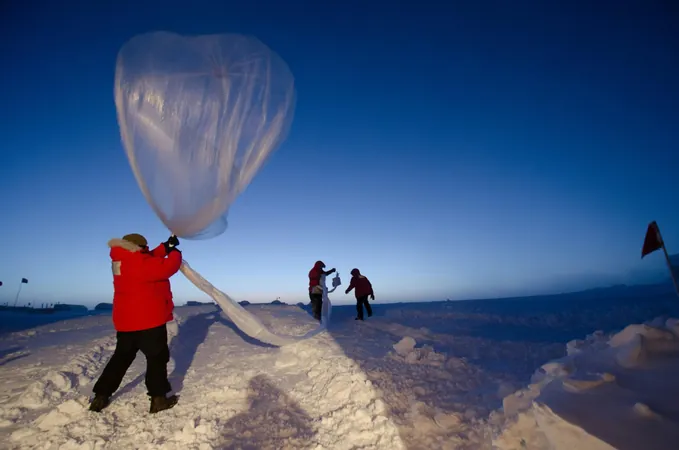
How AI is Revolutionizing Climate Science: The Incredible Impact of Machine Learning on Climate Physics
2025-01-23
Author: Nur
Introduction
The explosive growth in big data and computing capabilities is fundamentally reshaping the landscape of climate science. At the forefront of this transformation is machine learning (ML), a technology that is playing a pivotal role in mapping the intricate physics of our ever-changing climate.
Dr. Annalisa Bracco, Associate Chair and Professor at the School of Earth and Atmospheric Sciences, describes the ongoing developments in the field as "revolutionary." She emphasizes that a multitude of climate-related processes—ranging from ocean currents and melting glaciers to intricate weather patterns—can now be articulated using physical equations, thanks to advancements in machine learning. This enables researchers to uncover critical insights and enhances our capability to predict climate changes with greater accuracy.
Bracco is the lead author of a groundbreaking review paper that delves into the dynamic interplay between artificial intelligence and climate physics. This research is the culmination of a collaborative effort among esteemed scholars from various institutions, including Georgia Tech, Nansen Environmental and Remote Sensing Center, Utrecht University, the University of Chicago, European Center for Medium-Range Weather Forecasts, and the University of Colorado Boulder. The paper, titled "Machine Learning for the Physics of Climate," has been published in the prestigious journal *Nature Reviews Physics*.
Bridging the Knowledge Gap
One of the primary objectives of this research team was to foster a deeper understanding of how climate science interconnects with AI technologies. Bracco explains, "Machine learning is enabling us to investigate climate physics in ways that were previously unattainable. The increasing amounts of available data and observations now allow us to analyze climate at unprecedented scales and levels of detail."
The paper identifies three crucial areas where machine learning is making a noteworthy impact: addressing data gaps, enhancing the robustness of climate models, and improving predictions, particularly in the domain of weather forecasting. Nonetheless, the research also indicates the limitations of AI and highlights areas where researchers must focus their efforts to bridge these gaps.
According to Bracco, "Machine learning has been instrumental in extending the temporal and spatial scales of our measurements." It allows scientists to fill in missing data points, providing a more comprehensive dataset for climate research. However, she cautions that machine learning is not a panacea; it is most effective when there is a solid foundational data set to build upon.
"While ML can extrapolate from past abundant data, it currently lacks the ability to predict future climate trends or gather all the necessary data independently," she adds. "To push forward, we need scientists capable of identifying and collecting the right data and innovatively addressing existing challenges."
Innovative Climate Models and Enhanced Forecasting
Machine learning also plays a critical role in refining climate models that simulate complex systems, including the atmosphere, oceans, land interactions, biochemistry, and ice dynamics. "Current models face limitations due to computational constraints, as they often utilize a three-dimensional grid," Bracco states. Below this grid resolution, researchers resort to simplifying complex physical phenomena, a practice known as "parameterization."
Machine learning offers promising new strategies for improving these parameterizations, allowing models to operate at higher resolutions over shorter periods without excessive simplifications. "We can apply machine learning for deriving equations that better represent smaller-scale physical processes," she explains. "These refined equations can then be employed in larger models that can simulate climate over hundreds of years."
While a fully ML-based climate model remains a future aspiration, the research illustrates that machine learning is enhancing our ability to forecast weather phenomena, including El Niño events, with remarkable precision.
In the traditional model of weather prediction, knowledge of initial conditions—like temperature, humidity, and pressure—was essential. Now, machine learning enables researchers to utilize past data and recognize patterns from historical scenarios to predict future outcomes without relying exclusively on complex physical equations. "This process allows for significant reductions in the computational resources typically required," notes Bracco.
The Power of Human Collaboration
Despite the significant contributions of AI and ML in spearheading climate research, Bracco is clear that human collaboration remains vital to progress. "The in-person teamwork that drove this paper reflects the necessity of human connections," she recalls, reflecting on a workshop at the Kavli Institute for Theoretical Physics, which marked a monumental moment of gathering post-pandemic.
She emphasizes, "While machine learning is an exceptional tool, it is not a catch-all solution. There is an undeniable need for human researchers who can gather high-quality data and foster interdisciplinary collaborations among fields such as computer science, physics, mathematics, biology, and chemistry." This collaboration, she believes, represents both a significant challenge and an incredible opportunity to tackle the pressing issues of climate change.
By leveraging the strengths of machine learning and interdisciplinary efforts, we are on the threshold of potentially groundbreaking advancements in our understanding of climate science. The invaluable synergy of AI and human creativity might just be the key to unlocking innovative solutions for the planet’s most pressing environmental challenges.