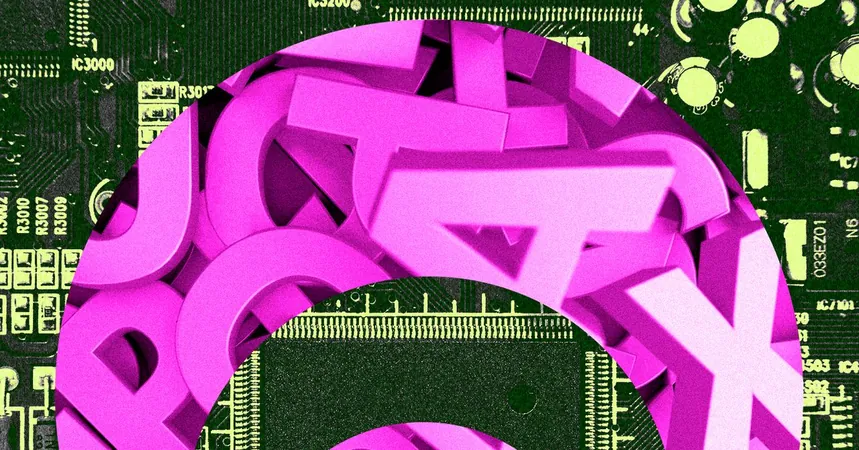
Revolutionizing AI: How Startups Are Ditching Data Centers for Global Collaboration
2025-04-30
Author: Charlotte
A New Era of AI Development
In a groundbreaking move, researchers have unveiled a novel large language model (LLM) that challenges traditional methods of artificial intelligence creation. This model, called Collective-1, harnesses the power of globally distributed GPUs, drawing from both private and public data to reshape AI’s future.
Behind Collective-1: Innovative Partnerships
Pioneered by startups Flower AI and Vana, Collective-1 signals a shift toward unconventional AI methodologies. Flower AI has developed techniques that enable training across hundreds of computers online, eliminating the need for centralized compute resources. Meanwhile, Vana has supplied data sources, including private messages from platforms like X and Telegram.
Small But Mighty: The Power of Collective-1
Though comparatively modest with only 7 billion parameters—miles behind the top contenders like ChatGPT and Gemini—Collective-1’s architecture sets the stage for expansive future growth. Nic Lane, co-founder of Flower AI, asserts that the distributed approach will allow for models much larger than Collective-1 as they aim to train models with 30 billion and later, 100 billion parameters.
Shifting the AI Landscape
The implications are significant: current AI development heavily favors well-resourced companies and countries that can afford massive data centers filled with cutting-edge technology. However, a decentralized model opens the door for smaller players, like startups and universities, to contribute to advanced AI research. This could also empower nations with less conventional tech infrastructure to pool their resources for formidable AI models.
Rethinking AI Training
The innovative distributed training method breaks down the computation typically centralized in data hubs, enabling operations over longer distances and even variable internet speeds. Google is also venturing into distributed learning with its new technique called DIstributed PAth COmposition (DiPaCo), promising more efficient calculations.
Photon: The Game-Changer
To facilitate the building of Collective-1, Lane and collaborators developed a tool named Photon that enhances the efficiency of distributed training. Compared to conventional training methods, Photon provides flexibility for incorporating new hardware, fostering an adaptable approach to building models.
Leveraging User Data Responsibly
Vana’s role in the development of Collective-1 goes beyond mere data provision. Their innovative software empowers users to share private data with AI builders while maintaining control over its usage. According to co-founder Anna Kazlauskas, this model allows users to monetize their contributions, representing a significant shift in how personal data is utilized in AI.
Unlocking New Paths in AI Training
This distributed approach not only democratizes AI but also opens up avenues for utilizing previously untapped data, especially in sensitive fields like healthcare. Mirco Musolesi, a computer scientist at University College London, highlights the potential of decentralized, privacy-sensitive data to enhance training methodologies without centralization risks.
Join the Conversation
What does the future hold for distributed machine learning, and would you consider sharing your data for a project like Collective-1? Let's discuss!