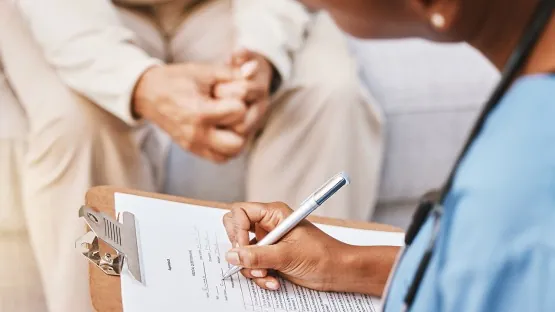
Considering Race Could Revolutionize Colon Cancer Predictions and Bridge Healthcare Gaps
2024-09-19
Introduction
In a groundbreaking study, researchers reveal that factoring in race when creating tools to predict colorectal cancer risk significantly enhances prediction accuracy over race-neutral algorithms. This insight comes amidst ongoing debates in the medical field about whether race should be considered a factor in clinical risk assessments.
Importance of Race in Colorectal Cancer Predictions
While some experts advocate for removing race from clinical algorithms, the new research underscores the potential benefits of including it, particularly for Black patients who often have poorly documented family histories of colorectal cancer. The study suggests that integrating race into predictive models can help identify those who would benefit most from screenings and preventive care.
Expert Insights
Emma Pierson, the lead author and a notable figure at Cornell Tech, emphasizes that neglecting to use race in these algorithms can lead to flawed conclusions. "If you don't use race, you're telling your algorithm that family history is equally useful for everyone, which is simply false," she stated. This perspective was part of a broader team effort that also included Anna Zink from the University of Chicago and Ziad Obermeyer from UC Berkeley, culminating in a study published in the *Proceedings of the National Academy of Sciences*.
Study Overview
The research focused on a cohort of 77,836 diverse participants, aged 40 to 74, recruited for insights into cancer causation through the Southern Community Cohort. The team devised two algorithms for predicting colorectal cancer risk; one incorporated race and the other did not. Using a wide array of risk factors—including age, weight, dietary habits, and medical history—the researchers compared the effectiveness of both algorithms across racial lines.
Findings
The findings were striking. Many Black participants reported unknown family cancer histories, indicating systemic data recording issues. The race-neutral algorithms not only underestimated cancer risks for Black individuals but also overestimated risks for their white counterparts. Conversely, the race-inclusive model demonstrated enhanced predictive accuracy, identifying a staggering 74.4% of the highest-risk participants as Black, a notable increase from the 66.1% identified by the race-blind algorithm.
Implications for Healthcare Access
This distinction is crucial because those identified as high-risk typically gain improved access to screenings and healthcare services, potentially saving lives through early intervention. Furthermore, Pierson highlights the necessity of revisiting race's role in healthcare algorithms as incorrect assumptions can lead to severe health disparities. Such disparities have historically resulted in Black patients facing obstacles in receiving optimal care, such as delayed access to kidney transplants or breast cancer screenings.
Cautions and Future Directions
However, Pierson cautions that while integrating race in these algorithms can rectify existing biases, we must also urge for advanced data quality improvements. "The goal should be to develop algorithms that are accurate based on the data available at present, but we must also work diligently to enhance the quality of medical records," she advocates.
Conclusion
As healthcare continues to evolve, understanding and addressing these discrepancies is vital for bridging gaps in health equity, ultimately leading to better outcomes for all communities. The fight against inequity in healthcare may be ongoing, but studies like these illuminate a path forward.