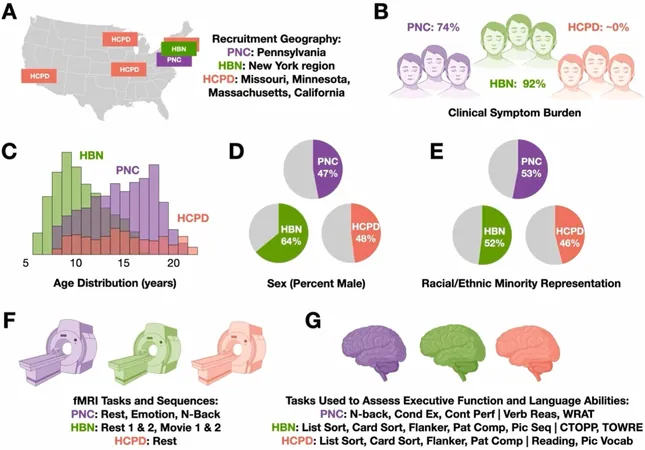
Revolutionizing Mental Health: How Diverse Datasets Are Paving the Way for Machine Learning in Neuroimaging
2024-11-04
Author: Olivia
Revolutionizing Mental Health: How Diverse Datasets Are Paving the Way for Machine Learning in Neuroimaging
In a groundbreaking study, researchers at Yale have made significant strides in connecting brain activity with behavior, an endeavor that holds tremendous potential for personalized treatment of mental health disorders and neurological conditions. The ability to relate neuroimaging data to behavioral patterns could transform how clinicians approach diagnoses and treatment plans.
Currently, scientists are employing brain imaging and behavioral data in training sophisticated machine learning models aimed at predicting symptoms or illnesses based on brain functionality. However, the true test of these models lies in their versatility; they must be applicable across a variety of populations and settings to be clinically useful.
Brendan Adkinson, the lead author of the recent study featured in Developmental Cognitive Neuroscience, points out that while predictive models tend to excel when evaluated on similar datasets, they often falter when tested against diverse data sources. "This limitation renders many models ineffective for real-world clinical situations," he explains.
The core of the issue arises from differences inherent in datasets, including factors such as age, sex, race, geography, and the presentation of clinical symptoms. Instead of viewing these variations as obstacles, researchers advocate for embracing them as essential elements of model development. Adkinson stresses, "For predictive models to hold clinical value, they must be capable of functioning effectively amidst these dataset-specific variances."
In an innovative approach to testing their models, the Yale team trained algorithms to predict two critical traits—language abilities and executive function—utilizing three markedly different large datasets. By training individual models on each dataset and then placing them against the other two, the researchers discovered promising outcomes. "Despite the noticeable dissimilarities between the datasets, our models maintained commendable performance, which, by neuroimaging standards, indicates that creating generalizable models is indeed possible," Adkinson states.
Looking to the future, Adkinson intends to delve deeper into how the principle of generalizability applies to specific populations. A major concern raised by the researchers is the predominance of data collection in metropolitan areas. This focus may inadvertently generate models that lack relevance for rural populations, thereby leaving a significant gap in clinical applicability.
Ultimately, as the field of neuroimaging progresses, harnessing diverse datasets can enhance our understanding of brain-behavior relationships and may even lead to groundbreaking advancements in tailored mental health treatments. The day may soon come when machine learning models not only predict symptoms with accuracy but also provide personalized therapeutic paths for individuals, regardless of their background or geographical location. Such advancements could redefine the landscape of mental health care.
Stay tuned, as we continue to monitor this fascinating field where technology meets brain science!