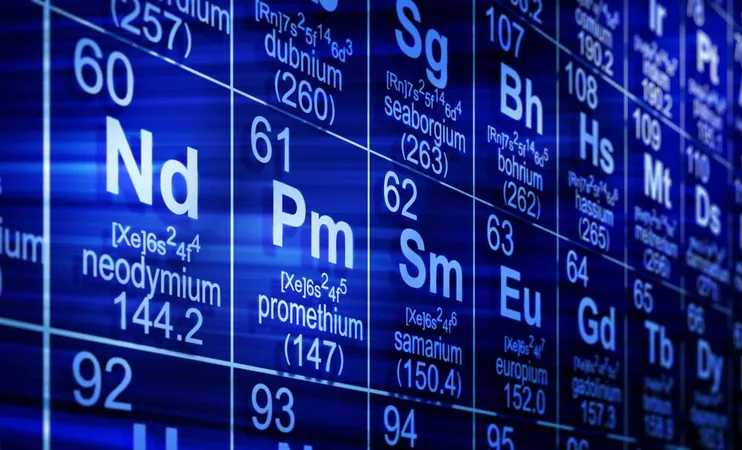
Revolutionizing AI: MIT's Groundbreaking Periodic Table for Algorithms
2025-05-01
Author: Michael
MIT Unveils a Game-Changer for AI Research
In a remarkable leap forward for artificial intelligence, researchers at MIT have introduced a pioneering framework that organizes AI algorithms similarly to how the periodic table classifies chemical elements. This innovative 'periodic table' categorizes algorithms based on their inherent properties, providing a new lens through which to view and utilize AI tools.
A Fresh Approach to Algorithm Classification
According to Popular Mechanics, the AI periodic table utilizes a novel model called Information Contrastive Learning, or I-Con. This mathematical foundation enables the classification of algorithms by evaluating their distinctive characteristics and performance metrics, primarily through a loss function.
I-Con assesses the variances between an algorithm’s predictions and the real outcomes, shedding light on its effectiveness. In a fascinating discovery, the MIT team identified that numerous algorithms, once thought to be radically different, actually share fundamental principles.
Understanding the AI Periodic Table
Just as the chemical periodic table groups elements based on atomic traits, the AI periodic table categorizes algorithms according to their mathematical properties. Algorithms with similar functionalities are clustered together, facilitating researchers' abilities to find the right tools for specific AI challenges.
This groundbreaking organization method aims to simplify the selection process for AI projects, enhancing collaboration and efficiency within the research community. The classification is chiefly based on how algorithms interact within the I-Con framework.
Serendipity Sparks Innovation
The creation of this AI periodic table stemmed from a lucky observation made by Alshamarri, an MIT graduate student. He noticed parallels between various machine learning methods, leading to an insightful exploration of their common mathematical structures.
As he shared, "The results highlighted in this research represent just a portion of the algorithms that might be unifiable through I-Con. We aspire for the community to adopt this perspective to foster collaboration and enhance algorithmic analysis across various machine learning techniques." This unanticipated insight could fundamentally alter how researchers approach the study and application of AI algorithms.
Blank Spaces: The Promise of Future Discoveries
Similar to the early iterations of the chemical periodic table, MIT’s AI version remains incomplete. There are still numerous gaps where the connections between certain algorithms and the I-Con framework are yet to be established. These open spaces indicate a wealth of opportunities for future exploration and innovation as new algorithms emerge or existing ones are reassessed through this framework.
The evolving nature of this periodic table not only suggests ongoing developments in AI research but also offers practitioners a valuable resource to stay abreast of advancements in the field. By organizing algorithms based on shared mathematical properties, MIT's groundbreaking periodic table is poised to revolutionize both research methodologies and practical AI applications.