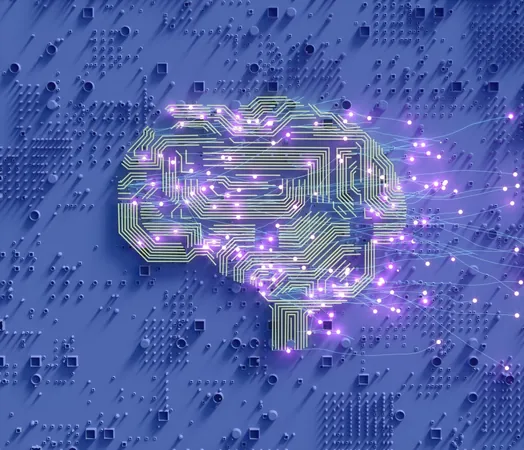
Groundbreaking AGI Challenge Leaves Top AI Models in the Dust
2025-03-25
Author: Arjun
In a significant move for artificial intelligence research, the Arc Prize Foundation, co-founded by the esteemed AI researcher François Chollet, unveiled a revolutionary test designed to evaluate the general intelligence of leading AI models. Dubbed ARC-AGI-2, this challenging assessment has perplexed even the most advanced AI technologies available today.
Initial results from the ARC-AGI leaderboard reveal that traditional “reasoning” models, including OpenAI’s o1-pro and DeepSeek’s R1, have managed to score only between 1% and 1.3% on the new test. Meanwhile, high-performance non-reasoning models such as GPT-4.5, Claude 3.7 Sonnet, and Gemini 2.0 Flash also stumbled, achieving approximately 1%.
So, what makes this test so demanding? The ARC-AGI assessments consist of intricate, puzzle-like problems that require AI to discern visual patterns from an array of colored squares and generate the correct output grid. Crucially, the problems are designed to compel AI systems to adjust to scenarios and challenges they have not previously encountered, distinguishing them from their predecessors.
To set a benchmark, over 400 participants were invited to take the ARC-AGI-2 test, resulting in a human baseline score where panels averaged an impressive 60% accuracy. In contrast, these human subjects demonstrated vastly superior problem-solving abilities compared to the AI models.
Chollet emphasized that the ARC-AGI-2 test serves as a more reliable measure of an AI model's true intelligence compared to its predecessor, ARC-AGI-1. The focus of the tests is on evaluating an AI's ability to learn new skills outside its training data effectively. Notably, the introduction of a new efficiency metric in ARC-AGI-2 aims to reduce reliance on "brute force" computing power to find solutions – a significant flaw identified in the earlier iteration.
As Greg Kamradt, co-founder of the Arc Prize Foundation, highlighted in a recent blog post, "Intelligence is not solely defined by the ability to solve problems or achieve high scores. The efficiency with which those capabilities are acquired and deployed is a crucial, defining component." This redefined focus raises the question: It’s not just about whether AI can solve a task, but how efficiently they can do so.
The introduction of ARC-AGI-2 highlights a growing demand within the tech industry for innovative benchmarks that can more accurately measure AI's progress towards true general intelligence. Notably, Thomas Wolf, co-founder of Hugging Face, recently shared concerns about the lack of sufficient tests in the AI industry, which fail to assess critical aspects of artificial general intelligence like creativity and adaptability.
As the quest for AGI continues, the arrival of the ARC-AGI-2 test marks a pivotal point in evaluating AI capabilities. The pressure is now on leading AI developers to bridge the daunting gap between human and artificial intelligence. Will they rise to the challenge, or will this new test redefine the landscape of AI research forever?