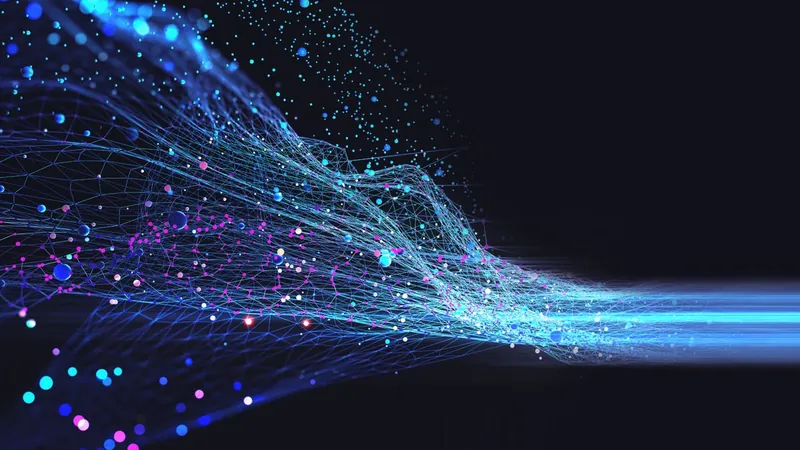
Scientists Sound Alarm: Large Language Models Are Unfit for Real-World Applications!
2024-11-16
Author: Liam
Recent findings raise doubts on large language models' reliability
Recent findings from prestigious institutions including MIT, Harvard, and Cornell have raised serious concerns about the reliability of large language models (LLMs) like GPT-4 and Anthropic's Claude 3 Opus. While these AI systems can produce astonishing outputs in controlled environments, researchers suggest they lack a coherent understanding of real-world dynamics, making them unsuitable for high-stakes applications.
Study reveals flaws in model outputs
In a groundbreaking study published on the arXiv preprint database, the scientists demonstrated that, although LLMs performed nearly flawlessly when given straightforward tasks—such as providing turn-by-turn navigation directions in New York City—the underlying maps they relied upon contained inaccuracies, including streets that do not exist. This discrepancy poses a significant risk, particularly if these models are deployed in critical areas like autonomous driving.
Challenges when faced with unexpected situations
The researchers discovered a staggering drop in accuracy when LLMs encountered unexpected changes, such as detours or road closures. In certain scenarios, the systems completely failed to provide usable directions. “This highlights the urgent need to assess the robustness of AI when exposed to dynamic and unpredictable environments,” emphasized Ashesh Rambachan, an assistant professor in the MIT Laboratory for Information and Decision Systems.
The promise of coherent world models
The promise of harnessing LLMs for scientific advancements hinges on their ability to create coherent “world models” based on extensive data. Yet, despite their impressive language capabilities, if these models cannot accurately reflect real-world variables, their utility in areas such as AI-driven navigation becomes questionable. For instance, a theoretical application could include generating navigational maps from taxi trip data without manual input; however, if those maps are flawed, the AI's effectiveness diminishes drastically.
Evaluating limitations with deterministic finite automations
To better understand the limitations of these models, researchers employed deterministic finite automations (DFAs)—concepts illustrating sequences of states like those found in games or navigation scenarios. They evaluated LLMs using two primary metrics: “sequence determination” and “sequence compression.” The former assesses whether an LLM can recognize and understand variations in states, while the latter evaluates its capability to maintain coherence across identical states.
Random training can yield surprising results
Interestingly, the experiments revealed that LLMs trained on randomly generated sequences often formed more accurate world models than those trained through structured approaches. Keyon Vafa, the lead author of the study, noted that “observing random strategies in games, such as Othello, provides a broader insight into potential moves, including less optimal ones that professional players might avoid.” This signifies that exposure to diverse scenarios might enhance an LLM's aptitude for adapting to unforeseen circumstances.
Fragility exposed in dynamic conditions
Despite their ability to generate valid moves in Othello and correct driving directions, none of the LLMs examined developed a coherent world model for the game, nor did they produce an accurate map of New York City. When the researchers included disruptions like road closures into the mix, the performance of the navigation models plummeted dramatically. “Simply closing off 1 percent of the possible streets led to a drop in accuracy from nearly 100 percent to just 67 percent,” Vafa noted, highlighting the fragility of these systems.
Call for critical re-evaluation of LLMs
The study calls for a critical re-evaluation of how LLMs are architected and employed in real-world scenarios. With these findings in hand, researchers highlight the necessity for improved methodologies to ensure that AI systems can maintain reliability in dynamic and unpredictable situations. “While LLMs occasionally impress us with their outputs, it is essential to question whether they truly understand our world,” concluded Rambachan. “We must seek more rigorous answers, rather than depend solely on our instincts.
Conclusion: The urgency of understanding AI limitations
As AI continues to permeate everyday life, including driving applications, the urgency to understand the limitations of these technologies cannot be overstated. Are we ready to confront the reality that our cutting-edge AIs may not be as competent as we believe, especially when navigating the complexities of the real world?