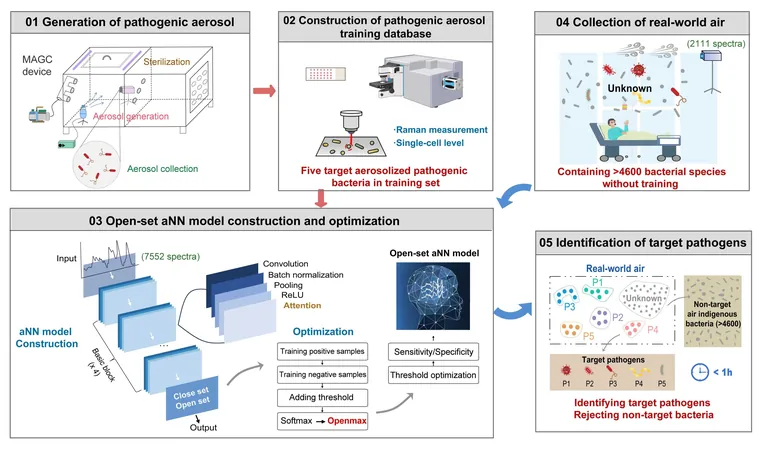
Revolutionary Single-Cell Technology Transforms Airborne Pathogen Detection in Real-World Environments
2025-01-13
Author: Sophie
Introduction
In an age where airborne pathogens pose significant health risks, especially during global pandemics, the urgent need for rapid and precise detection has never been more critical. Aerosols act as carriers, facilitating the spread of these pathogens from the environment to human hosts. Timely and accurate identification of airborne threats can significantly help in curbing infectious disease outbreaks and lowering the rates of hospital-acquired infections.
Limitations of Conventional Methods
Conventional methods for pathogen detection have been hampered by various limitations, including sluggish processing times, low sensitivity, and cumbersome procedures. However, a groundbreaking technique known as single-cell Raman spectroscopy has emerged as a game-changer in the field. This advanced technology characterizes the unique phenotypic features of microorganisms with impressive speed, operates without the need for culturing samples, and can simultaneously detect multiple targets.
Advancements in Technology
Recent developments in artificial intelligence have further enhanced the capabilities of Raman spectroscopy, refining its ability to differentiate subtle spectral differences between various bacteria. Despite these advancements, the challenge of creating comprehensive databases that cover the vast diversity of environmental microorganisms, many of which remain unculturable, has persisted. Identifying pathogens in the midst of complex airborne microbial communities is particularly daunting due to the intricate nature of these environments.
Innovative Approach by Researchers
A team of visionary researchers, led by Prof. Zhu Yongguan and Prof. Cui Li from the Institute of Urban Environment at the Chinese Academy of Sciences, has recently unveiled an innovative airborne pathogen detection technology that tackles these complexities head-on. Their system, reported in *Science Advances*, synergizes single-cell Raman spectroscopy with a cutting-edge open-set deep learning algorithm.
Raman Spectral Database
To facilitate this technology, the researchers constructed a Raman spectral database specifically for pathogens present in aerosol samples. They integrated an open-set loss function and established optimization thresholds into a powerful deep learning model. This approach allows for the identification of five critical airborne pathogens amid the noise of complex environmental data.
Efficiency and Accuracy
Impressively, for air samples that contain more than 1% pathogens, the entire process—from sample collection to result reporting—takes merely one hour. The system boasts an astonishing average identification accuracy of 93% for the targeted pathogens, while also achieving a remarkable 36% reduction in false positive rates compared to traditional closed-set algorithms. With the ability to detect pathogens down to the single-cell level, this method shows exceptional resilience against interference, outperforming classical detection techniques.
Real-World Applications
Moreover, this technology has been rigorously tested across multiple real-world environments, including hospitals, shopping malls, dining areas, waste processing facilities, microbiology labs, and public restrooms. Each validation underscores its efficacy in overcoming the sluggishness and inadequacies of traditional pathogen detection methods.
Conclusion
As this innovative technology paves the way for efficient environmental biosafety monitoring and early alerts, it is set to play a crucial role in the ongoing battle against airborne pathogen transmission. Could this be the breakthrough that revolutionizes public health safety? Only time will tell as this pioneering method is implemented on a broader scale.