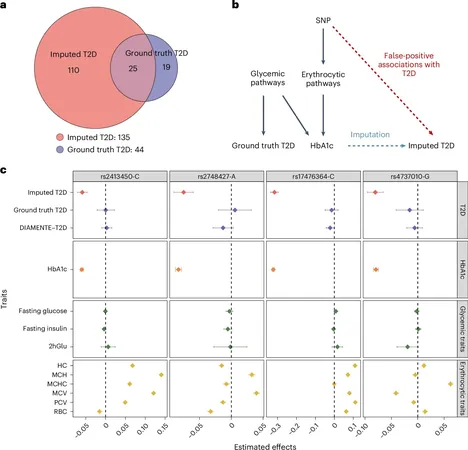
Researchers Sound Alarm on AI in Genomic Studies: Potential Dangers Exposed!
2024-11-04
Author: Jacob
Researchers Sound Alarm on AI in Genomic Studies: Potential Dangers Exposed!
Researchers from the University of Wisconsin–Madison are raising serious concerns about the growing reliance on artificial intelligence (AI) tools in genomic studies, warning that they can lead to erroneous conclusions regarding the relationships between genes and various physical characteristics, including susceptibility to diseases like diabetes.
As the usage of AI in genome-wide association studies (GWAS) proliferates, scientists are scanning an overwhelming array of genetic variations among large populations in search of correlations between genetics and health traits. However, they caution that the simplistic narratives derived from AI can often misrepresent the complexities underlying genetic influence.
The Twist in Genetics and Disease Connection
While some genetic mutations are clearly associated with specific conditions—like cystic fibrosis—most genetic relationships are not linear. GWAS attempts to clarify these intricate relationships by utilizing large genetic and health data repositories, such as the National Institutes of Health's All of Us project and the UK Biobank. Yet, sometimes these databases lack comprehensive health data on the very diseases researchers aim to understand.
Qiongshi Lu, an associate professor of biostatistics and medical informatics at UW–Madison, emphasizes, “Given the cost and effort involved in measuring certain health conditions, researchers may not have sufficient sample sizes to draw valid statistical conclusions about their genetic associations.”
AI's Role: A Double-Edged Sword
Faced with incomplete datasets, researchers are increasingly turning to advanced AI and machine learning models to predict disease risk and complex traits. However, Lu and his colleagues have highlighted the potential dangers of over-reliance on these technologies. Their recent study, published in *Nature Genetics*, demonstrates how a prevalent machine learning algorithm used in GWAS can incorrectly link various genetic variations to an individual's likelihood of developing type 2 diabetes.
“The issue arises when researchers accept the AI-generated diabetes risk as unequivocal truth,” Lu warns, “leading to the misinterpretation that these genetic variations directly correlate with diabetes, which is not necessarily the case.” This phenomenon of “false positives” is an alarming trend that can significantly skew our understanding of genetic contributions to diseases.
Introducing a Solution: A New Statistical Approach
To combat the inherent biases introduced by incomplete data and reliance on AI, the research team proposes a new statistical method aimed at enhancing the validity of AI-assisted genome-wide association studies. By applying this method, researchers can filter out biases from machine learning algorithms and achieve more accurate insights. “This new strategy is statistically optimal,” Lu notes, emphasizing its effectiveness in pinpointing genetic associations, including those related to bone mineral density.
Proxy Data: A Different Route to Disillusionment
In their ongoing research, Lu and his team reveal that the issues plaguing genetic studies extend beyond AI. They also critique the practice of relying on proxy data to bridge informational voids. For example, many databases, such as the UK Biobank, might offer exhaustive genetic data but often lack comprehensive information about late-onset diseases like Alzheimer's. Some researchers have tried to circumvent this by using family health histories as proxy data, which their studies suggest can create misleading links between genetic predispositions towards Alzheimer’s and enhanced cognitive performance.
“While larger datasets provide greater statistical power, they can also magnify biases and increase the chances of errors,” warns Lu, underscoring the need for rigorous statistical practices in handling genomic data.
As research in genomics continues to evolve, the University of Wisconsin–Madison team's findings serve as a powerful reminder: while AI has the potential to revolutionize our understanding of genetics, it must be applied with caution and critical oversight to avoid detrimental misinterpretations that could impact public health.
Stay tuned! The genomic revolution may hold the answers to many health mysteries—but how we navigate the challenges could hold the key!