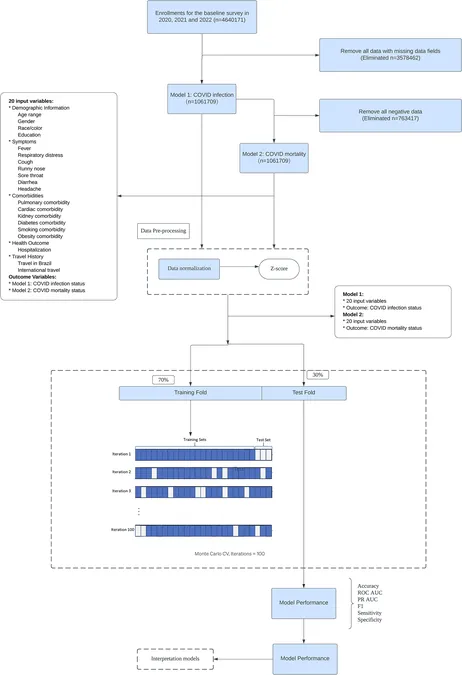
The Hidden Impact of Time: How Variants Shifted COVID-19 Predictions using Shapley Values
2025-04-24
Author: Jacob
A Public Health Crisis Reshaped by Time
As the World Health Organization (WHO) proclaims the end of the COVID-19 public health emergency, the global community grapples with the irrevocable scars left in its wake. With over 773 million confirmed cases and millions of lives lost, the statistics reveal an ongoing struggle against a relentless virus.
Variants and Their Evolving Risks
Throughout its course, COVID-19 has unleashed various variants, each with unique characteristics. The first significant threat, the Alpha variant, emerged in September 2020 from the UK, leading to increased fever rates and heightened vulnerability among older individuals. Following closely, the Delta variant, identified in late 2020 in India, brought forth respiratory challenges and alarming hospitalization rates. Eventually, the Omicron variant surfaced in November 2021, characterized by a rapid spread despite milder symptoms, posing new challenges to public health.
Modeling the Future: The Role of Machine Learning
In response to the pandemic, an array of predictive models has sprung up, utilizing machine learning to forecast infection rates and mortality outcomes. These models, from artificial neural networks to spatio-temporal analyses, have shown promise but also reveal significant limitations, particularly in accuracy across different time periods and variants.
The Puzzle of Model Performance
Research indicates discrepancies in prediction accuracy related to demographic factors, such as age and pre-existing conditions like diabetes and obesity. Some studies suggest unexpectedly high infection and mortality rates in younger populations, challenging previous assumptions about vulnerability. Critically, the temporal aspect—the effects of new variants over time—remains largely unexamined in existing models.
Shapley Values: A New Perspective on Predictions
This investigation aims to explore how changes over time influence the predictive power of COVID-19 models through an innovative lens: Shapley values. By quantifying the impact of individual features in models assessing infection versus mortality, we can shed light on the underlying factors affecting accuracy across different pandemic stages.
Diving Into Data: A Three-Year Analysis
Using a comprehensive dataset from Brazil spanning 2019 to 2022, our study evaluated over one million COVID-19 records, focusing on individual features like clinical symptoms and demographic information. The findings reveal distinct shifts in feature significance over time—factors such as cough and sore throat gained importance in 2022, while fever became less critical. This indicates that the virus's genetic evolution directly influences symptomatology and, consequently, the models built to predict outcomes.
Unraveling Mortality Predictions
In parallel, mortality models demonstrated remarkable stability across years, consistently identifying key predictors such as age and hospitalization status. The comparably high accuracy of these models underscores the effectiveness of using a fixed feature set over time, as opposed to the fluctuating contributions observed in infection models.
Caution in Interpretation: What This Means for Future Predictions
From our findings, a critical takeaway emerges: the temporal gap between training and testing data can skew the accuracy of infection predictions, while mortality predictions remain robust, reflecting a different relationship between qualitative features and COVID-19 outcomes. Shapley values serve as an invaluable tool for gaining deeper insights into feature significance, offering a clearer roadmap for future model development.
Conclusion: Predictive Models in a Post-Pandemic World
As we adapt to a world reshaped by COVID-19, understanding the intricacies of temporal impacts on predictive accuracy will be vital in tackling future health crises. By employing methodologies like Shapley values, we can enhance model transparency and reliability, ensuring effective public health strategies that respond dynamically to emerging threats.