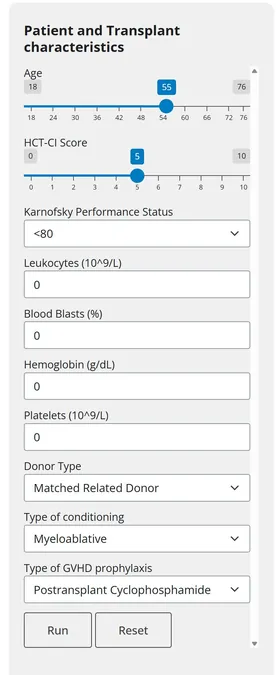
Breakthrough Machine Learning Model Revolutionizes Transplant Risk Assessment for Myelofibrosis Patients!
2025-03-27
Author: Arjun
A cutting-edge machine learning model developed by researchers from the European Society for Blood and Marrow Transplantation (EBMT) demonstrates superior performance over traditional statistical methods in assessing transplant risk for patients suffering from myelofibrosis. This groundbreaking study, recently published in the journal Blood, signals a new era in personalized medicine for blood cancer patients.
"While many models exist to pinpoint high-risk myelofibrosis patients, a significant gap remains in tools designed to evaluate transplant risk specifically," stated Dr. Juan Carlos Hernández Boluda, a leading hematologist at Hospital Clínico in Valencia and a prominent figure in EBMT's myeloproliferative neoplasms committee. "Our innovative tool offers a comprehensive approach to identifying patients at elevated risk of mortality post-transplant, allowing for strategic planning that could substantially improve patient outcomes."
Myelofibrosis is a rare and complex blood cancer characterized by abnormal bone marrow stem cells that produce excess blood cells and inflammatory factors. This condition leads to fibrosis, or scarring, in the bone marrow, resulting in symptoms such as anemia and splenomegaly (enlarged spleen), with stem cells often migrating to the spleen and liver.
Although various therapeutic options are available, allogeneic hematopoietic cell transplantation (allo-HCT) remains the sole curative intervention, wherein healthy stem cells from a donor replace the dysfunctional cells in the patient. However, only about 10% of myelofibrosis patients undergo transplant procedures due to the intricate decision-making process and a lack of reliable risk assessment tools, as lamented by Dr. Hernández Boluda.
This ambitious research aimed to enhance transplant eligibility assessments by focusing on overall survival predictions. The study analyzed data from 5,183 adults who had undergone their first allo-HCT from 2005 to 2020 across 288 EBMT-registered centers. Of these, 3,887 records were utilized to train the machine learning algorithm, while 1,296 records were reserved for model validation.
Through rigorous analysis, the research team employed the Kaplan-Meier method to estimate overall survival and evaluate non-relapse mortality. They also deployed independent statisticians to incorporate Cox regression methods and machine learning techniques within the same dataset, developing stratified prognostic models for post-transplant mortality risks.
By leveraging state-of-the-art random survival forests, the machine learning model emerged with a higher concordance index for predicting overall survival and non-relapse mortality compared to three alternative methods. Its precision illuminates a distinct subset of high-risk patients, with a staggering 40% likelihood of dying within a year post-transplant—translating to 25% of myelofibrosis patients.
To empower clinicians, the research team created a user-friendly, open-access web-based calculator. This predictive tool assesses a patient’s likelihood of survival post-transplant by analyzing ten critical characteristics, including patient age, overall health status, existing health conditions, and specific treatment factors.
"Clinicians can utilize this innovative calculator to engage in shared decision-making with their patients," remarked Dr. Donal McLornan from University College London Hospitals. “This tool is practical and straightforward, utilizing data that any transplant clinician already possesses."
Dr. Adrián Mosquera, another key researcher, emphasized the three attributes that make this model a landmark in medical AI: broad applicability, high accessibility, and clinical actionability. The tool not only aids medical teams in determining appropriate cell therapy options but also supports informed discussions between clinicians and patients.
While the study set a remarkable precedent, researchers noted limitations, mainly regarding missing data from the patient registry. They plan to integrate real-world data into the model for continual refinement. As this technology advances, the future of transplant risk assessment for myelofibrosis caregivers and patients is poised to become more secure and well-informed.
In a world of medical advancements, this machine learning model serves as a beacon of hope for countless patients battling myelofibrosis, ensuring that fewer lives are lost in the complex decision-making around transplant eligibility. Don’t miss out on following this revolutionary development that could redefine hematology practices!