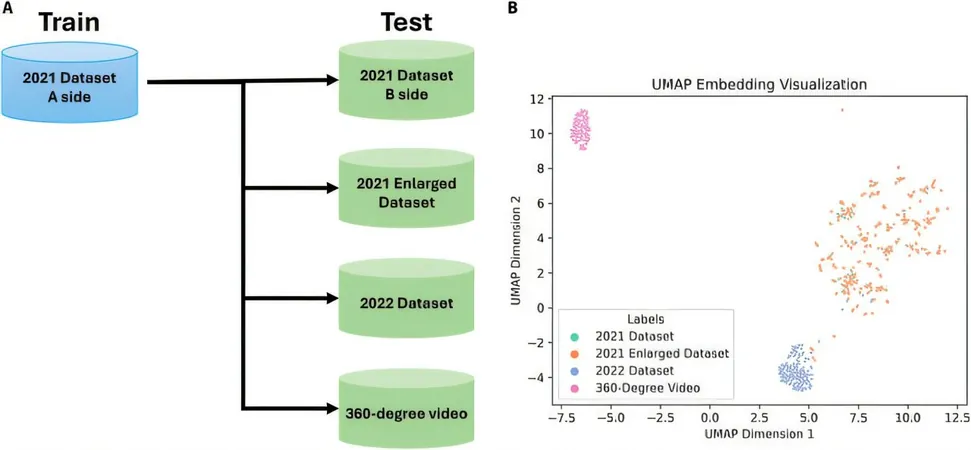
Revolutionary AI-Driven Technique Transforming Soybean Breeding!
2024-11-13
Author: Sophie
Introduction
As the global population continues to surge and the demand for protein skyrockets, modern agriculture faces unprecedented challenges. Soybeans, a crucial source of protein, are at the forefront of this battle. It is essential for plant breeders to enhance soybean plant architecture and improve per-plant yields to meet the needs of contemporary farming systems.
Challenges in Traditional Breeding Methods
Traditionally, soybean breeding has relied on time-consuming methods such as visual assessments and manual harvesting of individual plants. These techniques often fall short when it comes to evaluating important plant traits that depend on complex interactions between physiology, structure, and environmental variables. Current breeding tools lack the precision needed to effectively measure these traits, leading to inefficiencies in the breeding process.
Pioneering AI Advancements
To tackle these challenges, Wei Guo, an associate professor at the University of Tokyo (UTokyo), is pioneering advancements in artificial intelligence (AI) to revolutionize the field of plant phenomics. Guo has partnered with Dr. Akito Kaga, a soybean researcher at NARO, to develop a groundbreaking image capture and AI analysis pipeline that significantly improves the accuracy and speed of assessing single plant yield, architecture, and seed localization. Their groundbreaking findings appear in the journal *Plant Phenomics*.
Affordability and Accessibility
"What sets our work apart is the affordability of our method," explains Guo. "While many AI applications in agriculture require expensive aerial or robotic platforms, our system is accessible to soybean breeders with limited budgets."
Development of MSAnet
Ph.D. candidate Tang Li has played a pivotal role in creating a novel AI-based image analysis tool called the Multi Scale Attention Network (MSAnet). This deep learning pipeline is designed to automatically detect and estimate the number and spatial arrangement of soybean seeds on a plant in various field conditions.
Overcoming Hurdles in Development
Li notes, “The biggest hurdle in developing MSANet was ensuring we accurately focus only on the foreground while using minimal computational resources.” The process involves generating seed distribution heatmaps from upsampled images, which are then matched with neighboring images through a downsampling technique. A kernel density algorithm refines the seed counting process, achieving results unparalleled by previous systems.
Empowering Breeders
The outcome? Breeders now have access to intuitive graphs illustrating vertical seed distribution on individual plants. This information can be crucial for evaluating a wide range of previously hidden traits in potential new varieties or conducting genetic analyses on these traits.
Precision in Breeding Process
As the need for high-efficiency production increases, this innovative technique allows soybean breeders to accurately select superior crop varieties tailored for specific farming practices. Dr. Kaga emphasizes the importance of the MSANet: "By enabling accurate quantification of the lowest seed positions—an essential trait for modern machine harvesting—our method brings a new level of precision to the breeding process."
Future Implications
With these advancements, breeders can quickly identify promising new varieties that embody the desired combination of traits. "I'm thrilled that our concept of vertical seed distribution has been acknowledged by breeding expert Dr. Kaga, and I'm eagerly anticipating its real-world application,” adds Li.
Conclusion
The future of soybean breeding is bright, fueled by AI innovation, which promises to enhance food security and sustainability for a growing global population! Could this revolutionary technique be the key to feeding the world? Stay tuned for more updates!