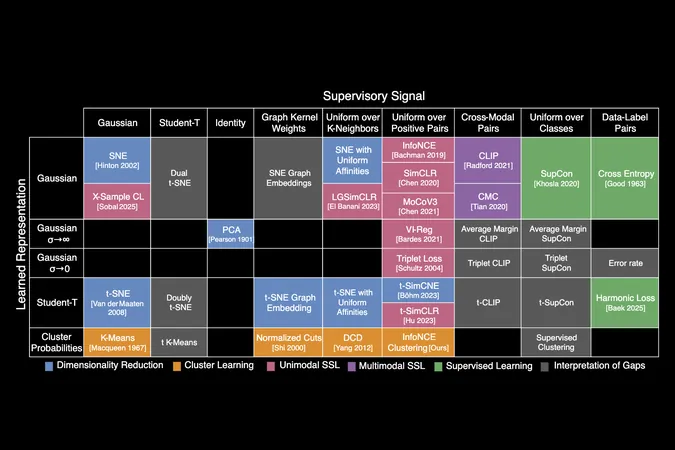
Revolutionizing AI: The Periodic Table of Machine Learning
2025-04-23
Author: Jessica Wong
MIT Unveils Groundbreaking Framework for AI Algorithms
In an innovative breakthrough, MIT researchers have developed a new periodic table that highlights the interconnections among over 20 classical machine-learning algorithms. This pioneering framework could be a game changer in the field of Artificial Intelligence, offering scientists a fresh perspective on combining strategies from various methods to enhance existing AI models or create entirely new ones.
For example, leveraging their new framework, the team successfully merged elements from distinct algorithms to craft a novel image-classification method that outperformed leading approaches by 8%.
A Unified Approach to Machine Learning
At the core of this study lies a unifying principle: all these algorithms share a focused relationship with data points. Even though they each tackle this connection in different manners, the fundamental mathematics driving them is strikingly similar. By identifying a common equation that underpins numerous classical AI algorithms, the researchers have redefined popular techniques and organized them into a comprehensive table based on the relationships they learn.
Much like the original periodic table of elements—once featuring empty spaces that scientists later filled with discoveries—this new periodic table of machine learning also has gaps. These voids represent potential algorithms yet to be uncovered.
According to Shaden Alshammari, the lead author and MIT graduate student, this table serves as a valuable toolkit, enabling researchers to innovate new algorithms without having to retrace old ideas. “It’s not merely a metaphor,” Alshammari asserts. “We’re reshaping the way we perceive machine learning as a structured system ripe for exploration.”
From Accidental Discovery to Groundbreaking Framework
Interestingly, the researchers did not initially set out to create this periodic table. Alshammari, while working in the Freeman Lab, started examining clustering—a machine-learning technique categorizing images according to their similarities. It was during this exploration that she discovered a remarkable similarity between the clustering algorithm and another called contrastive learning by delving into their underlying mathematics. The realization that these seemingly unrelated methods could be explained by a single equation was both unexpected and revolutionary.
Mark Hamilton, a senior author and MIT graduate, remarked, “We almost stumbled upon this unifying equation. Once it connected two methods, we began brainstorming new methodologies to integrate into this framework, and nearly every approach we tried fit in beautifully.”
A New Dawn for Algorithm Discovery
The newly coined framework—Information Contrastive Learning (I-Con)—provides a lens to view various algorithms in relation to this unifying equation, from spam detection to the advanced deep learning systems propelling modern AI.
As the researchers structured their periodic table, they uncovered gaps where uncreated algorithms could potentially exist. By integrating techniques from contrastive learning into image clustering, they developed a new algorithm that significantly boosted classification performance.
The dynamic nature of the periodic table allows for the integration of new connections and columns, fostering a collaborative environment for explorers in machine learning to think beyond their conventional boundaries.
A Catalyst for Innovation in Machine Learning
Hamilton explains that their framework not only illustrates how a single elegant equation—a product of information science—unravels a rich tapestry of algorithms spanning over a century of machine learning research, but also opens diverse pathways for future discoveries.
Yair Weiss, a professor not involved in the study, emphasizes the significance of such unifying approaches amidst the staggering volume of new papers flooding the field. “I-Con exemplifies a rare unifying method and could hopefully inspire similar frameworks across various realms in machine learning.”
Ultimately, this remarkable research effort was supported in part by entities including the Air Force Artificial Intelligence Accelerator and the National Science Foundation. With the insights gained, the future of AI innovation seems brighter than ever.