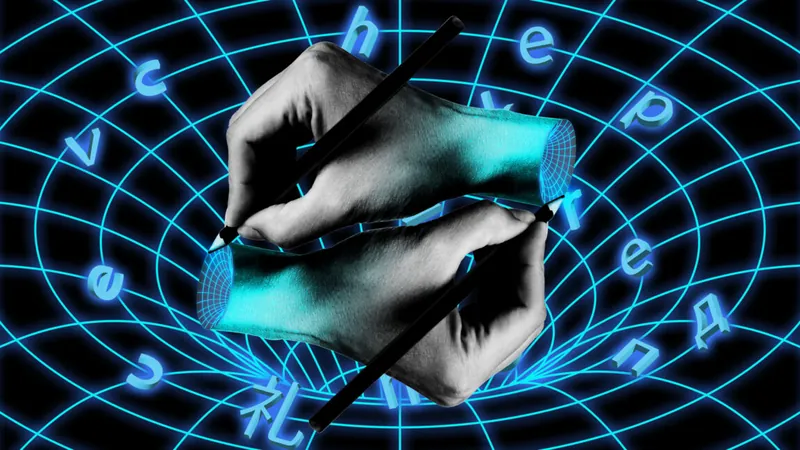
Gemini Hackers Unleash New Attacks with AI-Generated Prompt Injections – Are We Safe?
2025-03-28
Author: Ling
In a startling revelation within the field of AI security, researchers have unveiled a method that allows hackers to employ more sophisticated attacks on major large language models (LLMs) like OpenAI's GPT-3 and GPT-4 or Google's latest offering, Gemini. This new approach, known as indirect prompt injection, takes advantage of the models' inherent inability to differentiate between prompts defined by developers and the text they interact with from external sources.
Prompt injection attacks, while notably powerful, have historically faced a significant barrier. The underlying architecture of so-called closed-weights models—like GPT and Google’s Gemini—is shielded from the public eye, making it difficult for potential attackers to devise working prompt injection methods without extensive trial-and-error. Access to the models' inner workings is tightly controlled, leading to an arduous path of redundancy and resource consumption for those seeking to infiltrate these sophisticated systems.
A Breakthrough with Fun-Tuning
In a significant shift, a team of academic researchers has developed a groundbreaking technique called "Fun-Tuning," which leverages algorithmic generation to create effective prompt injections against Gemini. This innovative method uses the fine-tuning APIs available for Gemini, facilitating training on extensive datasets such as legal documents or medical records—all offered for free by Google.
What sets Fun-Tuning apart is its ability to systematically generate prompt injections with much higher success rates compared to manual ones. By employing discrete optimization, the technique evaluates numerous combinations to identify the most effective prompt injections, potentially changing the game for cyber attackers.
Harnessing the Power of Optimization
Fun-Tuning requires approximately 60 hours of computational work to create optimized injections—at a minimal cost of around $10, given that the fine-tuning API is free. This allows attackers to input multiple prompt injections and wait for the optimized output. Researchers have demonstrated that the success rate of attacks generated by Fun-Tuning could be as high as 82% against certain versions of Gemini, compared to a mere 28% for traditional methods.
During these experiments, researchers highlighted how adding seemingly random prefixes and suffixes to a prompt injection increased its effectiveness significantly. This process of appending gibberish-like tokens, which make up the underlying language model's vocabulary, creates an instruction that LLMs are more likely to comply with, thereby bypassing their safeguards.
Insights on Model Stability and Vulnerability
Fun-Tuning also sheds light on how fine-tuning impacts model stability, presenting opportunities for malicious actors to exploit weaknesses. By analyzing error rates, attackers can devise strategies to increase the likelihood of a prompt injection succeeding. Because fine-tuning maintains an inherent feedback loop through loss values—like a score that guides adjustments—attackers can leverage these insights for their benefit.
The implications are vast, as successful attacks appear to transfer well across different models within the Gemini family. Research shows that if an attack works on one model, it likely will on others within the same lineup, multiplying the potential threats exponentially.
Industry Response and Ceaseless Challenges
Google has yet to formally comment on this new attack strategy, but it is clear that the challenges posed by Fun-Tuning are non-trivial. The company's existing security measures include robust defenses against prompt injection attacks, alongside ongoing assessments meant to fortify the integrity of their LLMs. However, the very systems aimed at enhancing model performance are also providing attackers with the foothold they need.
In the face of such vulnerabilities, researchers have called for an urgent dialogue on balancing operational utility and security measures for fine-tuning technologies. Behind the veil of innovation lies a pressing need for robust solutions to safeguard against these evolving threats—echoing the concerns surrounding AI applications in various industries, from healthcare to finance, where data integrity and privacy are paramount.
The Future of AI Security
While advancements like Fun-Tuning highlight the formidable capabilities of artificial intelligence, they also serve as a reminder that no system is infallible. As the lines blur between AI assistance and malicious intent, it becomes crucial for developers, researchers, and security experts to collaborate in crafting defensive strategies that can outpace these new attack vectors. The question remains: how prepared are we to face the next wave of cyber threats in an increasingly AI-driven landscape?