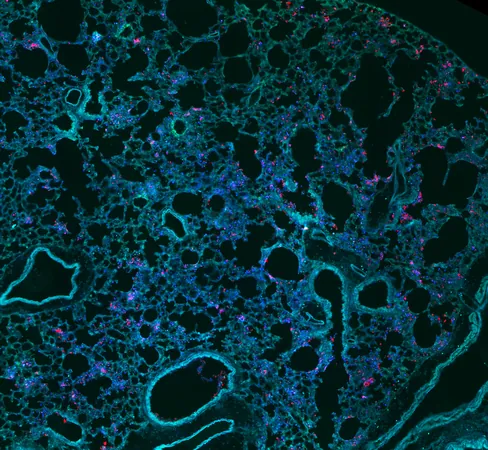
Breakthrough in Pneumonia Prognosis: New Machine Learning Model Predicts Patient Outcomes with Unprecedented Accuracy!
2024-11-06
Author: Mei
Breakthrough in Pneumonia Prognosis
In a groundbreaking study, researchers from Northwestern University have developed a revolutionary machine-learning model that leverages electronic health records (EHRs) to identify five distinct clinical states in pneumonia. This innovative approach allows for more accurate predictions regarding patient outcomes, significantly informing treatment decisions for clinicians facing the challenges presented by pneumonia, a leading global cause of death.
Challenges in Pneumonia Treatment
Pneumonia, characterized by fluid-filled sacs in the lungs leading to severe breathing difficulties, presents a unique set of challenges. Patients can exhibit vastly different symptoms and prognoses, leaving doctors grappling with how to effectively treat their patients. Surprisingly, traditional classification methods—based on the origin of pneumonia (whether community-acquired, hospital-acquired, or ventilator-associated)—often fall short in determining patients' recovery chances, according to Dr. Luís Amaral, the lead author of the study.
Key Findings of the Study
The new model identified three of the five pneumonia states as key indicators relating to disease outcomes, with one state alarmingly associated with a 7.5% likelihood of death within just 24 hours. This critical finding underscores the predictive power of the identified states, helping healthcare providers make crucial end-of-life decisions with better-informed insights.
Research Methodology
The research, published in the esteemed journal *Proceedings of the National Academy of Sciences*, utilized an advanced suite of machine-learning tools to analyze a wealth of patient data—from body temperature and breathing rate to glucose and oxygen levels. By clustering these varying data points, the team could construct distinct clinical states that provide much clearer prognostic information.
Broader Implications
Dr. Amaral, who leads the team at the McCormick School of Engineering and is a professor of medicine, emphasized that this novel classification could also mitigate the risks of over-treatment, guiding family members in understanding potential outcomes of their loved ones’ conditions.
Future Investigations and Adaptability
The implications of this research extend beyond pneumonia; the algorithms created may be adapted for other medical conditions, including septic responses in mice, as the team continues to explore their applicability in different contexts. Future investigations are also planned to further unravel why some patients transition between states, aiming to refine treatment protocols significantly.
Conclusion
As pneumonia endures as a complex and multifaceted disease, leveraging innovative technology and machine learning may ultimately lead to enhanced survival rates and improved quality of care for millions of patients worldwide. This research represents a clarion call for the integration of advanced analytics in healthcare, paving the way for more personalized and effective treatment strategies.
Stay tuned for the next chapter in pneumonia care—this could change everything!