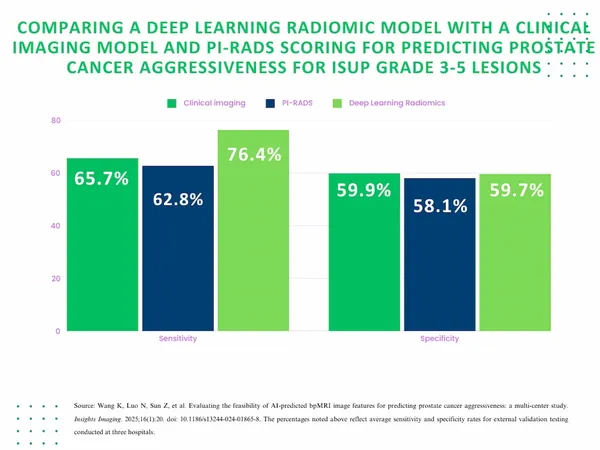
Can Deep Learning Radiomics with bpMRI Revolutionize Prostate Cancer Prognosis?
2025-01-22
Author: Wei Ling
Recent advancements in medical imaging have introduced a groundbreaking study that demonstrates how a deep learning radiomics model, utilizing biparametric magnetic resonance imaging (bpMRI) scans, significantly outperforms traditional methods for predicting prostate cancer progression. This innovative approach could change the landscape of prostate cancer diagnosis and treatment.
The research, published in the esteemed journal "Insights into Imaging," involved a comprehensive comparison between the deep learning radiomic model and established diagnostic methods, including PI-RADS scoring and conventional radiomic models. In this extensive study, 878 prostate cancer patients who underwent radical prostatectomy were analyzed, and the findings are nothing short of impressive.
During the validation phase, researchers noted that the deep learning radiomics model achieved an area under the curve (AUC) ranging from 70% to 79.1% for predicting prostate cancer progression. In contrast, traditional methods struggled, with AUCs of only 59.7% to 71.8% for clinical imaging and 55.4% to 61.3% for PI-RADS scoring. This remarkable performance highlights the potential of deep learning technology in improving diagnostic accuracy.
Specifically, the deep learning model demonstrated a more than 10% increase in sensitivity when assessing ISUP grade 3 to 5 lesions. The study revealed a sensitivity rate of 76.4% for the model compared to 65.7% for the clinical imaging method and 62.8% for PI-RADS. This significant enhancement in sensitivity may empower healthcare providers to identify aggressive cancer forms earlier and more reliably.
Co-author Dr. ZiXuan Kong from the Second Affiliated Hospital of Dalian Medical University in China emphasized that this model offers an objective and non-invasive diagnostic alternative. By reducing the need for unnecessary biopsies, it could minimize overtreatment and its associated side effects, thereby improving overall patient management strategies.
Three Key Takeaways:
1. **Enhanced Prognostic Accuracy**: The deep learning model outshines traditional methods in predicting prostate cancer progression, especially for higher-grade lesions (ISUP grade 3-5). This improvement could lead to early interventions, enhancing patient outcomes.
2. **Potential to Reduce Overtreatment**: The model's non-invasive nature presents a significant advantage, potentially reducing unnecessary biopsy procedures and focused treatment efforts on cases that truly require it.
3. **Robust Generalizability**: The deep learning radiomics model exhibited strong predictive consistency across multiple hospitals and different MRI settings, indicating its applicability in diverse clinical environments.
Despite its promising results, the study identifies certain limitations, such as the relatively small cohort size and the need for better categorization of prostate cancer lesions based on their anatomical locations. Nevertheless, the results pave the way for future research that could refine these methodologies and their applications in clinical practice.
In conclusion, as the medical community continues to embrace deep learning technologies, this study stands as a beacon of hope, potentially transforming how prostate cancer is evaluated and treated. The future may hold less invasive, more accurate, and more individualized prostate cancer care, ultimately improving survival rates.