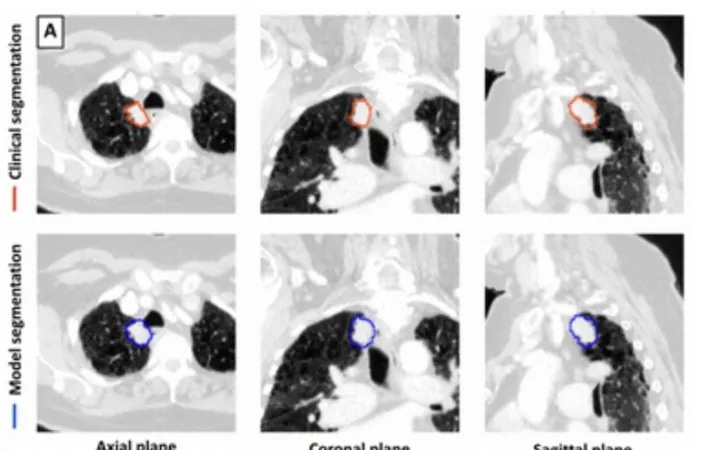
Groundbreaking Study Reveals AI's Potential in Lung Tumor Detection Using CT Scans!
2025-01-21
Author: Mei
A new groundbreaking study has just emerged, showcasing how deep learning technologies can revolutionize the detection and segmentation of lung tumors through computed tomography (CT) scans. The study, recently published in the esteemed journal Radiology, sheds light on the capabilities of a sophisticated AI model, offering both enhanced sensitivity and significant time savings for medical professionals.
In this retrospective study, researchers evaluated the deep learning model on a diverse dataset comprising 150 chest CT cases, which included 41 scans used for internal testing, 59 for external validation, and 50 derived from a non-tumor set. Impressively, the AI model was trained using over 1,500 CT scans, ensuring its robustness and adaptability across different clinical settings.
Staggering Results:
The findings reveal that the deep learning model achieved an impressive sensitivity rate of 92% and a specificity rate of 82% for diagnosing lung tumors. Lead author Dr. Mehr Kashyap, affiliated with Stanford University School of Medicine, emphasized the model's impressive consistency, stating, "Despite variations in scanning devices and clinical practices, the deep learning model showed strong performance, indicating its capability to generalize well across different environments."
Further analysis indicated that the AI technology offered a significant reduction in segmentation time—up to 59%. While manual segmentation typically ranged from 166.1 to 187.7 seconds, the AI completed the task in just 76.6 seconds on average, marking a leap in efficiency for oncological workflows.
Key Insights from the Study:
1. Enhanced Accuracy and Efficiency: The AI's remarkable sensitivity rate of 92% and specificity of 82% highlights its potential to aid in precise lung cancer diagnoses, while the 59% reduction in segmentation time suggests dramatic improvements in clinical efficiency.
2. Robust Performance Across Settings: Trained on a large and heterogeneous dataset, the model achieved a median Dice similarity coefficient (DSC) of 77%, indicating its stable performance even when operating across various hospitals and imaging equipment.
3. Challenges with Specific Tumor Types: Despite its strengths, the AI model faced challenges in accurately detecting larger tumors (above 50 cm³) and those with multiple tumors, with a DSC of just 70% and a sensitivity of 67% in such cases. This highlights the need for continued refinement.
Dr. Kashyap outlined the significant implications of this study, stating, "Rapid and accurate tumor segmentation can transform the way we assess tumor burden and treatment responses in oncology, potentially overshadowing current labor-intensive methods like RECIST that rely on subjective and limited measurements."
Though the findings are promising, the authors acknowledged certain limitations inherent to retrospective studies, such as the potential underestimation of tumor volume by the AI model, and the reduced effectiveness with very large tumors. Importantly, the model's ability to segment tumors related to atelectasis and those near soft tissue structures was not evaluated, revealing opportunities for further research.
As the medical community eagerly watches these developments, the potential for AI-powered tools to optimize cancer diagnostics promises a future where detection is not only faster but also more accurate— a significant leap forward in the fight against lung cancer! Stay tuned for further updates on this transformative technology!