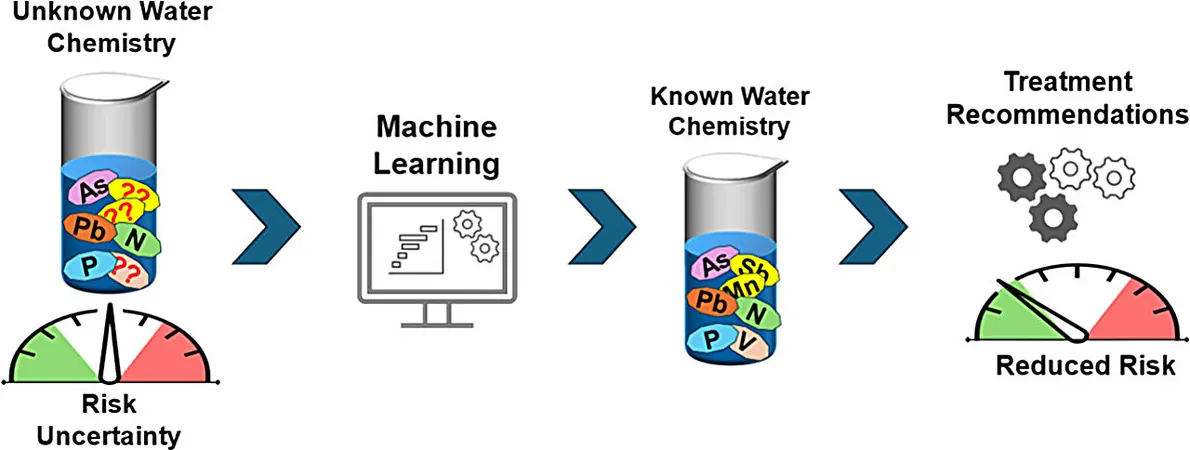
Revolutionary Machine Learning Tool Unveils Hidden Groundwater Contamination Risks!
2024-11-12
Author: William
Introduction and Overview
An innovative team of researchers has harnessed the power of machine learning to develop a groundbreaking framework capable of predicting inorganic pollutants in groundwater, even with limited data. This advancement is set to significantly enhance water quality monitoring, enabling regulators and public health officials to prioritize aquifers that require urgent testing.
Research Highlights
The proof-of-concept research, focusing on regions such as Arizona and North Carolina, holds promise for application across various areas lacking comprehensive groundwater quality data. The study, titled "Multiple Data Imputation Methods Advance Risk Analysis and Treatability of Co-occurring Inorganic Chemicals in Groundwater," is now available for public access in the reputable journal Environmental Science & Technology.
Importance of Groundwater Quality
Groundwater serves as a vital drinking water source for millions but often harbors harmful pollutants that pose serious health risks. Unfortunately, many regions face challenges in maintaining complete datasets for groundwater quality. “Water quality monitoring is labor-intensive and costly, especially when testing for multiple pollutants,” states Yaroslava Yingling, a distinguished professor at North Carolina State University and co-author of the research. “This highlights the need for a strategy that directs our limited monitoring resources effectively.”
Research Findings
The research identifies natural toxins such as arsenic and lead, which frequently appear together due to geological conditions. This raised an intriguing inquiry: can the presence and concentrations of other pollutants be predicted from existing water quality data?
Co-first author Alexey Gulyuk elaborates, “In addition to examining elements that threaten human health, we also explored predicting beneficial elements like phosphorus, which can have adverse effects if not managed properly in certain environments.”
Data Analysis and Model Training
To tackle this challenge, the researchers analyzed an extensive dataset, incorporating over 140 years worth of groundwater quality monitoring from North Carolina and Arizona, which included more than 20 million data points across 50 different parameters. This immense compilation trained their machine learning model to accurately forecast which pollutants might exist, even when faced with minimal information.
Significance of Findings
A key revelation from the study indicates that the machine learning model predicts a higher number of groundwater sources exceeding safe drinking water standards than previously believed. While field data suggested that 75% to 80% of sampled sites were safe, the model casts doubt on this, proposing that only 15% to 55% may indeed be free of risks.
“We’ve pinpointed numerous groundwater locations that warrant immediate further investigation,” says Minhazul Islam, another co-first author and Ph.D. student at Arizona State University. “By identifying potential contamination hot spots, state officials can deploy resources strategically, enhancing targeted sampling and effective treatment solutions.”
Future Prospects
The researchers are optimistic but acknowledge that the real test lies ahead—validating these predictions in real-world scenarios. “This approach shows exceptional promise, yet we must see how well it performs in practical applications,” remarks Gulyuk.
Looking ahead, the team aims to refine the model by diversifying its training data across different U.S. regions, integrating new environmental data sources to monitor emerging contaminants, and executing real-world tests to strengthen groundwater safety measures internationally.
Conclusion
According to Paul Westerhoff, co-author and Regents' Professor at Arizona State University, “We’re laying a foundation for proactive global water safety measures with this model.”
In addition, Jacob Jones, director of the Science and Technologies for Phosphorus Sustainability (STEPS) Center at NC State, suggests that extending this model's capabilities to manage phosphorus levels in groundwater could revolutionize how potential contamination risks are identified and managed. “Such an advancement would promote sustainable practices across different ecosystems and agricultural methodologies.”
Stay tuned as this pioneering research holds the key to safeguarding our precious groundwater resources and enhancing public health safety in the face of emerging environmental challenges!