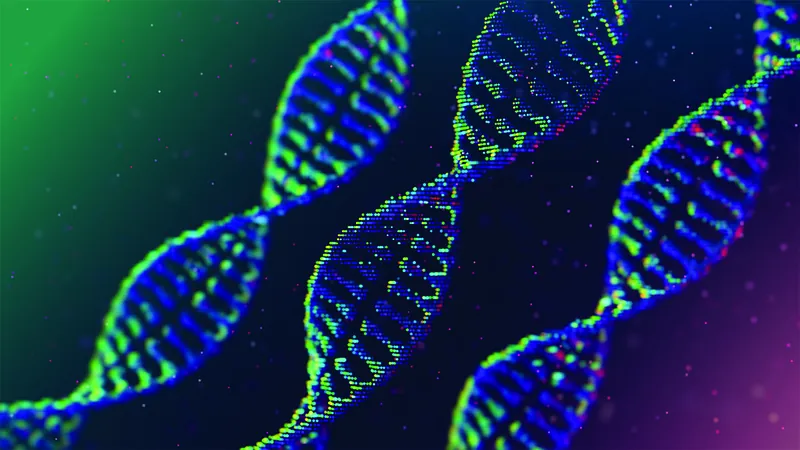
Evo AI Model: The Revolutionary “Rosetta Stone” Decoding Our Genetic Code
2024-11-15
Author: Li
Evo AI Model: The Revolutionary “Rosetta Stone” Decoding Our Genetic Code
A groundbreaking artificial intelligence model named Evo has emerged as a game-changer in the field of genetics, boasting the ability to decode and engineer genetic sequences. With its cutting-edge technology, Evo can manipulate cell functions, create new genes and protein sequences, and even innovate the existing CRISPR gene-editing system. This capability positions Evo as an incredibly powerful tool for diagnosing diseases and developing therapeutics.
Published in the prestigious journal Science, Evo is a multimodal machine learning model trained on an impressive dataset of 2.7 million evolutionarily diverse microbial genomes. This extensive training enables it to decode and create DNA, RNA, and protein sequences from molecular to genomic scales with unmatched accuracy, marking it as the first foundation model capable of analyzing DNA at such a scale. The Arc Research Institute in Palo Alto, where Evo was developed, has referred to it as the 'Rosetta Stone' for biology, due to its profound implications for genetic research and synthetic biology.
Evo’s predictive capabilities offer groundbreaking insights into biological processes. It can anticipate the impact of mutations across various cellular regulations while designing DNA sequences that could significantly enhance cell function. Christina Theodoris, PhD from the Gladstone Institute of Data Science and Biotechnology in San Francisco, noted that this technological leap could revolutionize disease diagnostics and treatments, saying, 'The ability to predict the effects of mutations would have tremendous diagnostic and therapeutic implications for disease.'
One of Evo’s most astonishing feats includes the development of a fully synthetic CRISPR gene editing tool. This innovative model can design a guide RNA that amplifies the efficiency of the CRISPR-associated protein 9 (Cas9) enzyme, which acts as genetic scissors, cutting DNA with precision. Furthermore, Evo is capable of constructing DNA sequences that exceed one million base pairs in length—a scale comparable to many real genomes.
Utilizing advanced deep learning techniques, Evo processes vast lengths of genetic data efficiently, facilitating a deep understanding of the complex interactions within the genetic code. Eric Nguyen, PhD from the Arc Institute, and his team have trained this large-scale biological sequence model on trillions of DNA nucleotides from a diverse range of organisms, establishing a nuanced understanding of DNA’s grammar that transcends single organism studies.
This model not only predicts how minor DNA alterations can impact an organism's evolutionary fitness but also generates realistic genomic sequences that can exceed one megabase—a significant advancement over previous models. Evo can even create new biological systems, further validated by laboratory assessments of synthetic CRISPR tools and other biological elements such as IS200 and IS605 transposons.
Evo operates at an intricate level of detail, analyzing sequences at single-nucleotide resolution. By doing so, it captures the complex information landscape inherent in DNA and synthesizes two critical aspects of biology: the interplay of DNA, RNA, and proteins—known as the central dogma of molecular biology—and the diverse scales at which evolution operates.
Armed with seven billion parameters and employing leading-edge deep learning architectures, Evo unravels the nuanced coevolution between coding and noncoding sequences while designing sophisticated biological systems like CRISPR-Cas complexes.
The researchers emphasize that ongoing advancements in large-scale biological sequence models like Evo, coupled with innovations in DNA synthesis and genome engineering, will accelerate our capacity to design and shape life itself. This promises not only to alter the landscape of genetic research but could lead to solutions for some of the most pressing health challenges of our time.