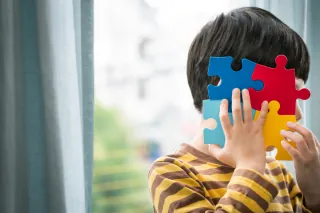
Revolutionary AI Study Challenges Conventional Autism Diagnosis Standards!
2025-03-26
Author: Emma
Introduction
In a groundbreaking new study, researchers at The Neuro (Montreal Neurological Institute-Hospital) of McGill University and Mila Quebec AI Institute have used advanced large language models (LLMs) to scrutinize digital health records, uncovering surprising insights that may redefine the criteria for autism diagnosis.
Traditional Diagnosis Standards
The traditional framework for diagnosing autism heavily relies on the DSM-5 criteria, which classifies symptoms into two primary categories: behaviors and social communication. However, this new analysis suggests that social interaction markers—commonly seen as pivotal indicators of autism, such as emotional reciprocity and non-verbal cues—may not be as reliable as once thought. In fact, these traits were found to be present in individuals with and without autism, leading researchers to question their diagnostic specificity.
Key Findings of the Study
By meticulously examining over 4,200 clinical reports from children in Quebec, the study highlights that while socialization aspects lacked clear differentiation in autism diagnoses, traits associated with repetitive behaviors, intense interests, and atypical sensory responses exhibited a substantial correlation with autism. These findings challenge the entrenched beliefs within the medical community and propose a significant shift in focus towards these behaviors when evaluating individuals for autism spectrum disorders.
Call for Re-evaluation
Published in the prestigious journal Cell, the study advocates for a re-evaluation of the existing diagnostic criteria, urging healthcare professionals to place a stronger emphasis on repetitive actions and special interests as indicators of autism.
The Role of AI in Diagnosis
But how exactly could artificial intelligence enhance this process? Currently, autism diagnoses are primarily based on extensive clinical evaluations, with no biological tests available to streamline assessments. This often results in delays in receiving critical services and support. By leveraging AI and focusing on the most predictive characteristics of autism, the research team believes it is possible to substantially speed up the diagnostic process while simultaneously improving its accuracy.
Future Predictions
Danilo Bzdok, the senior author of the study and a scientist at The Neuro and Mila, predicts that "in the future, large language model technologies may prove instrumental in reconsidering what we call autism today." This approach is seen as a complement to traditional methods that heavily rely on expert judgment.
Potential Impact
The implications of this study can be monumental, not only for current diagnostic practices but also for the broader understanding of autism itself. In a world where personalized medicine and tailored treatment are becoming the norm, this rethinking of autism criteria could lead to better outcomes for individuals on the spectrum.
Support and Collaboration
This pioneering study was made possible through the support of several esteemed organizations, including the Brain Canada Foundation and Health Canada, highlighting the importance of collaborative efforts in advancing medical research.
Conclusion
Stay tuned as the landscape of autism diagnosis continues to evolve!