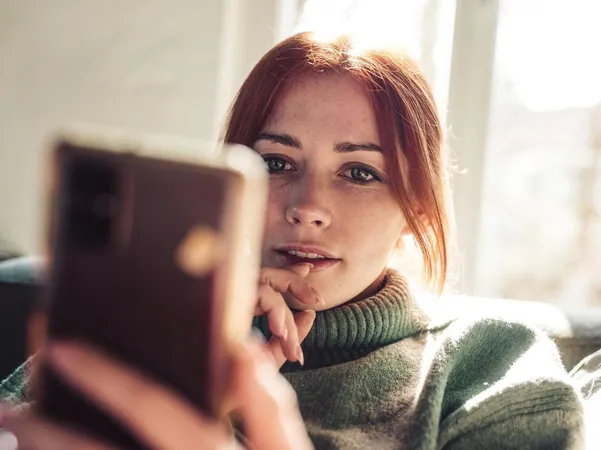
Small Language Models (SLMs) on the Rise: Can They Compete with the Giants of AI?
2024-11-08
Author: Liam
The Rapid Expansion of AI Technology
Generative AI tools like ChatGPT have become household names, integrating LLMs into daily life. These models are powered by extensive datasets pulled from a myriad of human-written texts, allowing them to engage in natural language conversations and perform complex tasks. However, their reliance on large servers and internet connectivity introduces a significant barrier for users in areas with spotty internet service or for those seeking greater privacy and localized solutions.
Imagine having a sophisticated AI assistant fully operational on your smartphone—no internet required! This is where the promise of SLMs comes into play. Designed to function efficiently on limited resources, SLMs present a viable alternative without the need for powerful cloud infrastructures.
Understanding LLMs vs. SLMs: Are They Rivals?
There's a misconception that SLMs and LLMs are in competition. In truth, they complement each other much like various types of vehicles serving different purposes. Larger models can tackle complex queries and are suited for diverse applications, while SLMs shine in specific, high-efficiency contexts, often on personal devices.
Performance vs. Accessibility: A Comparative Analysis
1. **Performance and Efficiency**: LLMs offer a wealth of knowledge and can provide detailed responses, but they require substantial computational power. In contrast, SLMs, while generally less comprehensive, can deliver quick responses straight from your device without the delays caused by internet latency.
2. **Accuracy and Adaptability**: LLMs excel in diverse inquiries, while SLMs thrive when the questions align closely with their training data. They are particularly effective at answering specialized queries, especially if designed with focused training sets.
3. **Cost and Resource Management**: Running LLMs can be cost-prohibitive due to their infrastructure needs, whereas SLMs are more resource-efficient and can operate on devices like smartphones or laptops, which means reduced operational costs.
4. **User Privacy**: LLMs often transmit user data to cloud servers, raising privacy concerns. With SLMs, data can remain on the user’s device, providing an extra layer of security—although users should still verify the privacy policies of specific applications.
5. **Latency and Interaction**: SLMs can offer instant responses, but performance can wane based on the device's capabilities. Conversely, LLMs benefit from high-performance servers, albeit with potential wait times during peak usage.
The Bright Future of SLMs
Recent studies highlight an accelerating interest in SLM research, noting their potential for various applications, particularly in environments demanding efficiency and speed. For instance, an SLM targeting mental health could offer users privacy and instant support—an invaluable feature in today’s society.
With advancements continuously reshaping the fields of AI and machine learning, the lines between LLMs and SLMs are blurring. Innovations are likely to emerge from both realms, making it possible for users to enjoy the benefits of each without having to choose sides.
Conclusion: A Unified Path Ahead
The landscape of AI isn't a battle between SLMs and LLMs but rather a harmonious coexistence that can enhance how we interact with technology. As both models evolve, opportunities will abound for collaboration and innovation.
As famously said by the Dalai Lama, “If you think you are too small to make a difference, try sleeping with a mosquito.” SLMs may be smaller in size, but their impact on the future of AI could be monumental. Stay tuned as we continue to explore the thrilling developments in both SLMs and LLMs—ensuring you stay at the forefront of the AI revolution!