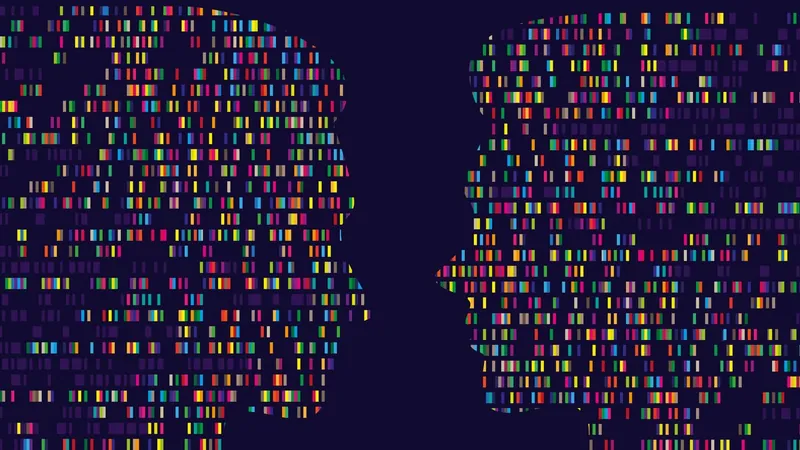
AI Benchmarks Are Falling Short: Is It Time for Human Evaluation?
2025-03-29
Author: Daniel
Artificial intelligence (AI) has made incredible strides in recent years, often triumphing over standardized tests designed to mimic human judgment and understanding. Established benchmarks such as the General Language Understanding Evaluation (GLUE) and the Massive Multitask Language Understanding (MMLU) dataset have previously provided a framework to assess how well AI models perform across a range of knowledge-based tasks.
However, as AI technology advances, these tests are increasingly seen as inadequate. The growing consensus among experts suggests that a new approach, one that incorporates human evaluation, may be necessary to truly gauge the capabilities of generative AI systems.
Michael Gerstenhaber, head of API technologies at Anthropic—the company behind the Claude family of large language models—asserted during a Bloomberg Conference that “we’ve saturated the benchmarks.” This sentiment highlights a widespread realization in the AI community that traditional evaluations may no longer reflect the real-world performance or applicability of these models.
Recent research emphasizes the importance of integrating human assessments into the evaluation process. A study published in The New England Journal of Medicine led by Adam Rodman from Boston's Beth Israel Deaconess Medical Center argues that humans should play a pivotal role in evaluating AI benchmarks, particularly in the medical field. Rodman notes that traditional medical benchmarks like MedQA have become too easy for AI, rendering them ineffective as measures of practical clinical skills.
To improve evaluations, the team advocates for adopting training methods used in medical education, such as role-playing and human-computer interaction. While these methods may take longer than conventional benchmarks, they are expected to become increasingly essential as AI systems evolve.
The role of human oversight in AI development has been a significant factor in recent advancements. Notably, the launch of ChatGPT involved a process known as “reinforcement learning from human feedback,” where human reviewers graded the AI’s outputs, helping to refine its capabilities.
Leading tech companies are now prioritizing human evaluation over traditional automated metrics. Google, for instance, spotlighted human ratings during the introduction of its open-source model, Gemma 3, framing it in competitive terms akin to sports scoring. OpenAI similarly touted human preference measures when presenting its state-of-the-art model, GPT-4.5, suggesting that this model not only performs well on automated tests but also resonates better with human evaluators.
As AI developers continue crafting new benchmarks, human participation is becoming a central theme. Innovations are emerging, like the updated Abstraction and Reasoning Corpus for Artificial General Intelligence (ARC-AGI), introduced by François Chollet from Google’s AI team. A recent study in San Diego engaged over 400 participants to calibrate the difficulty of tasks featured in ARC-AGI 2, highlighting the role of human cognition in establishing performance standards.
This blending of automated evaluations with significant human input signifies vast potential for enhancing the development, training, and testing of AI models. As AI technologies edge closer to achieving human-like reasoning, the dialogue around the necessity and methodology of human evaluations in AI is more vital than ever.
While the journey to artificial general intelligence continues, one thing is clear: the path forward will likely involve the deliberate integration of human insights alongside AI capabilities, fostering a more holistic understanding of the technology's impact on our lives.