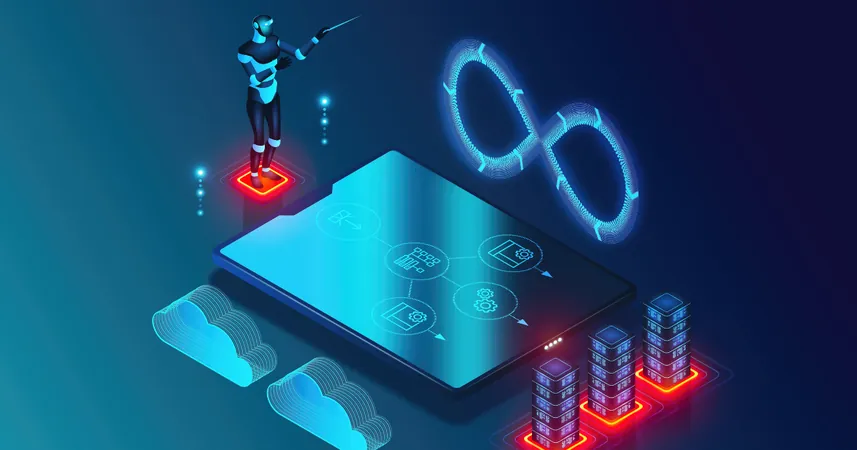
Pinterest Revolutionizes Goku Time-Series Database for Unmatched Efficiency
2024-11-06
Author: Liam
Pinterest Revolutionizes Goku Time-Series Database for Unmatched Efficiency
Pinterest has taken significant strides to modernize and enhance its Goku time-series database, implementing key updates that focus on optimizing both storage and resource utilization while maintaining top-tier service quality.
Initially developed to address specific limitations in OpenTSDB, Pinterest's in-house database engine, Goku, has undergone transformative improvements. The recent enhancements were detailed in a blog post from the Goku team, which introduced exciting new features like a metrics namespace and a system for identifying top write-heavy metrics. These developments have led to a remarkable 37% reduction in stored time series data by optimizing how data is managed and stored.
The introduction of a metrics namespace organizes metric configurations in a streamlined way, facilitating efficient data management. These configurations are dynamically stored in a shared config file, which all hosts in the Goku ecosystem monitor. Any updates trigger an immediate alert to the Goku processes, enabling them to adapt in real-time to changes.
Pinterest has also made architectural modifications that significantly chip away at infrastructure costs. Notably, advancements in indexing for metric names have slashed memory consumption from 12 GB to just 3 GB per host. Meanwhile, implementing dictionary encoding within the Goku Compactor has resolved critical out-of-memory challenges that previously hindered performance, thus allowing the company to leverage less expensive hardware solutions.
Memory optimization has been another focal point, as Pinterest’s engineering team meticulously tackled internal fragmentation and over-allocated memory. These efforts have yielded substantial reductions in memory use, exemplified by updates that brought memory consumption down by an impressive 8-11 GB per host.
Efficient storage and processing of time-stamped data have been further enhanced by advanced time-series compression algorithms. These algorithms are essential in minimizing data sizes by uncovering patterns and redundancies, which not only expedites query processing but also significantly trims storage expenditures. Techniques employed include delta encoding, delta-of-delta encoding, and XOR-based compression. For instance, TimescaleDB, a leading open-source time-series database, achieves over 90% storage efficiency, leading to remarkable cost savings.
Companies such as Meta have adopted similar strategies with their Gorilla time-series database, which utilizes compression methods to drastically reduce storage footprints and boost query performance.
Pinterest's advancements are part of a larger trend in the tech industry aimed at optimizing time-series data management systems. This trend includes notable initiatives like Apple's FiloDB, Netflix’s Atlas, Uber’s M3, Meta’s Gorilla, and Salesforce's Argus. Many of these projects, including Goku, are making their way to open-source platforms like GitHub, showcasing a collective move towards more scalable and economically sound data infrastructures.
As a direct result of these enhancements, Pinterest has celebrated a remarkable 40% reduction in time-series storage and a staggering 70% decrease in operational costs. These gains have further positioned the company to handle a 30% increase in organic storage growth without necessitating additional resource allocation.
In a landscape where observability costs are rising—often being the second-largest expense after infrastructure—Pinterest's journey serves as a beacon for other enterprises. The insights offered by industry professionals on platforms like Reddit continue to highlight the growing importance of efficient data management. As one user aptly noted, observability expenses are becoming a significant financial consideration for many organizations.
This blend of innovation and strategic planning is not just transforming Pinterest but is likely to inspire a slew of advancements across the tech industry, paving the way for smarter data infrastructures in the near future.