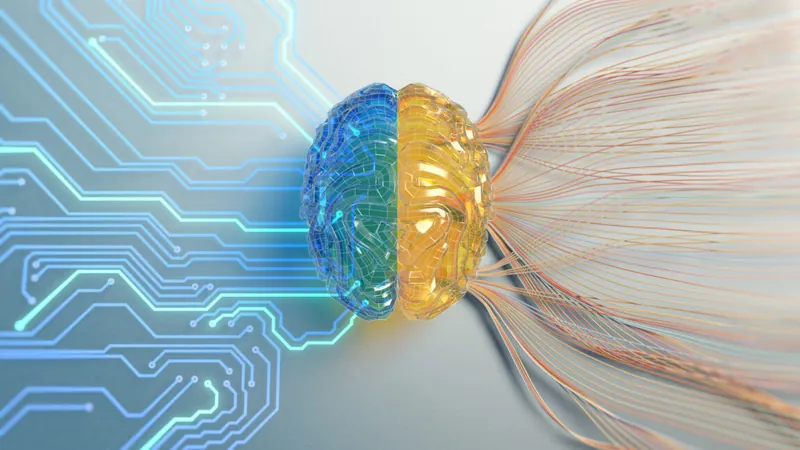
Are Large Language Models Ready to Think Outside the Box? New Findings on Non-Verbal Reasoning!
2024-12-12
Author: Yan
Are Large Language Models Ready to Think Outside the Box? New Findings on Non-Verbal Reasoning!
In recent times, Large Language Models (LLMs) have dominated the AI landscape, exhibiting unparalleled proficiency in language predictions through their transformer architecture. However, when faced with intricate reasoning tasks demanding abstract logical skills, researchers have noted significant limitations. The conventional method of approaching problems solely through “language space” seems to falter, leading to errors even in advanced reasoning models.
To combat these issues, a pioneering group of researchers from Meta’s Fundamental AI Research team (FAIR) and UC San Diego are exploring innovative approaches. They propose new models operating in "latent space," which refers to a hidden computational layer just before the LLM churns out human-readable language. This evolution in model training doesn't entirely overhaul the reasoning potential of LLMs, but preliminary findings suggest marked improvements in accuracy for specific logical challenges and open up exciting avenues for further research.
Understanding Latent Space: What Is It?
The current reasoning approach employed by models like ChatGPT entails the creation of a "chain of thought." Each logical step is framed as a sequence of natural language tokens, which intricately weave through the model. The researchers, however, identified this dependency on natural language as a "fundamental constraint." Successful reasoning often necessitates in-depth planning concerning certain critical tokens to navigate the correct logical trajectory among numerous possibilities.
Traditional chain-of-thought models tend to generate tokens purely for "textual coherence," which, according to researchers, adds little value to the actual reasoning process. They argue for an "ideal" scenario where LLMs can reason independently of language constraints and only convey their conclusions in comprehensible language when necessary.
Their research unveils a groundbreaking methodology called “Training Large Language Models to Reason in a Continuous Latent Space.” In the innovative COCONUT model (Chain Of CONtinUous Thought), the hidden states are encoded as "latent thoughts," effectively replacing conventional written logical steps during both training and query processing. This novel approach allows reasoning to unfurl outside the confines of language, leading to a streamlined logical flow termed as “continuous thought.”
Striving for Efficiency: The Power of Multi-Path Reasoning
The exploration of logical processing in latent space proves advantageous for model efficacy. Perhaps the most significant breakthrough is the model's capacity to “encode multiple potential next steps simultaneously.” Rather than pursuing logical options sequentially—much like a greedy algorithm—operating within latent space fosters a form of immediate backtracking, akin to the breadth-first search through a network.
Remarkably, this simultaneous processing capability manifests in testing, despite the model not being expressly trained for it. The research indicates that while the model might not arrive at the right conclusion right away, it can hold onto various possibilities within its continuous thoughts, progressively discarding incorrect paths in response to implicit value functions.
Interestingly, this multi-path reasoning capability did not markedly enhance COCONUT's accuracy in straightforward mathematical tests (like GSM8K) or general assessments (like ProntoQA). However, it excelled in more elaborate ProntoQA-style queries characterized by complex logical conditions—think along the lines of “every apple is a fruit, every fruit is food.”
In these situations, conventional chain-of-thought models often find themselves in logical dead-ends or even fabricating entirely fictional rules while attempting to resolve intricate chains of inference. Prior studies have also indicated that the explicitly verbalized logical steps from these models may leverage distinct latent reasoning processes than the intended ones.
A Glimpse into the Future of AI Reasoning
This fresh research adds to the growing treasure trove of studies delving deep into the inner workings of large language models at the neural network level. While the field may not yet have produced a monumental breakthrough, the researchers suggest that equipping models with "continuous thoughts" from the outset could lead to enhanced generalization across a broader spectrum of reasoning scenarios.
As the world watches closely, LLMs might soon morph from mere wordsmiths into sophisticated reasoning entities capable of thought beyond the limitations of language. The implications of such advancements could be profound—transforming sectors from education to healthcare, potentially revolutionizing the way we interact with technology. Stay tuned for thrilling updates as this groundbreaking research unfolds!