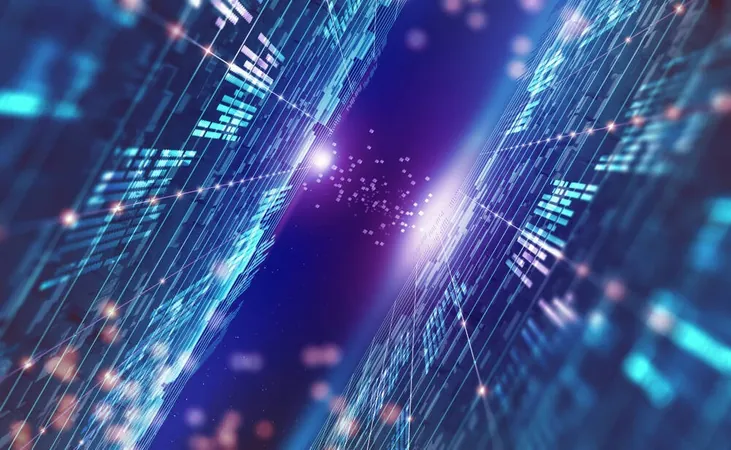
Vector Search: The Game-Changer for Enterprise Databases
2025-04-24
Author: Michael
The Rise of Vector Search in Databases
In a major shift over the past two years, vector search has emerged as a critical feature for enterprise databases, spurred by the explosion of generative AI. Leading cache database Redis was among the first to incorporate this capability, allowing systems to store, index, and query millions of vectorized pieces of information drawn from foundational models like OpenAI's ChatGPT.
A Flood of Competitors in the Vector Search Arena
Since 2023, a multitude of database systems such as MongoDB, Cassandra, PostgreSQL, Snowflake, and SingleStore have announced their own vector search functionalities, saturating the field. In efforts to stand out, these vendors are racing to add unique features that deepen their appeal within today's AI-centric technological landscape.
Innovations in Vector Search from Redis and Yugabyte
Redis has taken strides with its new service, LangCache, designed to cut down on expensive and slow calls to large language models (LLMs). CEO Rowan Trollope explained that this middle layer between applications and LLMs can speed up responses by caching answers to similar queries.
On another front, YugabyteDB is working to enhance its PostgreSQL-compatible platform to better support AI through vector indexing libraries like Usearch and Faiss. Their founders note that these improvements aim to maximize the efficiency of the pgvector extension, keeping pace with the demands of specialized vector databases.
The Challenges of Adopting AI in Business
Gartner's recent analyses reveal a daunting reality: many ambitious AI projects face high failure rates due to unprepared data infrastructures. By 2025, it's projected that 60% of AI initiatives could be abandoned if organizations do not create AI-ready data practices.
Roxane Edjlali from Gartner stresses the importance of implementing vector data stores to enhance data management solutions, which is crucial for leveraging AI effectively. Continuous investment in metadata management, data observability, and AI governance is essential as business data needs evolve.
The Competition: How Other Major Players Are Adapting
As western competitors like Oracle lead the charge with natural language vector search in relational databases, analytics giants like Teradata are also pivoting to accommodate GenAI needs. Teradata has integrated Nvidia's AI tools to bolster their cloud platform Vantage, indicating a serious commitment to meeting the burgeoning demand for AI-driven insights.
Martin Willcox from Teradata emphasizes the increasing demand for sophisticated analytics as businesses explore AI-driven customer interactions. He highlights the need for a vector database that not only scales but also possesses the enterprise-ready qualities that traditional systems require.
SingleStore's Unique Offerings
SingleStore, previously MemSQL, has managed to support vector search capabilities since 2017, focusing on both Online Transaction Processing (OLTP) and Online Analytical Processing (OLAP). They are refining their indexed approximate-nearest-neighbor search to yield faster results and facilitate developer ease in crafting applications.
Their technical evangelist, Akmal Chaudhri, mentions that around 45 of their 400 global customers are leveraging these vector functionalities, particularly in regulated industries looking to create tailored chatbots.
Final Thoughts on the Future of AI-Ready Data
It's clear that as organizations grapple with massive data stores, vector search can provide the means to interrogate and glean valuable insights from this information. Yet, according to Gartner, a significant 63% of organizations lack effective data management practices to fully embrace AI capabilities.
While the excitement surrounding generative AI is palpable, it's essential to remember that not all machine learning solutions fit every situation. Companies must navigate this landscape thoughtfully to optimize their data strategies and realize the full potential of AI.