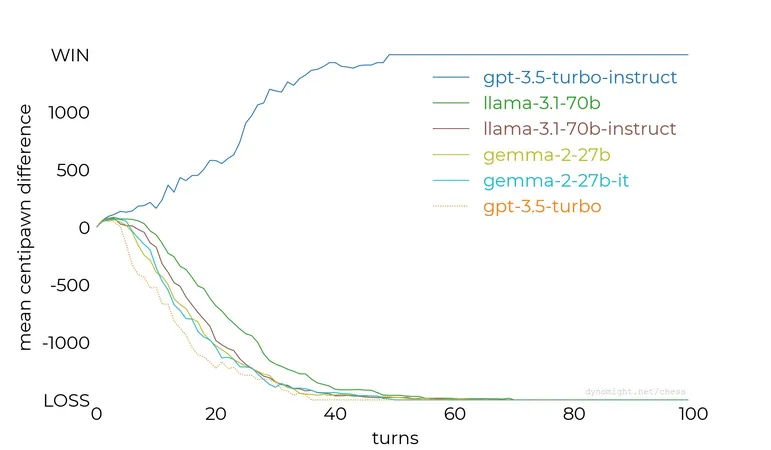
Unraveling the Surprising Chess Skills of LLMs: Are They the Future of Strategy?
2024-11-16
Author: Ming
Unraveling the Surprising Chess Skills of LLMs
At first glance, the idea of challenging a large language model (LLM) to a game of chess might sound ludicrous. After all, these AI systems are primarily designed for language processing and have not been explicitly programmed to understand the intricacies of chess, such as board states, strategies, or the movements of pieces like rooks and knights. However, a recent exploration by chess enthusiast Dynomight, shared in a blog post, reveals an unexpected twist in this narrative.
LLMs vs. Stockfish
Testing various LLMs against the formidable Stockfish chess engine, ranging from the smaller Llama model to the more advanced GPT-3.5, the results largely aligned with expectations. Yet, there was a remarkable revelation: the gpt-3.5-turbo-instruct model seemed to hold its ground surprisingly well against Stockfish, even when the latter was set to a lower difficulty.
The Experiment
In the experiment, each model received a uniform prompt instructing it to behave like a chess grandmaster, utilize standard chess notation, and select their next move. What stood out was the stark contrast in performance between the instruct model and the other variants. Delving deeper, it becomes apparent that OpenAI has crafted the instruct model with a purpose, defining it as an 'InstructGPT 3.5 class model'. A related 2022 paper from OpenAI elaborates on how InstructGPT differs from its standard GPT counterpart by being extensively fine-tuned using human feedback.
Role of Human Feedback
This meticulous tuning seems to allow instruct models to excel in instruction-based queries — a crucial trait when it comes to chess. For context, discussions around the differences between the Turbo and Instruct versions of GPT-3.5 have also surfaced on platforms like Hacker News, often using chess as a benchmark for comparing capabilities.
ChatGPT and RLHF
ChatGPT, identified as a sibling to InstructGPT, utilizes a mechanism called Reinforcement Learning from Human Feedback (RLHF), hinting at a structure where user feedback continually refines its performance. Despite OpenAI’s repeated caveats — that neither InstructGPT nor ChatGPT can guarantee accurate responses at all times — the limited scope of chess presents a unique testing ground where these models demonstrate enough competence to avoid an embarrassing defeat by a dedicated chess AI.
Implications for the Future
As artificial intelligence continues to evolve, the potential for LLMs to disrupt traditional fields like chess becomes more intriguing. Could these models, trained primarily for language, adapt and refine their strategic thinking in games of skill? Their unexpected proficiency raises questions about the future evolution of AI in complex domains. With ongoing advancements, the line between human and machine intelligence may blur even more, leaving enthusiasts and skeptics alike to ponder the exciting possibilities that lie ahead. Will we be witnessing a new era of chess champions forged from the code of instruct models? Only time will tell!