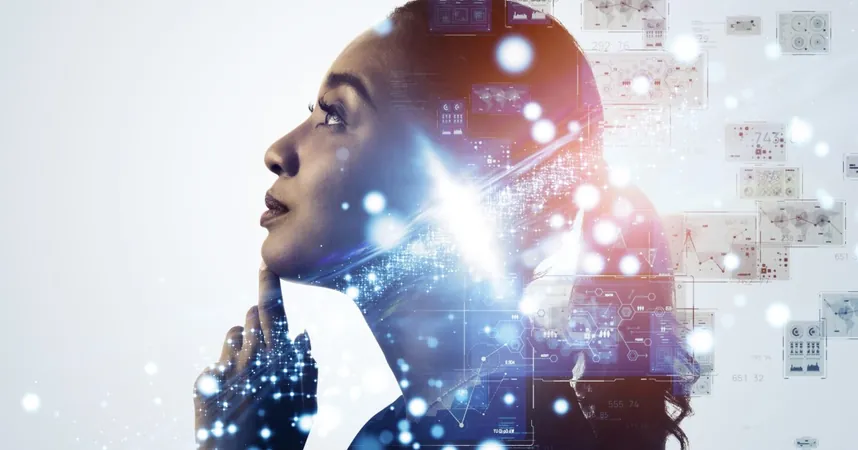
Shocking New Research Reveals AI's Struggles with Historical Knowledge!
2025-01-19
Author: Jessica Wong
Groundbreaking Study on AI and Historical Knowledge
In a groundbreaking study published recently, researchers have discovered that artificial intelligence (AI), despite its impressive capabilities in areas like coding and media generation, falls drastically short when it comes to mastering history. The study reveals that leading large language models (LLMs) such as OpenAI's GPT-4, Meta's Llama, and Google's Gemini fail to meet even basic historical standards on high-level exams.
Hist-LLM: A Unique Benchmark
The research team developed a unique benchmark called Hist-LLM, specifically designed to test these advanced AI models on historical questions. This benchmark draws from the Seshat Global History Databank, an expansive repository of historical data named after the ancient Egyptian goddess of wisdom, Seshat. The results, unveiled at the prestigious NeurIPS AI conference last month, highlighted a staggering reality: the most accurate model, GPT-4 Turbo, achieved only about 46% correctness, comparable to random guessing!
Key Findings from the Research Team
Dr. Maria del Rio-Chanona, an associate professor of computer science at University College London and one of the paper’s co-authors, emphasized the key findings. "While these LLMs showcase remarkable capabilities, they fundamentally lack the deep understanding necessary for tackling complex historical inquiries. They may handle basic facts well, but for PhD-level questions, they still fall short," she stated.
Illustrative Errors and Examples
Illustrative examples shared with TechCrunch reveal significant errors made by these models. For instance, when asked if scale armor existed in ancient Egypt during a particular era, GPT-4 Turbo incorrectly affirmed it, despite the fact that this technology emerged 1,500 years later. Such inaccuracies raise questions: Why can LLMs adeptly handle intricate topics like programming yet stumble over historical details?
Underlying Issues: Data Reliance and Bias
Del Rio-Chanona posits that LLMs often rely on widely available data, leading them to overlook less prominent historical facts. For example, when questioned about the existence of a professional standing army in ancient Egypt during a certain timeframe, GPT-4 incorrectly stated that there was one. This misstep may stem from the abundance of information about armies in other civilizations, such as Persia, overshadowing the specifics of Egyptian history.
Performance Disparities and Inclusivity Concerns
The research also uncovered trends indicating that OpenAI and Llama models significantly underperformed regarding certain regions, particularly sub-Saharan Africa. This suggests inherent biases within their training datasets, raising concerns about the inclusivity of historical knowledge.
Optimism for the Future
Despite these disappointing results, study leader Peter Turchin remains optimistic. He asserts that while LLMs are not yet a viable substitute for human historians, there is still great potential for these systems to assist in future historical research. The research team is actively refining their benchmark by integrating more data from underrepresented regions and crafting more sophisticated inquiries.
Conclusion and Future Directions
"In summary, our findings draw attention to the crucial areas where LLMs need improvement while also highlighting their potential as valuable tools for historians," the paper articulates, marking a pivotal moment in understanding AI's role in academia.
As AI continues to evolve, the implications of this research could reshape how we integrate technology into the study of history, leading us to ponder: will future versions of these models finally bridge the gap? Stay tuned as the journey unfolds!