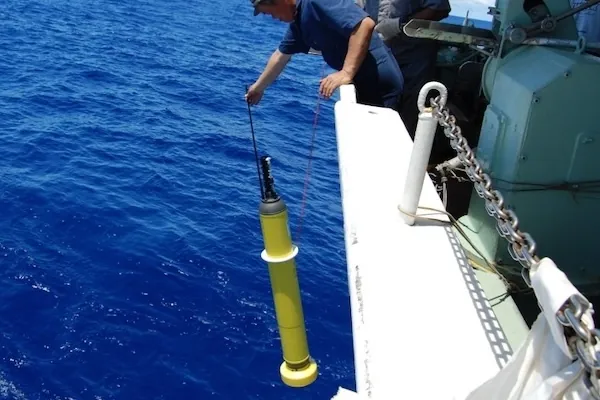
Groundbreaking Machine Learning Technique Reveals Alarming Decline of Ocean Oxygen Amid Climate Change
2024-11-14
Author: Arjun
Introduction
In a stunning breakthrough, researchers at Georgia Tech have unveiled a revolutionary machine learning technique that could reshape our understanding of the diminishing oxygen levels in the ocean—a worrying trend posing a significant threat to marine habitats worldwide.
Importance of Oxygen in the Ocean
Oxygen, which is essential for the survival of marine life, is generated by photosynthesis in surface waters performed by plants and algae, both terrestrial and marine. Unfortunately, recent data indicates that oxygen concentrations are plummeting in various ocean regions. Scientists attribute this decline to rising surface temperatures affecting the chemical properties of seawater. As temperatures increase, the water's ability to hold oxygen decreases, creating an intricate problem that Georgia Tech's innovative approach is designed to tackle.
Challenges in Measuring Ocean Oxygen Levels
“Determining the extent of oxygen depletion in the oceans has been a complex task due to sparse historical measurement data and inconsistent collection times,” noted Taka Ito, an oceanographer and professor in the School of Earth and Atmospheric Sciences at Georgia Tech. “To comprehend the global status of oxygen levels and their fluctuations, we need to bridge significant data gaps.”
Developing a Machine Learning Method
To overcome this challenge, a team of graduate student researchers led by Ito developed a cutting-edge machine learning method aimed at producing a clearer picture of the oceans' declining oxygen levels. By meticulously analyzing multiple datasets, the team crafted a comprehensive monthly map detailing oxygen content declines over several decades. Their research, recently published in the Journal of Geophysical Research: Machine Learning and Computation, provides a vital tool for assessing one of the most pressing threats facing marine ecosystems today.
Significance of Machine Learning in Oceanography
“Marine scientists require a thorough understanding of the spatial distribution of oxygen in the ocean, the extent of its changes, the locations of these changes, and the underlying reasons,” explained Ahron Cervania, a Ph.D. student in Ito's lab. “While traditional statistical methods have been employed for such estimates, machine learning techniques significantly enhance both the accuracy and resolution of these assessments.”
Project Background and Methodology
Launched three years ago with support from the National Science Foundation, the project initially targeted data from the Atlantic Ocean to fine-tune the machine learning model. Through sophisticated computational simulations, the team tackled the challenge of missing oxygen observations, enabling them to evaluate the model's effectiveness in reconstructing data from limited inputs.
Once this approach demonstrated its reliability, the researchers broadened their focus to encompass global observations. They developed advanced algorithms to analyze oxygen levels in conjunction with temperature, salinity, and pressure. By leveraging historical ship data from as early as the 1960s alongside recent measurements collected from Argo floats, the team successfully created a global monthly map of ocean oxygen levels extending from 1965 to the present.
Findings and Implications
“Our machine learning method has allowed for a more precise assessment of the rate of oxygen loss across various time periods and locations,” Cervania remarked. “Our results show that incorporating float data significantly enhances our estimates while minimizing uncertainty.”
The team discovered that global ocean oxygen levels have decreased at an alarming average rate of approximately 0.7 percent per decade between 1970 and 2010—pointing to a swift reaction to contemporary climate changes with dire implications for marine ecosystem health. This rate corresponds closely with findings of other studies, underscoring the effectiveness and accuracy of their machine learning approach.
Validation of Findings
“We evaluated trends in global oxygen levels and the overall oceanic oxygen inventory, essentially tracking the rate of change over the last fifty years,” Cervania said. “It’s encouraging to see our rate align with estimates derived from various methods, which reinforces our confidence. We are establishing a robust estimate that incorporates both our findings and those of related studies.”
Conclusion
Ito emphasized that the method addresses a long-standing challenge in oceanography: the integration of diverse data sources, each possessing varying degrees of accuracy and uncertainty, to better understand ongoing oceanic changes.
“The incorporation of progressive technologies like machine learning is critical in bridging data gaps, providing invaluable insights into how our oceans are responding to climate change,” he stated.
As awareness grows around the urgent threats posed by climate change, this cutting-edge research underscores the critical need to monitor and address ocean oxygen loss, possibly paving the way for sustainable solutions to protect our planet’s marine ecosystems.