant concern lies with the data primarily collected from urban and suburban settings; this raises the risk of developing models that may not apply to individuals living in rural areas. Adkinson, who hails from a rural background himself, cautions, “If our predictive models prove robust enough for clinical use yet fail to generalize to certain demographic groups, such as those in rural locales, it compromises the quality of care for these populations.”
The research conducted by Adkinson and his team opens a window into the potential future of mental health predictions, signifying that embracing diversity in data is not just beneficial, but essential for equity in clinical applications. Imagine a world where psychiatric and neurological treatments are not only innovative but also tailor-made to meet the unique needs of every corner of society!
In conclusion, as researchers continue to refine these models with diverse datasets, we may well be on the brink of a revolution in mental health care — where predictions are not just accurate, but also inclusive, ensuring better outcomes for all communities.