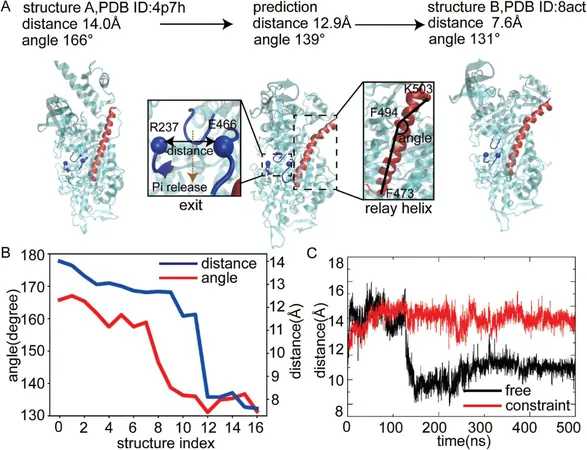
Breakthrough in Protein Research: Team Unveils Deep Learning Model to Predict Protein Conformational Changes!
2024-10-28
Author: Wei Ling
Introduction
In an exciting development in the field of biochemistry, a research team has introduced a groundbreaking deep learning model designed to predict the conformational changes of proteins. Their findings have been published in the esteemed journal, Advanced Science, marking a significant leap forward in our understanding of protein dynamics.
Enhancing Protein Dynamics Understanding
While the AI-driven tool AlphaFold has made headlines for accurately predicting static protein structures, the new deep learning model addresses a critical aspect of protein function: the dynamic characteristics dictated by conformational changes. These changes play a vital role in how proteins operate, and understanding them could unlock new pathways in medical research.
Bridging the Data Gap
One of the significant hurdles faced by scientists in this realm has been the limited availability of kinetic data that detail how proteins transition between conformational states. To counter this issue, the research team ingeniously combined a physically constrained coarse-grained molecular dynamics model with enhanced sampling methods, crafting a robust computational framework optimized for simulating protein allostery.
Simulation Success
In their ambitious endeavor, the researchers successfully simulated the conformational changes of an impressive 2,635 proteins, each transitioning between two known stable states. This comprehensive simulation effort has yielded the first large-scale database of protein dynamics, offering a treasure trove of structural information that can enhance our understanding of protein movement and functionality.
Introducing PATHpre
From this rich database, the team developed a versatile deep learning model called PATHpre, specifically engineered to predict allosteric pathways between stable protein states. Remarkably, PATHpre demonstrated its reliability across a diverse array of proteins ranging from 44 to 704 amino acids in length, showcasing its adaptability to various protein systems, including morphogenic proteins crucial for cellular growth.
Validation of Predictive Capabilities
Validation of the model's predictive capabilities was conducted through comparisons against experimental data from several protein systems, resulting in consistently accurate outcomes. Notably, the researchers unveiled a previously unknown allosteric regulation mechanism in human β-cardiac myosin—an essential protein for heart function—highlighting the model's potential to uncover new biological insights.
Implications for Therapeutic Interventions
The implications of the PATHpre model extend far beyond basic research; it is set to revolutionize our understanding of protein behavior, opening up new avenues for therapeutic interventions aimed at addressing protein misfolding diseases. As researchers continue to explore the intricacies of protein dynamics, we can expect to see exciting developments that could pave the way for innovative treatments for a variety of health conditions.
Conclusion and Future Directions
The groundbreaking research was led by Prof. Wang Qian from the University of Science and Technology of China (USTC) and Prof. Bai Fang from ShanghaiTech University, emphasizing the collaborative spirit driving advancement in this crucial scientific area. Stay tuned, as this development promises to reshape the landscape of protein research and therapeutics!