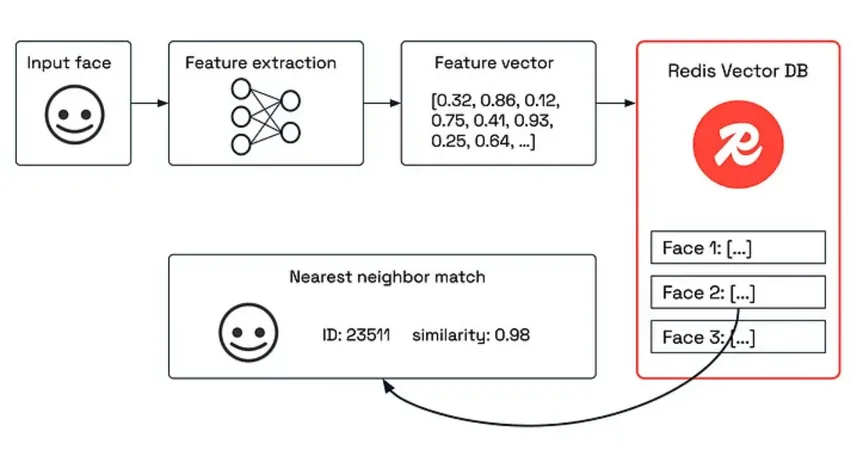
Redis 8 Revolutionizes AI with Cutting-Edge Vector Sets!
2025-04-20
Author: Jacques
Redis Unleashes Vector Sets for AI Applications!
In a groundbreaking development, Redis has officially introduced Vector Sets, a game-changing data type specifically engineered for vector similarity and tailored for the rapidly evolving landscape of AI applications. This innovative addition marks the highly anticipated return of Salvatore Sanfilippo, fondly known as ‘antirez,’ the original creator of Redis, who rejoined the company to elevate its offerings.
What Are Vector Sets?
Vector Sets can be thought of as a twist on Redis's traditional Sorted Sets, but instead of assigning a score to string elements, they connect those elements to a specific vector. This sleek functionality allows users to effortlessly add items and subsequently retrieve those that bear the closest resemblance to a given vector. Plus, they come with advanced filtered search capabilities, enabling the use of vector similarity alongside scalar filters, creating a powerhouse of analytical possibilities.
Sanfilippo's Vision Realized!
Sanfilippo elucidates the essence of this new structure on his blog: 'The goal here is to forge a new data type resembling a Set that replaces the scalar score with a vector, enabling users to add and extract elements seamlessly while focusing solely on Redis’s core data structure properties.' This structure empowers users to efficiently query elements similar to specified vectors, revolutionizing data interaction.
Backed by Robust Technology!
At the heart of Vector Sets lies the advanced HNSW data structure, backed by a custom implementation to enhance speed and functionality. Rowan Trollope, CEO of Redis, heralds Sanfilippo’s return, stating that his expertise has birthed an API that’s not only user-friendly but also resonates with Redis’s commitment to high-performance, low-complexity solutions.
Unlocking New AI Use Cases!
Vector databases are becoming essential for Generative AI (GenAI) applications, enabling them to fetch semantically relevant information that enhances the context of Large Language Models (LLMs). From powering intelligent chatbots and recommendation systems to facilitating real-time face recognition, the use cases are boundless. Mirko Ortensi, a product manager at Redis, elaborates on how Vector Sets can streamline face recognition by storing known faces as vectors and executing efficient searches for matching identities.
A Vision for Performance!
Sanfilippo stresses the meticulous approach taken to implement vector similarity by reengineering HNSWs from the ground up. His commitment to not just adopting existing code but creating a tailored solution underscores the innovative spirit of Redis. This latest version also integrates multithreading for every vector similarity request and introduces 8-bit and binary quantization capabilities, setting the stage for astonishing processing speeds.
More than Just Vector Sets!
But the excitement doesn’t stop there! Redis has rolled out LangCache, a semantic caching service designed specifically for AI applications. LangCache aims to significantly reduce the costly and time-consuming calls to LLMs by efficiently caching their responses. This promises to not only enhance performance but also optimize costs.
With these advancements, Redis is not just keeping up with the demand for AI capabilities—it’s leading the charge towards a smarter, faster, and more efficient future!