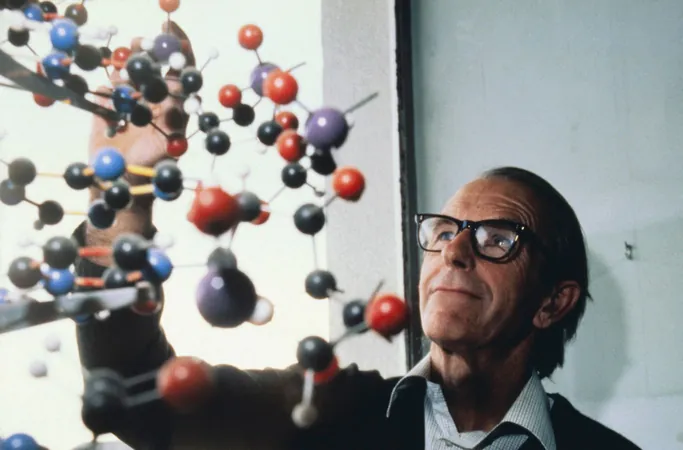
Microsoft Unveils Revolutionary AI Approach to Transform Materials Science
2025-01-21
Author: William
Introduction
In the age of generative AI, many are familiar with popular tools like Dall-E that can create stunning images. However, the true potential of generative AI extends far beyond simple image generation, especially when it comes to more complex fields like materials science. In a groundbreaking initiative, a team at Microsoft is harnessing the power of AI through a diffusion model aimed at discovering innovative materials for advanced applications.
MatterGen: A New Era in Material Discovery
Microsoft has introduced a program called MatterGen, which uses AI to streamline the often painstaking and resource-intensive process of material discovery. Traditionally, identifying a new material suitable for a specific application has been akin to 'finding a needle in a haystack.' Researchers have struggled through time-consuming experimental methods, or more recently, computational screenings of extensive materials databases. Despite advancements, the search for materials with desirable properties can still require sifting through millions of potential candidates.
The Diffusion Model Advantage
By applying a diffusion model, the Microsoft team has added considerable efficiency to this process. When the AI was trained on over 608,000 stable materials, it generated promising candidates for novel materials that could fit a wide range of high-tech applications, such as in electronics, energy storage, and construction. This process not only accelerates discovery but also reduces the costs associated with traditional trial-and-error methods.
How MatterGen Works
At the heart of MatterGen's technology is the diffusion process. Essentially, the AI begins with a defined object, such as an image or a molecular structure, then applies a 'noising' technique that transforms it into an abstract version. The model then 'denoises' the object, producing a new material with enhanced qualities tailored to researchers' specifications. This innovative approach signifies a significant leap in how materials can be developed.
Challenges Ahead
However, the journey is not without its challenges. One significant hurdle is the issue of compositional disorder; this refers to the random arrangement of atoms within synthesized materials, which can radically affect their properties. Microsoft researchers highlight that conventional algorithms often struggle to distinguish between materials that differ only in the arrangement of similar elements.
Real-World Implications of Microsoft’s AI
To illustrate this, consider metal alloys like brass, composed of copper and zinc. In a perfectly structured alloy, the atoms would align in a consistent fashion. However, due to compositional disorder, these atoms can swap positions, leading to variations that ultimately influence attributes like strength and electrical conductivity.
Impact on Industry
Microsoft's exploration into materials science isn't just theoretical; it has major implications for industries that rely on cutting-edge technology. For instance, the team emphasized the pressing need for innovation in lithium-ion battery technology. Since its inception in the 1980s with lithium cobalt oxide, this battery type has powered everything from smartphones to electric vehicles. However, given the scarcity and environmental impact of lithium mining, researchers are on a quest for alternatives that require less lithium.
Sustainable Battery Innovations
Recent advancements show promising results, with teams, including those at the Pacific Northwest National Laboratory, successfully developing battery designs that use 70% less lithium. This could mark a pivotal shift in energy technology, allowing for more sustainable practices while meeting the growing demand for efficient power sources.
Looking to the Future
As Microsoft continues to refine its generative AI techniques, the implications for materials science are profound. Imagine a future where not only are supply chains optimized and production costs lower, but the resulting materials are also safer and of higher quality. The integration of AI in this space signals a transformative era in materials engineering—a future where the possibilities are limited only by our imagination.
Conclusion
Stay tuned, as innovations like MatterGen hold the key to unlocking a new world of advanced materials!