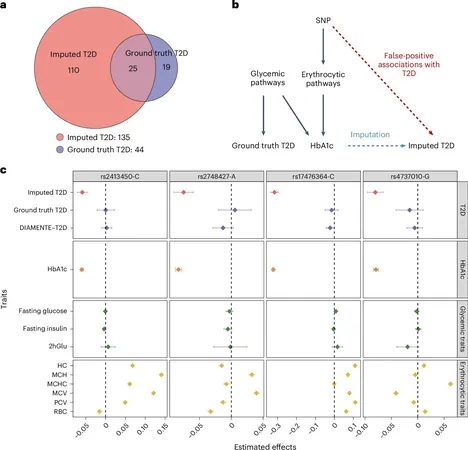
Alarming Flaws in AI-Enabled Genomic Research Could Mislead Medical Science
2024-11-04
Author: Wei Ling
Recent research from the University of Wisconsin–Madison has unveiled troubling flaws in the increasingly popular use of artificial intelligence (AI) within genomic studies. Experts caution that these powerful tools, while enticing, might lead scientists to incorrect conclusions regarding the relationships between genes and physical traits—such as key risk factors for diseases like diabetes.
Complications of Genetic Links in Disease Development
The role of genetics in health is well recognized, with certain gene changes undeniably linked to increased disease risk, such as in cystic fibrosis. However, the interplay between genetic factors and traits can be intricate, presenting a significant challenge for researchers. Projects such as the NIH's All of Us initiative and the UK Biobank provide vast databases of genetic and health data, but unfortunately, vital information related to certain health conditions can often be sparse.
According to Qiongshi Lu, an associate professor in UW–Madison’s Department of Biostatistics and Medical Informatics, "Some characteristics are either prohibitively costly or labor-intensive to measure, which leaves researchers with insufficient data to reach meaningful statistical conclusions."
The Dangers of AI Data Bridging
With these data gaps, many researchers have turned to advanced AI solutions in a bid to compensate for missing health information. "The use of cutting-edge machine learning techniques has surged in recent years," remarks Lu. "Researchers now use these sophisticated models to make predictions about complex traits and disease risks, even when data is limited."
However, a recent study by Lu and his team, published in Nature Genetics, highlights the dangers of this approach. They found that relying solely on machine learning could lead to "false positives," where algorithms misidentify genetic variations linked with diseases such as type 2 diabetes—an issue not confined to this condition alone.
"If research hinges on the machine learning-predicted diabetes risk as the actual risk, it could erroneously suggest strong correlations between multiple genetic variations and diabetes that simply do not exist," Lu explains. This widespread bias poses a significant risk in AI-assisted genomic research.
A New Statistical Solution on the Horizon
In response to these challenges, Lu and his colleagues introduced a novel statistical method designed to enhance the accuracy of AI-assisted genome-wide association studies. Their method effectively addresses the biases that machine learning can introduce, particularly when dealing with incomplete data sets.
“This strategy is statistically optimal,” states Lu, noting its effectiveness in accurately establishing genetic associations with traits such as bone mineral density.
Proxy Data Pitfalls: A Broader Concern
The risks associated with AI are not the only concern in genomic research. The team also uncovered issues related to studies that use proxy data to fill in gaps, which can also lead to erroneous conclusions. For example, while databases such as the UK Biobank contain rich genetic datasets, they often lack comprehensive information on diseases prevalent later in life, such as neurodegenerative diseases.
Researchers have attempted to circumvent this issue by obtaining proxy data through family health history surveys—essentially relying on individuals to report their relatives' health issues, such as an Alzheimer’s diagnosis. Lu's team warned that such proxy studies can produce "highly misleading" genetic correlations between Alzheimer's risk and cognitive abilities.
"As the statistical power of biobank datasets increases, so too does the risk of biases and errors," Lu cautions. "Our recent findings underscore the necessity for meticulous statistical practices in genomic research, particularly in large-scale studies."
Conclusion: A Wake-Up Call for Researchers
The alarming insights from UW–Madison's research serve as a crucial reminder for scientists in genomic studies to tread carefully. As AI technologies continue to evolve and permeate medical research, ensuring accuracy and reliability remains paramount. Failure to address these flaws could not only misguide scientific understanding but also potentially endanger public health outcomes. Researchers are urged to prioritize rigorous statistical analysis, urging caution before placing unwavering trust in AI predictions. This could be the moment that reshapes the future of genomic studies—if the scientific community takes heed.