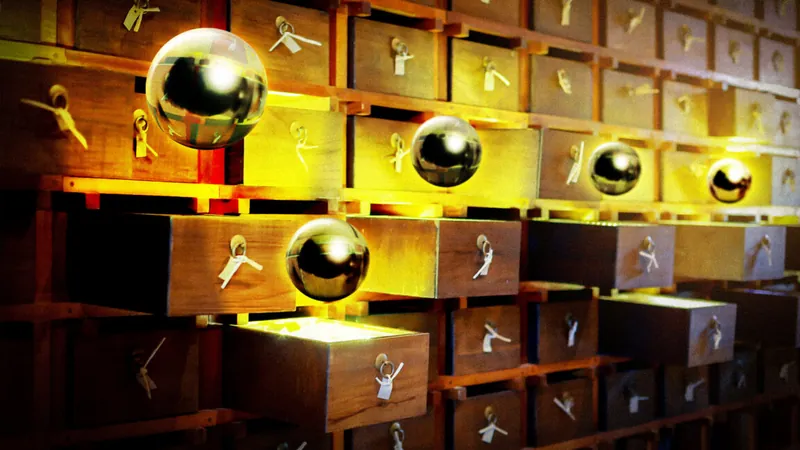
The Unlikely Architect of the Deep Learning Revolution: How One Scientist's Vision Ignited a Tech Phenomenon
2024-11-11
Author: Chun
Introduction
In the fall of 2008, the world of artificial intelligence seemed stale, and neural networks had been largely disregarded by many scholars. During my first semester as a computer science graduate student at Princeton, I attended a lecture on AI that left me with the impression that neural networks were a forgotten relic. Indeed, despite their promising results in the late 1980s and early 1990s, research had dwindled in favor of more mathematically appealing approaches, such as support vector machines.
The Visionary Project: ImageNet
However, unbeknownst to me, a groundbreaking project was underway in the very same computer science building. Led by the visionary Professor Fei-Fei Li, this initiative sought to create ImageNet, an unprecedented dataset comprising 14 million images across nearly 22,000 categories. Li was not motivated by a desire to enhance neural networks; rather, she aimed to challenge the status quo and demonstrate the potential of deep learning through expansive datasets.
Li faced considerable skepticism from peers during her two-year journey to develop ImageNet. One mentor cautioned her that she was overextending, stating, “The trick is to grow with your field, not leap ahead of it.” Despite the doubts, Li pressed on, believing that the size and diversity of the dataset would ultimately prove beneficial to machine learning algorithms, which at the time thrived on only limited data.
ImageNet's Impact
By the time ImageNet was publicly released in 2009, its impact remained largely unnoticed. It wasn’t until 2012, however, that this ambitious endeavor bore fruit. A team at the University of Toronto, utilizing the dataset, trained a neural network named AlexNet, which not only achieved record-breaking accuracy in image recognition but also heralded the emergence of the deep learning boom.
Key Figures in the Revolution
Central to this revolution, aside from Li's audacious vision, were two other key figures: Geoffrey Hinton and Nvidia's CEO Jensen Huang. Hinton had long been a proponent of neural networks, tirelessly advocating for their relevance in machine learning despite encountering skepticism for decades. On the other hand, Huang recognized early on the potential applicability of graphics processing units (GPUs) for general computing tasks, leading to the creation of CUDA—a platform that turned GPUs into powerful tools for tasks beyond gaming.
Notably, applicants like Hinton’s team, including Alex Krizhevsky and Ilya Sutskever, used Nvidia’s CUDA to process and train models at astonishing speeds, far surpassing traditional CPUs. This synergy of a robust dataset, innovative algorithms, and advanced hardware set the stage for unparalleled advancements in AI.
The 2012 ImageNet Competition
The real turning point came during the annual ImageNet competition in 2012 when Hinton's deep neural network outperformed all previous entrants with a staggering top-5 accuracy of 85%. This event not only reinstated belief in neural networks but also emphasized the importance of large datasets—creating a loud wake-up call in the tech community.
Revolutionizing AI Research
Fei-Fei Li’s creation of ImageNet proved to be more than just a dataset; it revolutionized AI research, leading to neural networks being recognized as a valid approach to machine learning once again. Coinciding with advancements in computing power, these developments unleashed innovations across multiple industries, from autonomous vehicles and facial recognition to biomedical research.
A Lesson in Visionary Thinking
Not simply a tale of success, this narrative offers a crucial lesson in the importance of visionary thinking and audacious risk-taking. As we look toward the future, it’s pivotal that today's technological leaders remember the value of challenging conventional wisdom. With AI evolving rapidly, we may soon see another group of nonconformists who will challenge norms once again—and propel us into the next wave of innovation.
Conclusion
Sometimes, progress comes from being stubbornly insistent on ideas that initially seem far-fetched. Following the trajectory established by these pioneers, it is likely that the next paradigm of AI could emerge from propositions we are yet to consider plausible. Stay vigilant; the future of AI could depend on the ideas that have not yet made the headlines!