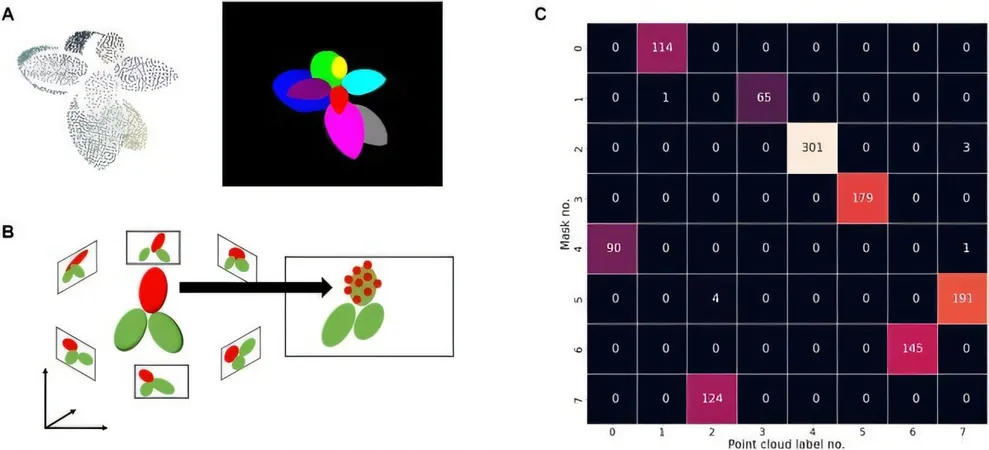
Revolutionary 3D Leaf Edge Reconstruction Method Set to Transform Plant Morphology Analysis!
2024-11-11
Author: Sophie
Leaves play a vital role in photosynthesis and numerous physiological functions, showcasing an incredible array of shapes tailored to help plants thrive in diverse environments. However, traditional methods of measuring leaf morphology have predominantly relied on 2D imaging, which often overlooks the intricate 3D structures that are essential for a thorough understanding of leaf functionality.
Recognizing the limitations of conventional techniques, scientists have been spurred to innovate more advanced 3D imaging methods. Nevertheless, accurately mapping leaf edges—particularly in complex structures—remains a formidable challenge.
Recent research published in Plant Phenomics has introduced a groundbreaking approach for nondestructively analyzing leaf morphology, enabling a precise 3D mapping of plant structures. This innovative method combines state-of-the-art deep-learning-based 2D instance segmentation with curve-based 3D reconstruction techniques, setting a new standard in the field.
The researchers initially tested their method on virtual leaf models under optimal conditions, successfully reconstructing 3D leaf edges by deriving 2D edges from true masked imagery. A crucial factor in this process was the support threshold (τt); while lower values led to reconstruction inaccuracies, higher values resulted in coverage issues.
In cases involving multiple leaves, effectively identifying leaf correspondences was pivotal for achieving accurate reconstructions, particularly in the face of occlusions. The results indicated that reconstructing larger leaves was generally more manageable, whereas smaller leaves with significant curvature posed notable challenges.
Notably, the method showed sensitivity to camera noise; however, increasing the number of images taken did not necessarily enhance precision, indicating a need for a refined imaging approach.
Using Mask R-CNN on real plant data, the team was able to generate individual leaf masks, although smaller leaves proved difficult to reconstruct, as reflected in lower average precision (AP) scores. When applied to actual soybean plants at various growth stages, the method successfully reconstructed most leaves at optimal support thresholds but encountered hurdles with heavily occluded or smaller leaves, sometimes leading to artifacts and shape distortions.
Testing across a range of leaf types revealed that while lobed edges were captured effectively, serrated and elongated leaves displayed reduced detail, particularly at the tips. The method performed well with leaves exhibiting up to three holes, but accuracy diminished with increased hole numbers.
In summary, this pioneering approach successfully captures intricate leaf morphologies but requires further refinement to tackle exceptionally complex or obstructed structures.
Dr. Koji Noshita, one of the study's key researchers, commented, “Our method represents a significant breakthrough in 3D leaf edge reconstruction. By merging deep learning with curve-based techniques, we can achieve unparalleled detail in leaf morphology analysis, carrying critical implications for agricultural research.”
This state-of-the-art 3D leaf edge reconstruction technique signifies a monumental advancement in plant phenotyping. It opens up a world of possibilities for in-depth studies of plant morphology, with vast applications in both agricultural and ecological research. The team believes that ongoing enhancements of this method will lead to more efficient and productive agricultural practices, ultimately addressing the pressing global food security challenges we face today.
Don’t miss out on the future of agriculture—unlock the secrets of plant growth with this ground-breaking technology!