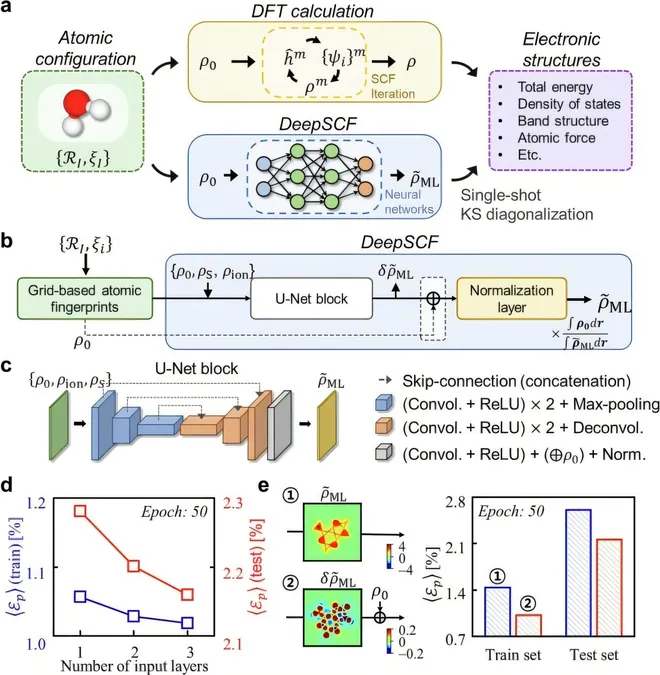
Breakthrough AI Method Revolutionizes Quantum Mechanics Calculations!
2024-10-30
Author: Wei
Breakthrough AI Method Revolutionizes Quantum Mechanics Calculations!
In an extraordinary development blending artificial intelligence with quantum mechanics, researchers from KAIST have devised a revolutionary approach that could dramatically reduce the time required for complex quantum calculations. This method, which foresees atomic-level chemical bond information in three-dimensional space, has the potential to reshape scientific computing in both the physics and chemistry fields.
Hot off the heels of distinguished recognition, with both the 2024 Nobel Prizes in Physics and Chemistry awarded for advancements involving AI, Professor Yong-Hoon Kim and his team at KAIST's School of Electrical Engineering are making headlines once again. Their research introduces a 3D computer vision artificial neural network-based method that eliminates the need for the traditionally complex algorithms required for atomic-level quantum mechanical calculations typically reliant on supercomputers.
The Conventional Challenge of Quantum Calculations
In quantum mechanics, density functional theory (DFT) uses supercomputers to predict material properties by solving intricate quantum equations. However, this computational method requires a lengthy self-consistent field (SCF) process, necessitating multiple iterations—often ranging from tens to hundreds—restricting its practical application to a limited number of atoms. This challenge has hindered advancements in numerous fields, including drug design and the development of advanced materials.
A Game-Changing Solution with DeepSCF
Professor Kim's innovative team turned to artificial intelligence in their pursuit of efficiency. They developed the DeepSCF model, which harnesses a neural network algorithm to bypass the tedious SCF process. By doing so, they accelerated calculations significantly, validating the methodology's potential for both complex and larger systems.
The researchers targeted the electron density—a crucial element in DFT that encapsulates all quantum mechanical information of electrons—along with the residual electron density, revealing vital chemical bonding information. To train their AI model, they utilized a diverse dataset of organic molecules, enhancing model accuracy by exposing it to various rotational and deformational scenarios.
Implications for Science and Industry
The ramifications of this breakthrough are vast. By circumventing lengthy calculation times, the DeepSCF model can facilitate faster research and development processes across a spectrum of applications—from semiconductor design to molecular engineering. Furthermore, the principles established through this research could influence future explorations in artificial intelligence, providing new paradigms for material calculations on multiple scales.
Professor Yong-Hoon Kim stated, "We have discovered a pathway to link quantum chemical bonding information with an artificial neural network. Our work lays the groundwork for accelerating material simulations and exploration in quantum mechanics, ultimately pushing the boundaries of scientific innovation."
This innovative approach, published in the journal npj Computational Materials, signals an exciting future where accuracy in quantum simulations can be achieved at unprecedented speeds, marking a pivotal shift in the intersection of AI and science. Will this be the dawn of a new era in quantum mechanics? Only time will tell!