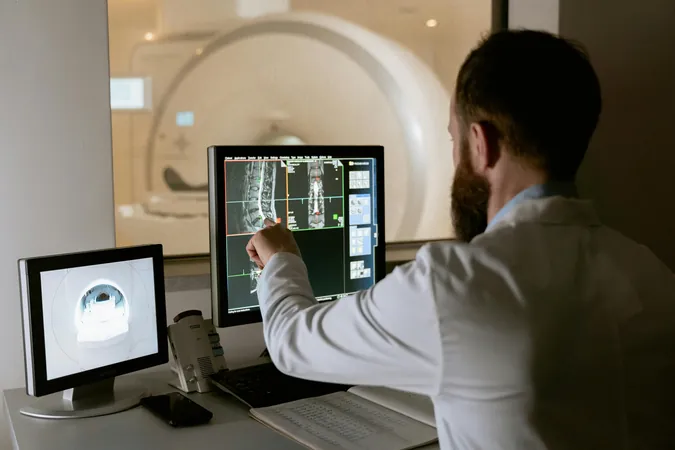
Breakthrough AI Model Matches Clinical Experts in Analyzing Complex Medical Scans – The Future of Diagnostics?
2024-10-01
A team of researchers at UCLA has unveiled an innovative deep-learning framework that is revolutionizing the way medical professionals analyze and diagnose complex 3D medical images, such as MRIs. This cutting-edge model achieves accuracy on par with seasoned medical specialists, but with a staggering reduction in the time required for analysis. The groundbreaking work has been detailed in an article published in the prestigious journal *Nature Biomedical Engineering*.
What sets this model apart from other AI systems is its exceptional adaptability across diverse imaging techniques. The research team has tested it using various modalities, including 3D retinal scans for identifying disease risk markers, ultrasound videos monitoring heart function, 3D MRI scans for assessing liver disease severity, and 3D CT scans for detecting chest nodules. This versatility could enhance diagnostics in numerous clinical settings, with further studies already in the pipeline.
Artificial neural networks, the backbone of the SLIViT model (which stands for SLice Integration by Vision Transformer), learn by processing massive datasets that are meticulously examined and labeled by clinical experts. Traditional 2D imaging offers limited depth perception, while the new 3D imaging techniques provide a more comprehensive view, requiring more time and expertise to interpret accurately. For instance, a single 3D retinal scan may consist of close to 100 individual 2D images, demanding several minutes of focused inspection from a trained specialist to identify subtle disease indicators.
"While numerous AI techniques exist for 2D image analysis, developing and labeling extensive 3D datasets necessary for those models has historically been unrealistic," explained Oren Avram, Ph.D., a postdoctoral researcher and co-first author of the study. "Existing models often focus on a single imaging type or disease, which limits their effectiveness."
The SLIViT model combines unique AI components and learning strategies that empower it to predict disease risk factors from various imaging modalities, even with moderately sized labeled datasets. "By leveraging prior medical knowledge from accessible 2D datasets, SLIViT overcomes the challenge of needing large training samples," stated Berkin Durmus, a UCLA Ph.D. student and co-first author.
Remarkably, SLIViT demonstrates superior performance not only compared to similar AI models but also in relation to manual evaluations by clinical specialists, completing tasks up to 5,000 times faster. Moreover, it has the resilience to handle clinical datasets that may not be well-organized.
Dr. SriniVas R. Sadda, a leading professor at UCLA Health, expressed his excitement about SLIViT's efficiency, particularly with limited training datasets. "This model's ability to function optimally with just a few hundred training samples is a game changer for 3D biomedical imaging."
Despite the thrill of innovation, the research team acknowledges the inherent challenges in medical AI development. As Eran Halperin, Ph.D., points out, even with seemingly unlimited resources, the need for sizable data sets limits progress in many clinical scenarios, especially when identifying new disease-related factors.
However, SLIViT presents a solution, as one trained clinician can annotate a small dataset within days, significantly expediting the analysis of many unannotated volumes and producing results similar to those of medical experts.
The UCLA team has ambitious plans moving forward. They aim to expand their research to encompass additional imaging modalities and to explore SLIViT's potential for predictive disease forecasting, which could greatly facilitate early diagnosis and personalized treatment strategies. Furthermore, they are dedicated to addressing systemic biases within AI models to prevent exacerbating health disparities.
With this remarkable leap in AI technology, the healthcare landscape is poised for a transformation. Are we witnessing the dawn of a new era in medical diagnostics? Only time will tell, but the potential benefits for both clinicians and patients are monumental. Stay tuned for future updates on this groundbreaking research!