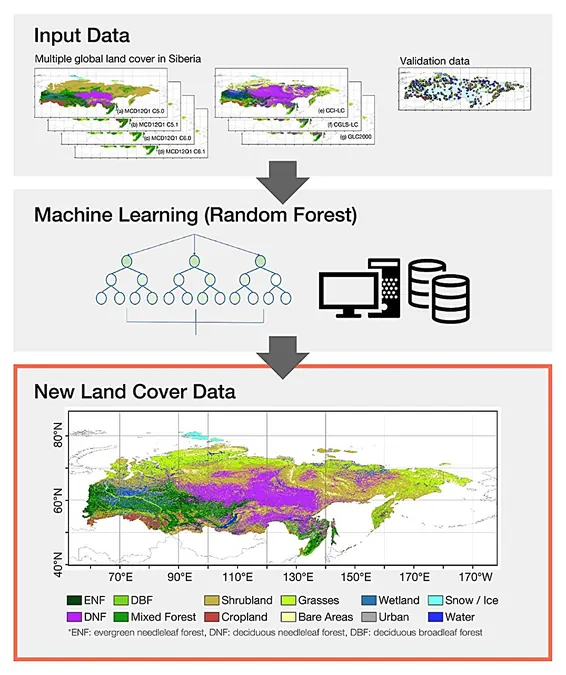
Breakthrough in Land Cover Mapping: How Machine Learning is Transforming Climate Predictions for Siberia
2025-03-25
Author: Jia
Siberia, one of the world's most expansive and crucial ecological regions, is pivotal to our global carbon cycle. With its extensive forests, wetlands, and permafrost, Siberia serves as a significant carbon reservoir. However, climate change is wreaking havoc on this delicate ecosystem, causing shifts in vegetation and accelerating permafrost thaw, which pose serious risks to both local and global environments.
A key aspect of influencing climate predictions is accurately classifying land cover, yet the sparse availability of ground observation data in regions like Siberia has posed significant challenges. A revolutionary study led by Professor Kazuhito Ichii at Chiba University, Japan, has made substantial strides by producing a highly precise land cover map for Siberia, which could dramatically enhance climatic predictions.
The research employs advanced methods, including a random forest classifier—an effective machine learning algorithm—to address inconsistencies found in existing datasets. The results? A remarkable 85.04% accuracy rate in land cover classification, which was previously hampered by outdated or flawed data.
This groundbreaking study, in partnership with Nagoya University and published in "Progress in Earth and Planetary Science," aims to rectify discrepancies observed in leading data sets. As Professor Ichii noted, "During the Pan-Arctic Water-Carbon Cycles project, we identified significant variability in existing datasets. This realization triggered our pursuit of a more reliable dataset."
The researchers meticulously compared various global datasets, ensuring a comprehensive evaluation of land cover that ultimately highlights critical areas of forests, wetlands, and permafrost. These elements are essential for climate analysis. Errors identified in previous datasets, particularly in high-latitude areas, could have led to unreliable climate projections, which this new map addresses effectively.
Munseon Beak, a graduate student involved in the study, emphasized the importance of re-evaluating land cover classifications, stating, "By improving the data quality in an area previously assumed to be well understood, we've opened up new possibilities for climate forecasting."
The implications of this refined dataset are monumental. Accurate land cover data not only improves climate models but also better predicts environmental changes in the region. The urgency is underscored by Assistant Professor Yuhei Yamamoto, who remarks, "Integrating multiple sources and enhancing classification accuracy provides essential insights for researchers, especially as dramatic climatic changes accelerate in Siberia."
Climate change in Siberia is substantially noticeable, with shifts like the northward movement of the Siberian Taiga and landscape alterations caused by thawing permafrost. The newly developed land cover data becomes an essential resource for scientists aiming to monitor and understand these critical changes moving forward.
Additionally, this study identifies crucial factors influencing vegetation distribution. As Professor Tetsuya Hiyama explains, "Geographical distribution analysis across varying climates has highlighted precipitation as a significant determinant of vegetation patterns, particularly during warmer summer months."
As researchers continue to unravel the complexities of Siberian ecosystems through sophisticated data techniques, this advancement not only fills gaps in scientific knowledge but also empowers climate scientists to better manage and predict future climatic scenarios. This study exemplifies the potential of machine learning technology in addressing some of the most pressing environmental challenges of our time.