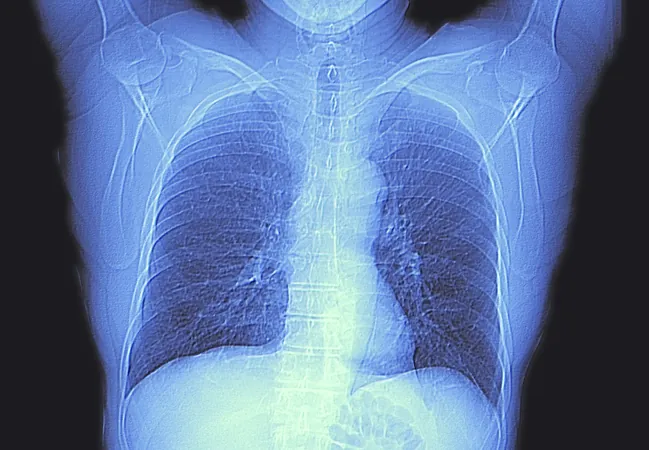
Breakthrough in Lung Cancer Detection: New Deep Learning Model Achieves Remarkable Accuracy in CT Scans!
2025-01-22
Author: Daniel
Groundbreaking Deep Learning Model for Lung Cancer Detection
A groundbreaking deep learning model has shown tremendous promise in the accurate detection and segmentation of lung tumors from CT scans according to a recent study published in *Radiology*, the official journal of the Radiological Society of North America (RSNA). This innovative research could revolutionize lung cancer treatment by enhancing early diagnosis and refining therapy planning processes.
Significance of the Advancement
Dr. Mehr Kashyap, the lead author and a resident physician at Stanford University School of Medicine, emphasized the significance of this advancement. 'Our study represents a pivotal step towards the automation of lung tumor identification and segmentation,' he stated. 'This technology could lead to significant improvements in areas such as automated treatment planning, tumor burden assessment, and treatment response evaluations.'
Training and Evaluation of the Model
Building upon previous applications of artificial intelligence (AI) in lung cancer imaging, this study marks a considerable advancement. The model was trained on an extensive, diverse dataset, making it one of the most sophisticated tools currently available for lung tumor detection.
The research team conducted a retrospective study utilizing data from 1,504 pre-radiation treatment CT scans, which included 1,828 tumor segmentations to train the model. The model's accuracy was subsequently evaluated against 150 CT scans, and its performance metrics were impressively high. The results showcased a sensitivity of 92% and a specificity of 82% in detecting lung tumors, which is a notable leap from traditional AI models that often rely on manual input or smaller datasets.
Model Performance and Comparison
For context, when evaluating 100 CT scans featuring single tumors, the model achieved a Dice Similarity Coefficient (DSC) score of 0.77 for segmentation overlap, closely trailing the physician's overlap score of 0.80. Notably, the model completed segmentation tasks in a fraction of the time it takes human radiologists.
Advanced Technology in Use
A key factor contributing to the model's enhanced performance is its utilization of a 3D U-Net architecture, which allows for comprehensive capturing of interslice information. This technological innovation enables the identification of smaller lesions that 2D models struggle to differentiate from surrounding structures like blood vessels and airways.
Diverse Training Dataset
The success of this model can be attributed to the vast size and diversity of its training dataset, derived from routine scans of patients undergoing radiotherapy across multiple medical facilities. This has equipped the model with a rich understanding of varied tumor types, scanning technologies, and physician segmentation techniques, thereby increasing its adaptability to different clinical contexts.
Challenges Ahead
However, the model does face challenges. While effective for most tumor sizes, it tends to underestimate the volume of very large tumors, a vital piece of information for developing a comprehensive treatment plan. Researchers noted that this discrepancy could stem from a lack of representative data during the model's training phase.
Future Studies and Potential
Looking ahead, further studies are imperative to explore the model's potential for estimating tumor burden and evaluating ongoing treatment responses compared to other models. Additionally, the researchers aim to assess the model’s capability to predict clinical outcomes based on its tumor burden estimates when utilized alongside other prognostic tools and diverse clinical data.
Conclusion
The future of lung cancer detection holds exciting possibilities, and this model may prove to be a key player in transforming patient care and outcomes in oncology. Stay tuned for more updates on this incredible technology’s journey to clinical application!