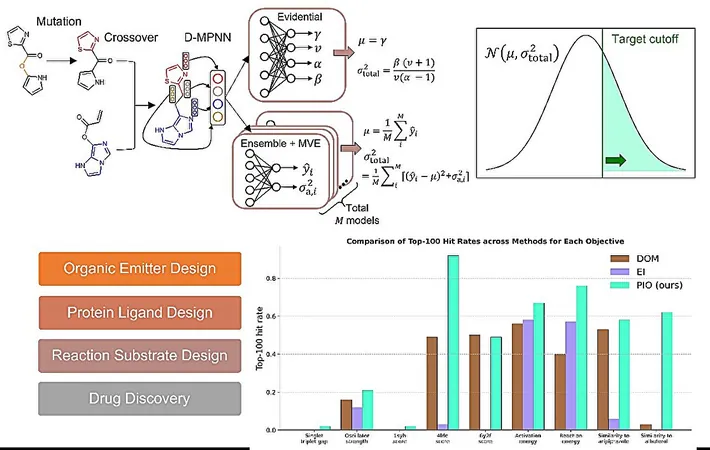
Revolutionizing Molecular Design: How AI is Harnessing Uncertainty for Breakthrough Discoveries
2025-04-16
Author: William
AI Meets Uncertainty: A Game-Changer in Molecular Design
In a groundbreaking advancement, researchers from National Taiwan University have unveiled a revolutionary method that enhances AI-assisted molecular design by incorporating uncertainty quantification (UQ) into graph neural network (GNN) models. This pivotal discovery not only boosts the efficiency of molecular optimization but also makes it significantly more robust.
Breaking Down Barriers: The Power of Uncertainty-Aware Models
Their research, recently highlighted in **Nature Communications**, elucidates how integrating UQ into computational-aided molecular design (CAMD) workflows can lead to smarter decision-making in both materials science and drug discovery. This innovative approach systematically explores whether UQ-enhanced directed message passing neural networks (D-MPNNs) can effectively navigate the vast and often unpredictable chemical landscapes that traditional machine learning models struggle with.
Navigating Complex Chemical Spaces: A New Hope
Real-world applications frequently encounter the challenge of dealing with molecules that exist beyond the confines of known training data, making reliable predictions difficult. To combat this, the research team combined D-MPNNs with genetic algorithms, paving the way for more flexible and library-free molecular optimization.
Tackling Real-World Challenges: Benchmarking Success
They rigorously assessed various uncertainty-aware optimization strategies across **16 diverse benchmark tasks** drawn from the Tartarus and GuacaMol platforms. These tasks encapsulate critical challenges in organic electronics, reaction engineering, and drug development, particularly multi-objective scenarios where multiple, often conflicting, molecular properties need to be weighed.
Probabilistic Improvement Optimization: The Star Performer
Among the strategies explored, probabilistic improvement optimization (PIO) consistently outperformed the rest. PIO effectively leverages UQ to predict the likelihood that a candidate molecule will meet specific design criteria, thus sidestepping unreliable extrapolations and honing in on chemically promising territories.
Expert Insight: A New Era for Chemical Design
Prof. Yi-Pei Li, the lead author of the study, shared, "Chemical design often demands a delicate balance among competing objectives within vast and uncertain search spaces. By integrating uncertainty quantification into machine learning-guided optimization, we present a methodological approach to tackle this complexity, significantly enhancing the reliability of AI-generated suggestions."
The Future of AI in Chemistry and Materials Science
This groundbreaking research lays a strong groundwork for uncertainty-aware molecular discovery, heralding an era of more dependable and data-efficient AI applications in the realms of chemistry and materials science, ensuring that the future of molecular design is not just intelligent but also reliable.